Llorma : Local Low-Rank Matrix Approximation
International Conference on Machine Learning(2016)
摘要
Matrix approximation is a common tool in recommendation systems, text mining, and computer vision. A prevalent assumption in constructing matrix approximations is that the partially observed matrix is low-rank. In this paper, we propose, analyze, and experiment with two procedures, one parallel and the other global, for constructing local matrix approximations. The two approaches approximate the observed matrix as a weighted sum of low-rank matrices. These matrices are limited to a local region of the observed matrix. We analyze the accuracy of the proposed local low-rank modeling. Our experiments show improvements in prediction accuracy over classical approaches for recommendation tasks.
更多查看译文
关键词
Matrix approximation,non-parametric methods,kernel smoothing,collaborative Filtering,recommender systems
AI 理解论文
溯源树
样例
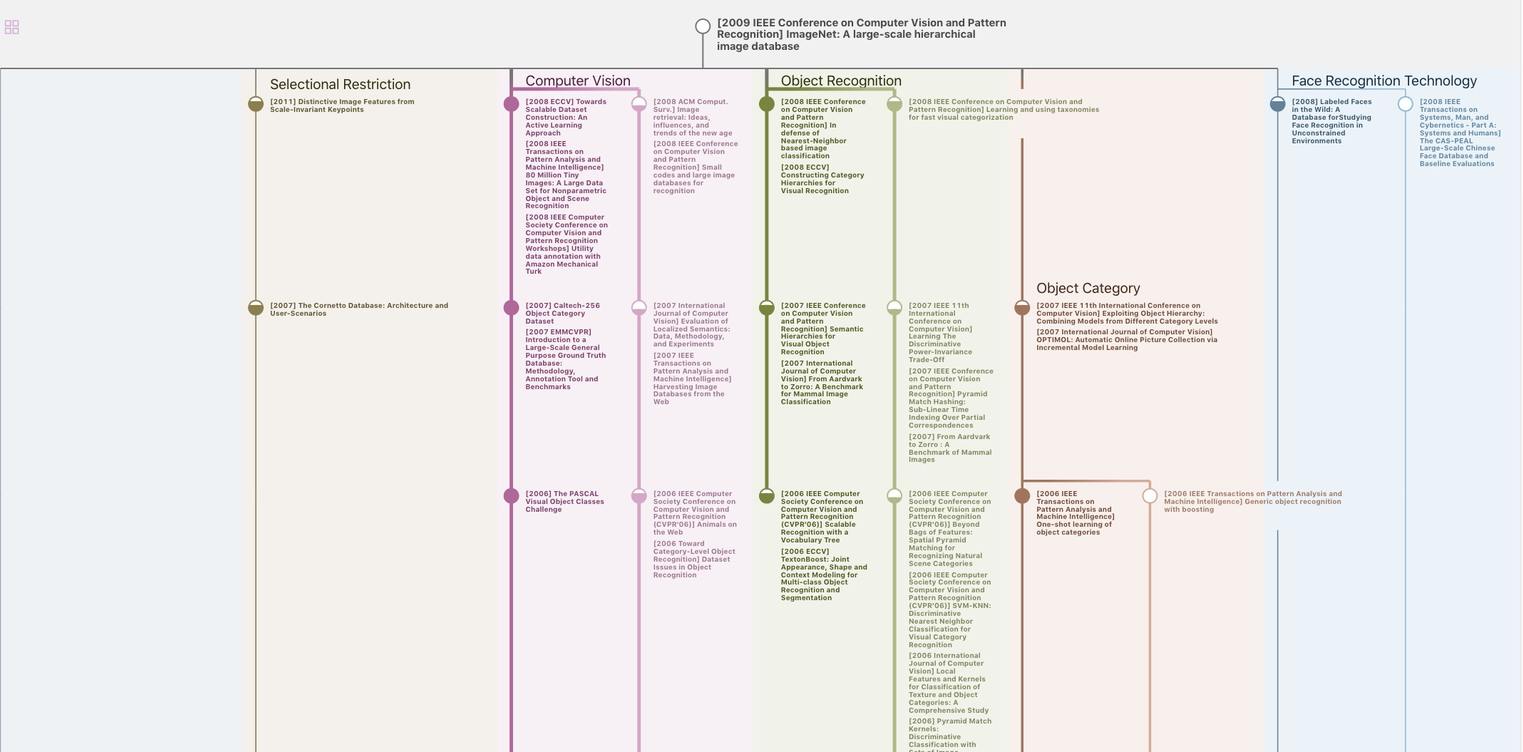
生成溯源树,研究论文发展脉络
Chat Paper
正在生成论文摘要