Faster Subset Selection for Matrices and Applications.
SIAM JOURNAL ON MATRIX ANALYSIS AND APPLICATIONS(2013)
摘要
We study the following problem of subset selection for matrices: given a matrix X is an element of R-nxm (m > n) and a sampling parameter k (n <= k <= m), select a subset of k columns from X such that the pseudoinverse of the sampled matrix has as small a norm as possible. In this work, we focus on the Frobenius and the spectral matrix norms. We describe several novel (deterministic and randomized) approximation algorithms for this problem with approximation bounds that are optimal up to constant factors. Additionally, we show that the combinatorial problem of finding a low-stretch spanning tree in an undirected graph corresponds to subset selection, and discuss various implications of this reduction.
更多查看译文
关键词
subset selection,low-stretch spanning trees,volume sampling,low-rank approximations,k-means clustering,feature selection,sparse approximation
AI 理解论文
溯源树
样例
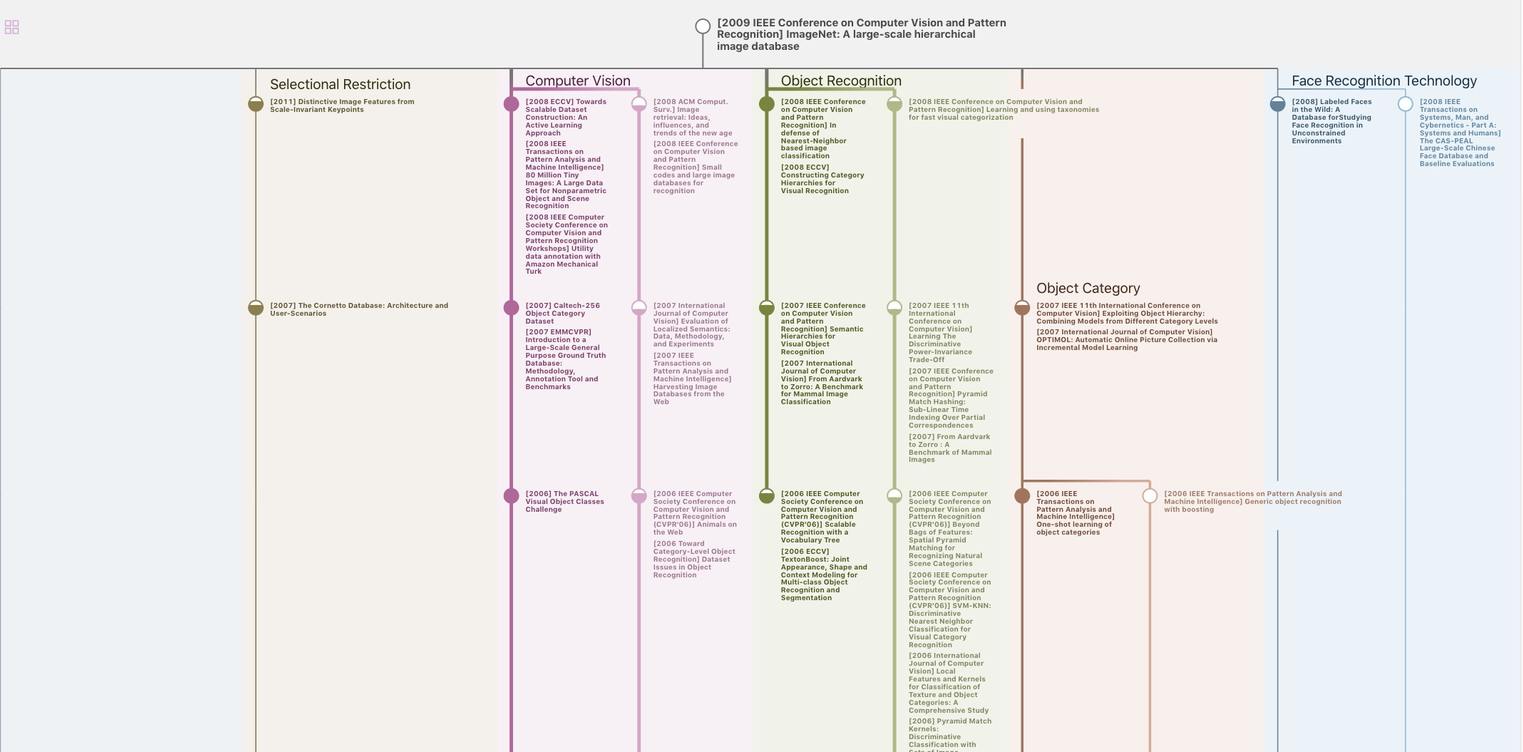
生成溯源树,研究论文发展脉络
Chat Paper
正在生成论文摘要