Learning Faster Than Promised By The Vapnik-Chervonenkis Dimension
DISCRETE APPLIED MATHEMATICS(1989)
摘要
We investigate the sample size needed to infer a separating line between two convex planar regions using Valiant's model of the complexity of learning from random examples [4]. A theorem proved in [1] using the Vapnik-Chervonenkis dimension gives an O((1/ε)ln(1/ε)) upper bound on the sample size sufficient to infer a separating line with error less than ε between two convex planar regions. This theorem requires that with high probability any separating line consistent with such a sample have small error. The present paper gives a lower bound showing that under this requirement the sample size cannot be improved. It is further shown that if this requirement is weakened to require only that a particular line which is tangent to the convex hulls of the sample points in the two regions have small error then the ln(1/ε) term can be eliminated from the upper bound.
更多查看译文
AI 理解论文
溯源树
样例
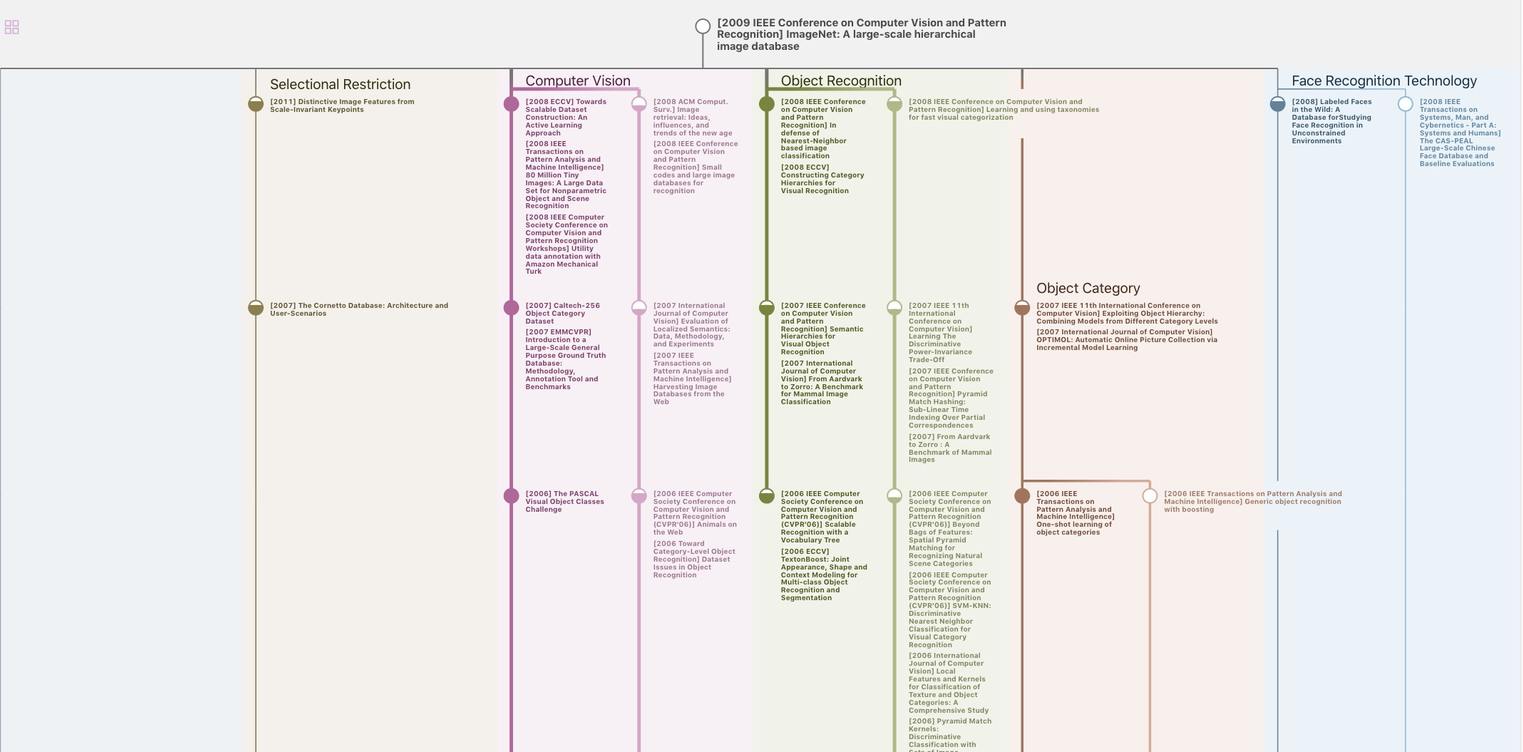
生成溯源树,研究论文发展脉络
Chat Paper
正在生成论文摘要