Online topic detection, tracking, and significance ranking using generative topic models
Online topic detection, tracking, and significance ranking using generative topic models(2009)
摘要
Online processing of text streams is an essential task of many legitimate applications. The objective is to deconstruct the documents into semantically coherent threads or topics, analyze the development of the topics over time, and identify newly emerging topics. This must be accomplished by processing only the textual content and publication time of documents without requiring any metadata, such as hyperlinks or citation data. This dissertation presents an "Online Topic Model" (OLDA), a topic model that automatically captures the thematic patterns and identifies emerging topics of text streams and their changes over time. The proposed approach allows the topic modeling framework, specifically the Latent Dirichlet Allocation (LDA) model, to work in an online fashion such that it incrementally builds an up-to-date model (mixture of topics per document and mixture of words per topic) when a new document (or a set of documents) appears. A solution based on the Empirical Bayes method is proposed. The idea is to incrementally update the current model according to the information inferred from the new stream of data with no need to access previous data. The dynamics of the proposed approach also provide an efficient means to track drifts in topics and detect the emerging topics in real time. As many topics tend to reappear consistently in text streams, incorporating semantics that were discovered in previous streams is expected to enhance the prediction of future topics. Embedding semantic information within the document representation and/or distance metrics has been shown to improve the efficiency of vector space approaches in discovering the semantic structure of the textual data. However, no attempts have been made to embed semantic information to enhance online document modeling within the framework of LDA. This dissertation extends the proposed online topic model to incorporate semantic history propagated from models that were estimated within a "sliding history window." Since the proposed approach is totally unsupervised and data-driven, the effect of different factors is analyzed, including the window size, history weight, and equal/decaying history contribution. In addition, the setting of the number of latent variables is extremely critical and directly affects the quality of the model and the interpretability of the estimated topics. Since the actual number of underlying topics is unknown and there is no definite and efficient approach to accurately estimate it, the inferred topics of any topic model do not always represent meaningful themes. This dissertation presents the first automated unsupervised analysis of LDA models to identify and distinguish junk topics from legitimate ones, and to rank the topics based on their semantic significance. The basic idea consists of measuring the distance between topic distribution and "junk distribution." In particular, three definitions of "junk distribution" are introduced, and a variety of metrics are used to compute the distances, from which an expressive figure of topic significance is implemented using a 4-phase Weighted Linear Combination approach.
更多查看译文
关键词
significance ranking,proposed online topic model,future topic,generative topic model,Online topic detection,current model,topic model,proposed approach,junk topic,LDA model,junk distribution,text stream,estimated topic
AI 理解论文
溯源树
样例
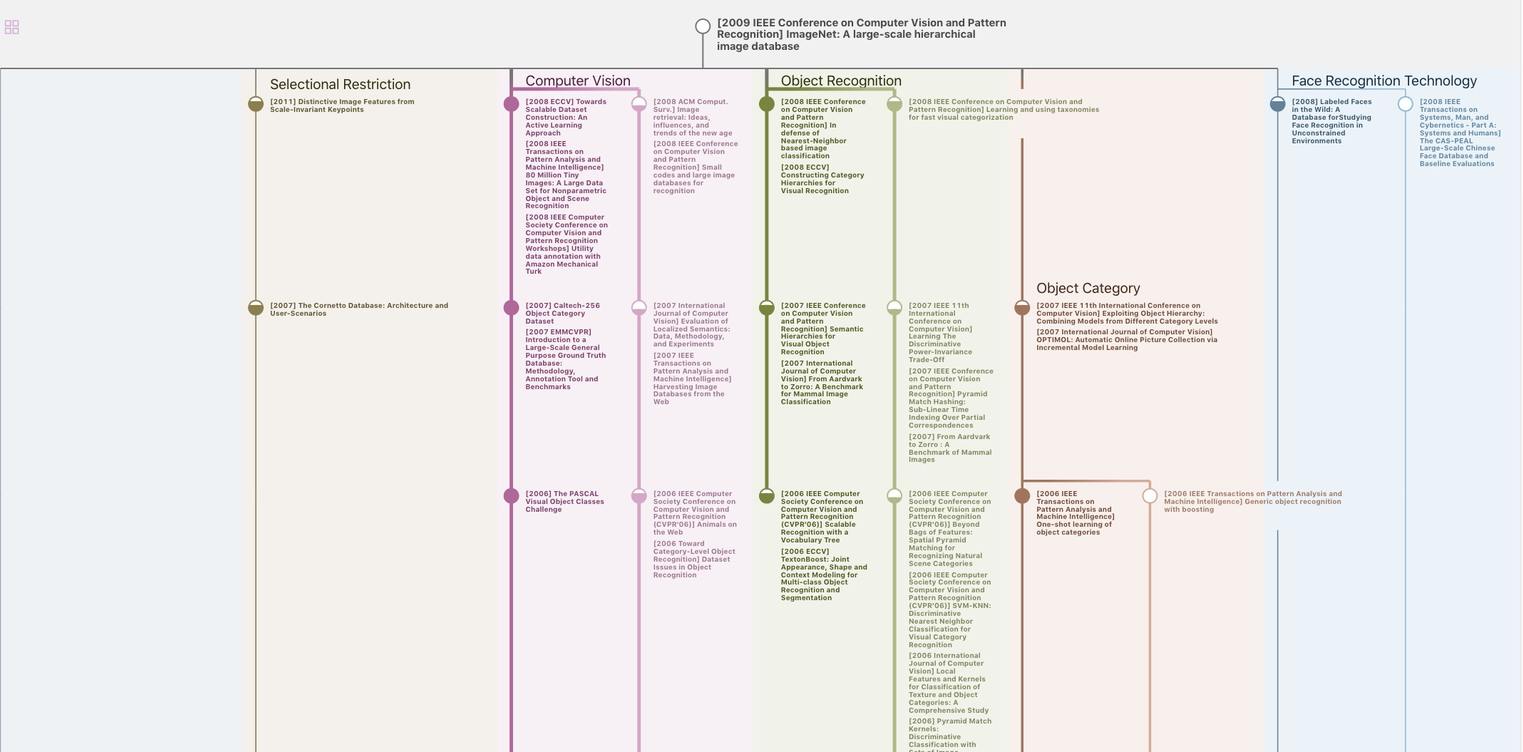
生成溯源树,研究论文发展脉络
Chat Paper
正在生成论文摘要