On the regularization of image semantics by modal expansion
CVPR(2012)
摘要
Recent research efforts in semantic representations and context modeling are based on the principle of task expansion: that vision problems such as object recognition, scene classification, or retrieval (RCR) cannot be solved in isolation. The extended principle of modality expansion (that RCR problems cannot be solved from visual information alone) is investigated in this work. A semantic image labeling system is augmented with text. Pairs of images and text are mapped to a semantic space, and the text features used to regularize their image counterparts. This is done with a new cross-modal regularizer, which learns the mapping of the image features that maximizes their average similarity to those derived from text. The proposed regularizer is class-sensitive, combining a set of class-specific denoising transformations and nearest neighbor interpolation of text-based class assignments. Regularization of a state-of-the-art approach to image retrieval is then shown to produce substantial gains in retrieval accuracy, outperforming recent image retrieval approaches.
更多查看译文
关键词
retrieval accuracy,extended principle,semantic space,semantic representation,image feature,image retrieval,semantic image,recent image retrieval approach,modal expansion,RCR problem,image semantics,image counterpart
AI 理解论文
溯源树
样例
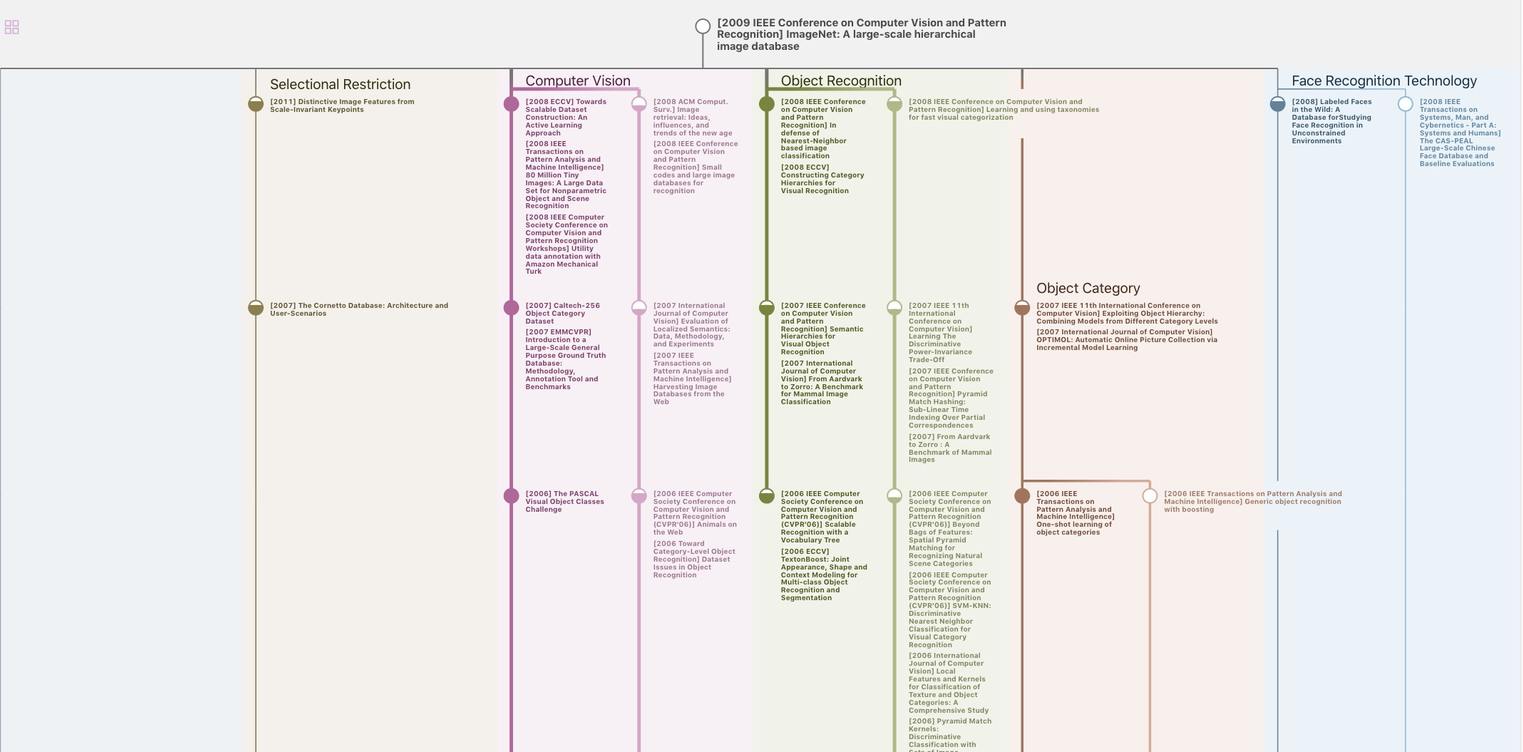
生成溯源树,研究论文发展脉络
Chat Paper
正在生成论文摘要