Generalized Information-Theoretic Measures for Feature Selection.
ADAPTIVE AND NATURAL COMPUTING ALGORITHMS, ICANNGA 2013(2013)
摘要
Information-theoretic measures are frequently employed to select the most relevant subset of features from datasets. This paper focuses on the analysis of continuous-valued features. We compare the common approach with discretization of features prior the analysis, to the direct usage of exact values. Due to the overwhelming costs of computing continuous information-theoretic measures based on Shannon entropy the Renyi and Tsallis generalized measures are considered. To enable computation with continuous Tsallis measures a novel modification of the information potential is introduced. The quality of the analysed measures was assessed indirectly through the classification accuracy in conjuction with the greedy feature selection process. The experiments on datasets from UCI repository show considerable improvements of the results when using both generalized continuous measures.
更多查看译文
关键词
Feature selection,Information theory,Renyi entropy,Tsallis entropy
AI 理解论文
溯源树
样例
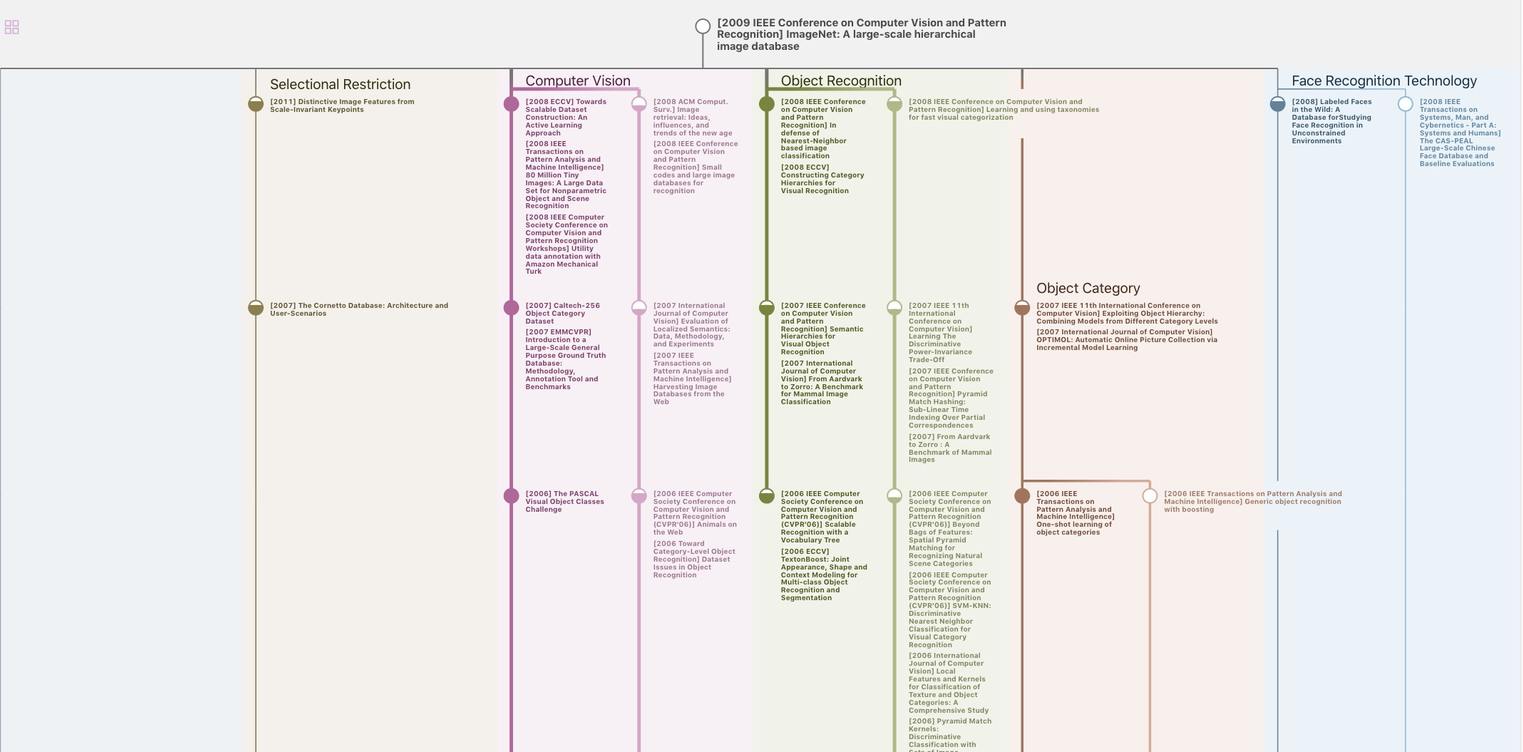
生成溯源树,研究论文发展脉络
Chat Paper
正在生成论文摘要