MULTILEVEL PCA AND INDUCTIVE LEARNING FOR KNOWLEDGE EXTRACTION FROM OPERATIONAL DATA OF BATCH PROCESSES
CHEMICAL ENGINEERING COMMUNICATIONS(2007)
摘要
A new methodology for monitoring batch processes is presented which is based on analysis of historical operational data using both principal component analysis (PCA) and inductive learning. Historical data of batch operations are analysed according to stages. For each stage, PCA is employed to analyse the trajectories of each variable over all batch runs and groups the trajectories into clusters. The first one or two PCs for all variables at a stage are then used in further PCA analysis to project the operation of the stage onto operational spaces. Production rules are generated to summarise the operational routes to produce product recipes, and to describe variables' contributions to stage-wise state spaces. A method for automatic identification of stages using wavelet multi-scale analysis is also described. The methodology is illustrated by reference to a case study of a semi-batch polymerisation reactor.
更多查看译文
关键词
principal component analysis,inductive learning,knowledge discovery,data mining,batch processes,polymerisation
AI 理解论文
溯源树
样例
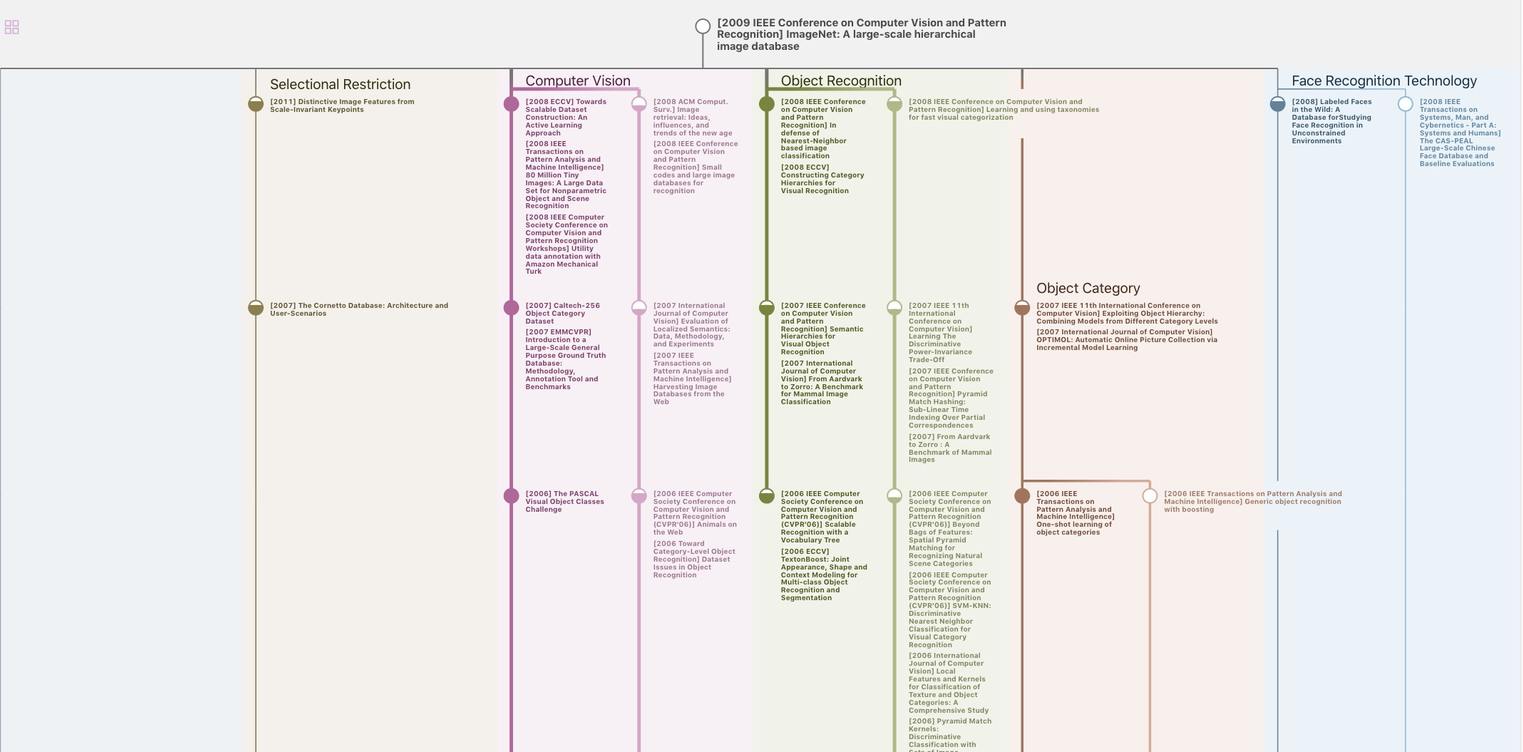
生成溯源树,研究论文发展脉络
Chat Paper
正在生成论文摘要