A Bayesian Approach to Unsupervised One-Shot Learning of Object Categories
ICCV(2003)
摘要
Learning visual models of object categories notoriously requiresthousands of training examples; this is due to thediversity and richness of object appearance which requiresmodels containing hundreds of parameters. We present amethod for learning object categories from just a few images(1 ~ 5).It is based on incorporating "generic"knowledge which may be obtained from previously learntmodels of unrelated categories. We operate in a variationalBayesian framework: object categories are represented byprobabilistic models, and "prior" knowledge is representedas a probability density function on the parameters of thesemodels. The "posterior" model for an object category is obtainedby updating the prior in the light of one or more observations.Our ideas are demonstrated on four diverse categories(human faces, airplanes, motorcycles, spotted cats).Initially three categories are learnt from hundreds of trainingexamples, and a "prior" is estimated from these. Thenthe model of the fourth category is learnt from 1 to 5 trainingexamples, and is used for detecting new exemplars a setof test images.
更多查看译文
关键词
machine vision,expectation maximization,bayesian approach,markov processes,computer vision,digamma function,probability density function,maximum likelihood,probabilistic model,bayesian method,face recognition,monte carlo methods,unsupervised learning
AI 理解论文
溯源树
样例
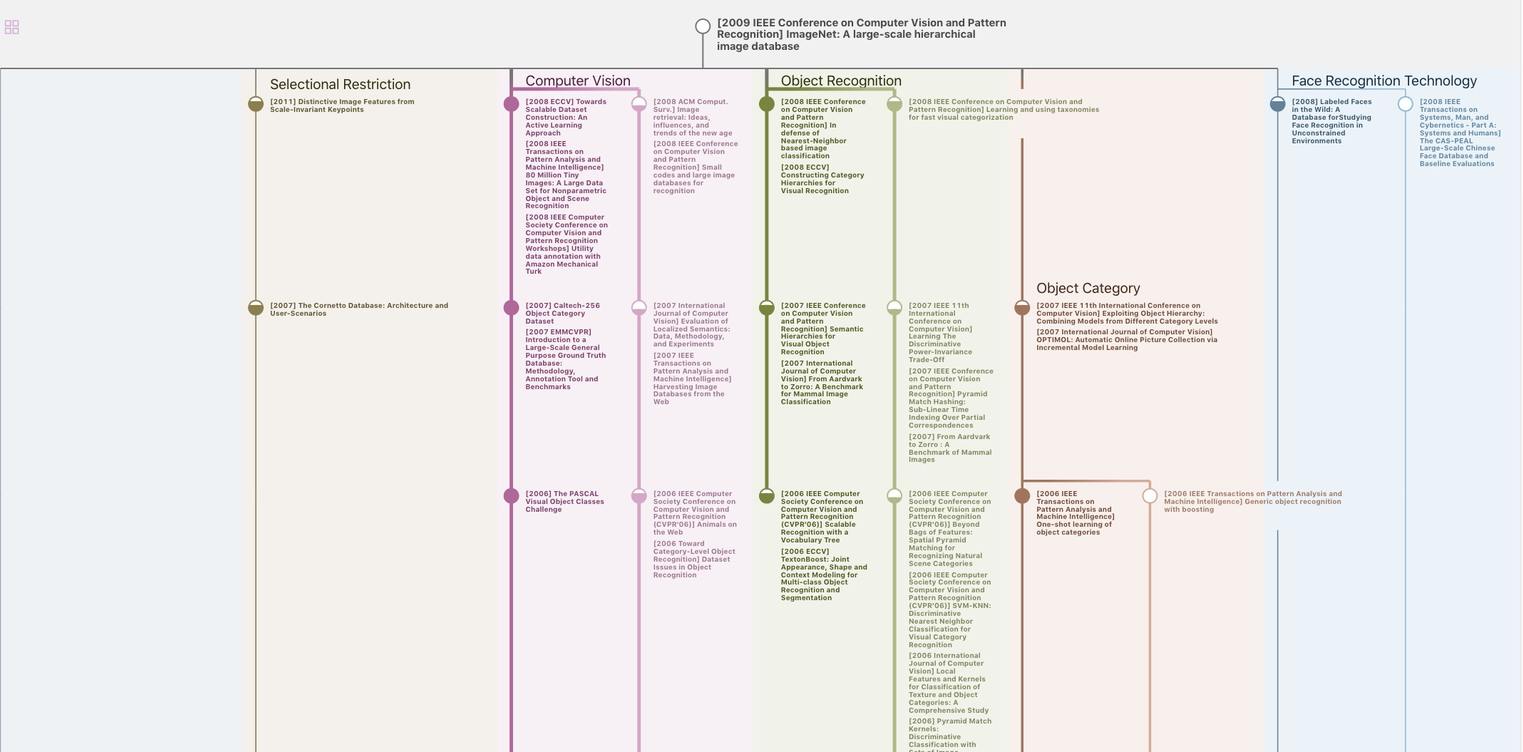
生成溯源树,研究论文发展脉络
Chat Paper
正在生成论文摘要