Lazy Paired Hyper-Parameter Tuning.
IJCAI '13: Proceedings of the Twenty-Third international joint conference on Artificial Intelligence(2013)
摘要
In virtually all machine learning applications, hyper-parameter tuning is required to maximize predictive accuracy. Such tuning is computationally expensive, and the cost is further exacerbated by the need for multiple evaluations (via cross-validation or bootstrap) at each configuration setting to guarantee statistically significant results. This paper presents a simple, general technique for improving the efficiency of hyper-parameter tuning by minimizing the number of resampled evaluations at each configuration. We exploit the fact that train-test samples can easily be matched across candidate hyper-parameter configurations. This permits the use of paired hypothesis tests and power analysis that allow for statistically sound early elimination of suboptimal candidates to minimize the number of evaluations. Results on synthetic and real-world datasets demonstrate that our method improves over competitors for discrete parameter settings, and enhances state-of-the-art techniques for continuous parameter settings.
更多查看译文
关键词
hyper-parameter tuning,candidate hyper-parameter configuration,continuous parameter setting,discrete parameter setting,early elimination,general technique,hypothesis test,multiple evaluation,power analysis,predictive accuracy
AI 理解论文
溯源树
样例
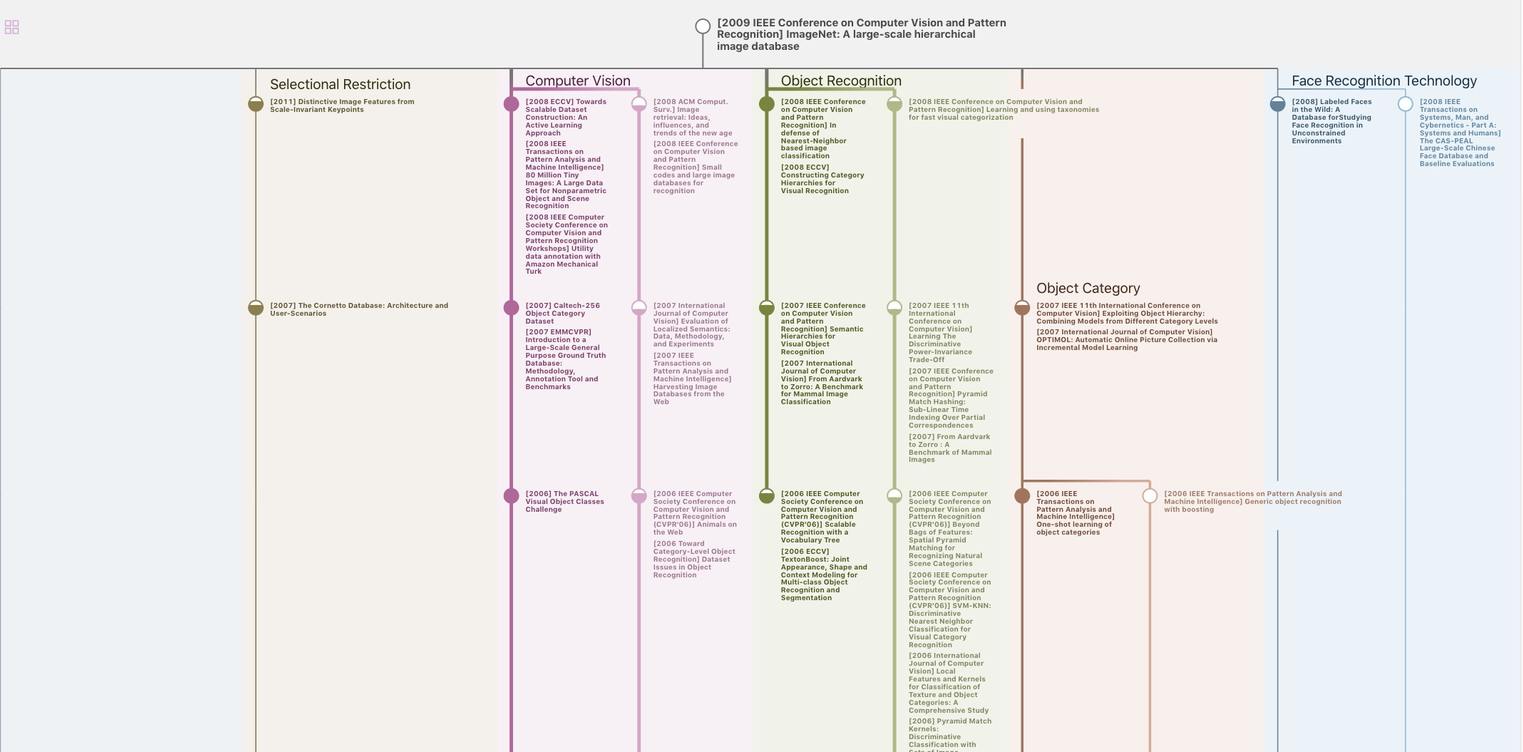
生成溯源树,研究论文发展脉络
Chat Paper
正在生成论文摘要