A sparse-response deep belief network based on rate distortion theory.
Pattern Recognition(2014)
摘要
Deep belief networks (DBNs) are currently the dominant technique for modeling the architectural depth of brain, and can be trained efficiently in a greedy layer-wise unsupervised learning manner. However, DBNs without a narrow hidden bottleneck typically produce redundant, continuous-valued codes and unstructured weight patterns. Taking inspiration from rate distortion (RD) theory, which encodes original data using as few bits as possible, we introduce in this paper a variant of DBN, referred to as sparse-response DBN (SR-DBN). In this approach, Kullback–Leibler divergence between the distribution of data and the equilibrium distribution defined by the building block of DBN is considered as a distortion function, and the sparse response regularization induced by L1-norm of codes is used to achieve a small code rate. Several experiments by extracting features from different scale image datasets show that our approach SR-DBN learns codes with small rate, extracts features at multiple levels of abstraction mimicking computations in the cortical hierarchy, and obtains more discriminative representation than PCA and several basic algorithms of DBNs.
更多查看译文
关键词
Deep belief network,Kullback–Leibler divergence,Information entropy,Rate distortion theory,Unsupervised feature learning
AI 理解论文
溯源树
样例
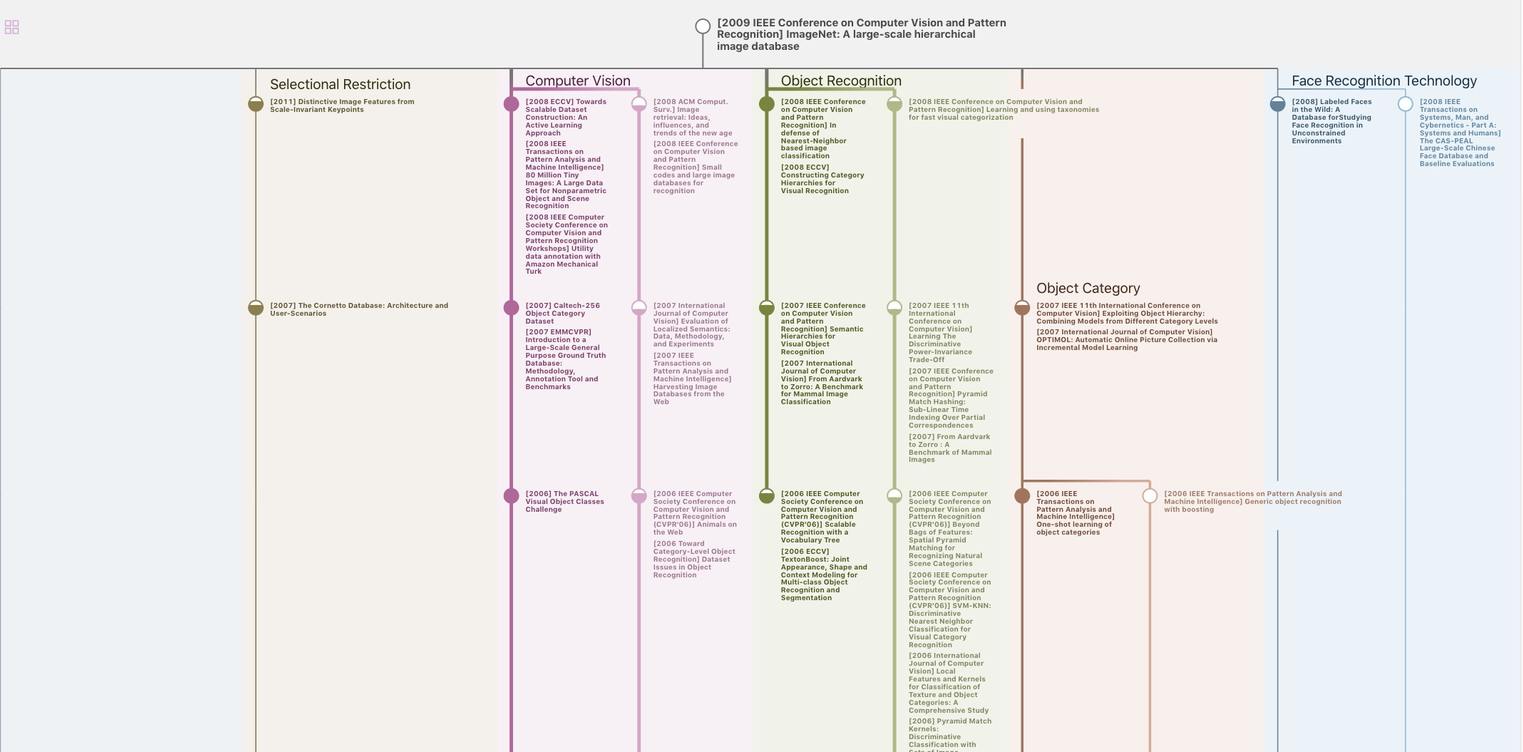
生成溯源树,研究论文发展脉络
Chat Paper
正在生成论文摘要