Evaluating Models of Latent Document Semantics in the Presence of OCR Errors.
EMNLP '10: Proceedings of the 2010 Conference on Empirical Methods in Natural Language Processing(2010)
摘要
Models of latent document semantics such as the mixture of multinomials model and Latent Dirichlet Allocation have received substantial attention for their ability to discover topical semantics in large collections of text. In an effort to apply such models to noisy optical character recognition (OCR) text output, we endeavor to understand the effect that character-level noise can have on unsupervised topic modeling. We show the effects both with document-level topic analysis (document clustering) and with word-level topic analysis (LDA) on both synthetic and real-world OCR data. As expected, experimental results show that performance declines as word error rates increase. Common techniques for alleviating these problems, such as filtering low-frequency words, are successful in enhancing model quality, but exhibit failure trends similar to models trained on unprocessed OCR output in the case of LDA. To our knowledge, this study is the first of its kind.
更多查看译文
关键词
document-level topic analysis,real-world OCR data,unprocessed OCR output,unsupervised topic modeling,word-level topic analysis,document clustering,latent document semantics,model quality,multinomials model,text output,OCR error
AI 理解论文
溯源树
样例
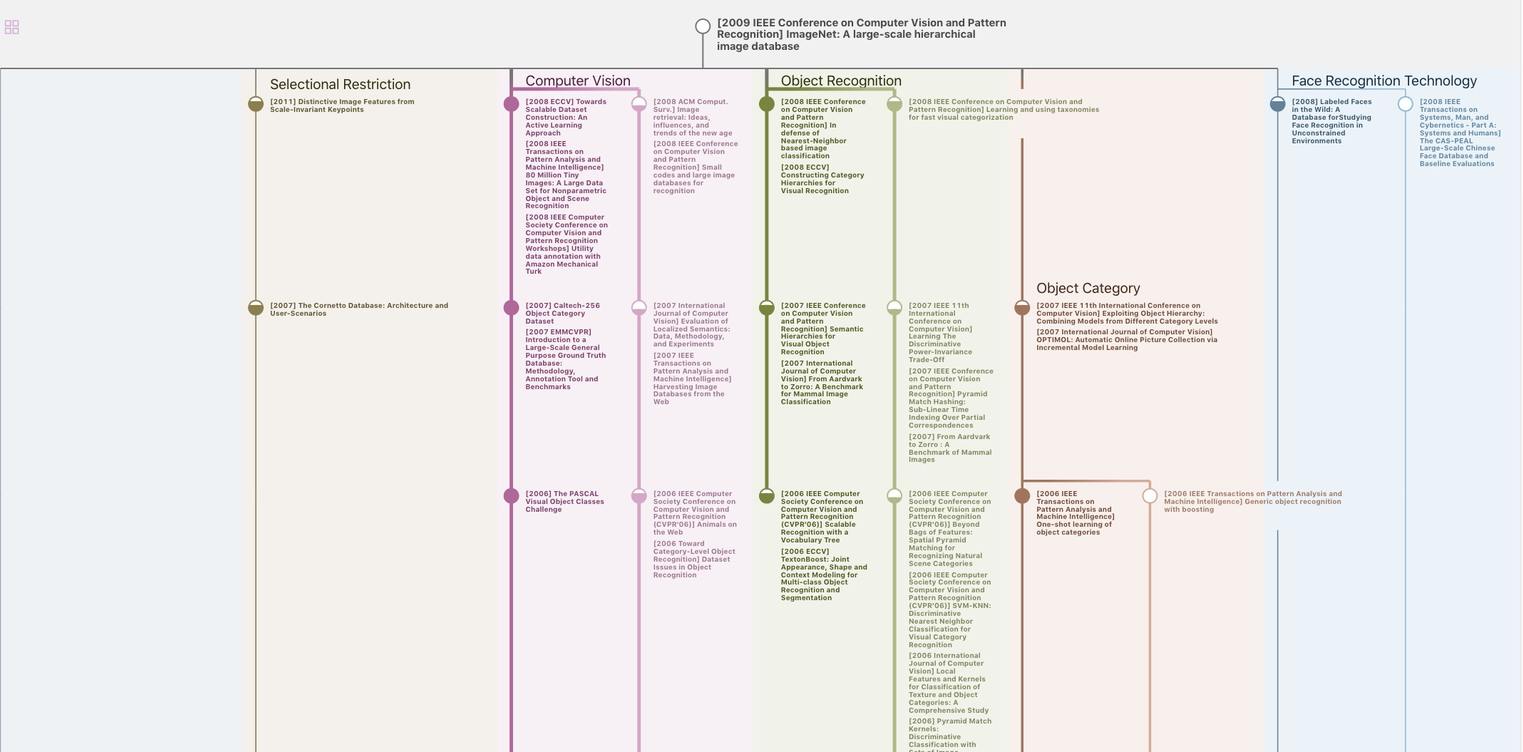
生成溯源树,研究论文发展脉络
Chat Paper
正在生成论文摘要