Reconstruction-free action inference from compressive imagers.
IEEE Transactions on Pattern Analysis and Machine Intelligence(2016)
摘要
Persistent surveillance from camera networks, such as at parking lots, UAVs, etc., often results in large amounts of video data, resulting in significant challenges for inference in terms of storage, communication and computation. Compressive cameras have emerged as a potential solution to deal with the data deluge issues in such applications. However, inference tasks such as action recognition require high quality features which implies reconstructing the original video data. Much work in compressive sensing (CS) theory is geared towards solving the reconstruction problem, where state-of-the-art methods are computationally intensive and provide low-quality results at high compression rates. Thus, reconstruction-free methods for inference are much desired. In this paper, we propose reconstruction-free methods for action recognition from compressive cameras at high compression ratios of 100 and above. Recognizing actions directly from CS measurements requires features which are mostly nonlinear and thus not easily applicable. This leads us to search for such properties that are preserved in compressive measurements. To this end, we propose the use of spatio-temporal smashed filters, which are compressive domain versions of pixel-domain matched filters. We conduct experiments on publicly available databases and show that one can obtain recognition rates that are comparable to the oracle method in uncompressed setup, even for high compression ratios.
更多查看译文
关键词
Correlation,Cameras,Image coding,Training,Three-dimensional displays,Image reconstruction,Optical sensors
AI 理解论文
溯源树
样例
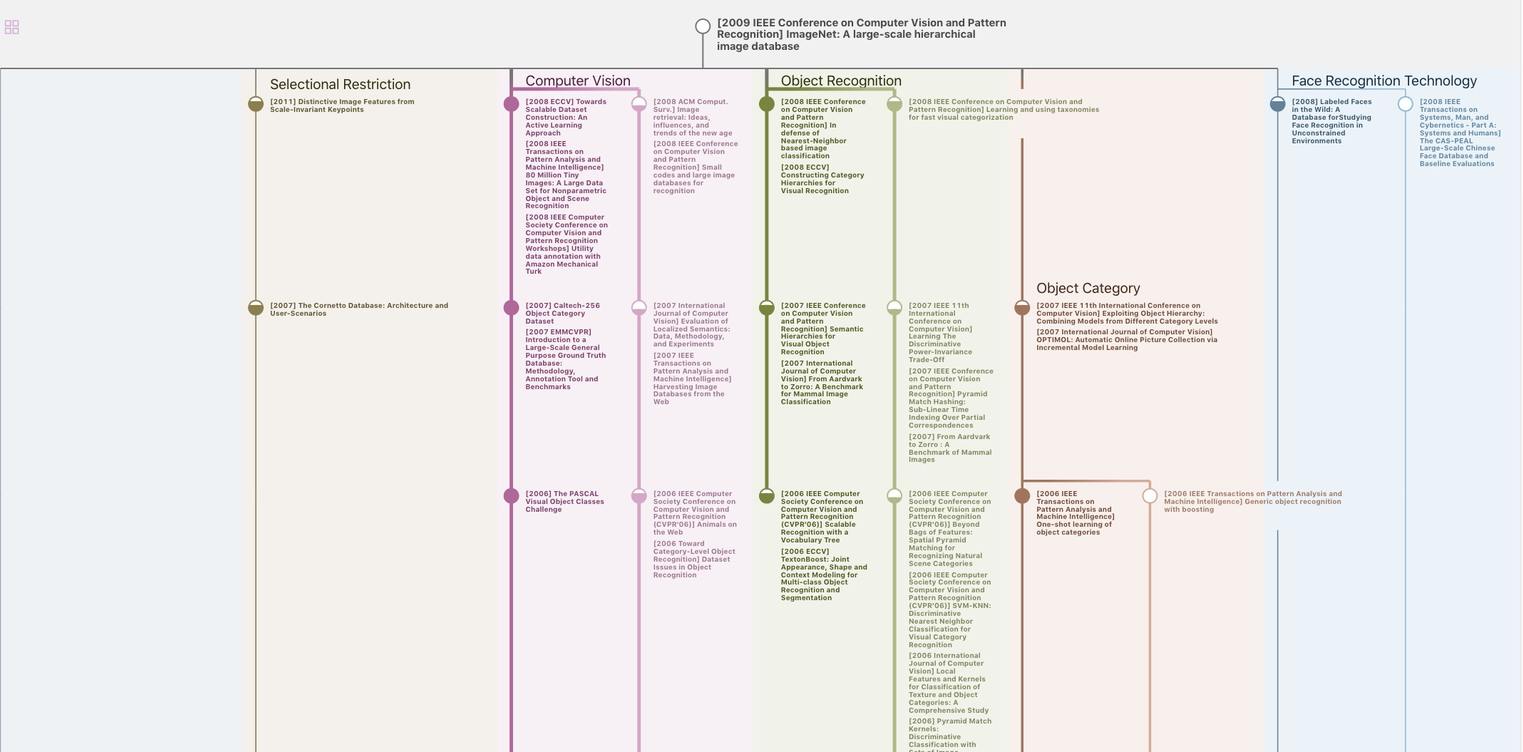
生成溯源树,研究论文发展脉络
Chat Paper
正在生成论文摘要