Visual tracking using sequential importance sampling with a state partition technique
ICIP (3)(2005)
摘要
Sequential importance sampling (SIS), also known as particle filtering, has drawn increasing attention recently due to its superior performance in nonlinear and non-Gaussian dynamic problems. In the SIS framework, estimation accuracy depends strongly on the choice of proposal distribution. In this paper we propose a novel SIS algorithm called PF-SP-PEKF that is based on a state partition technique and a parallel bank of extended Kalman filters designed to improve the accuracy of the proposal distribution. Our results show that this new approach yields a significantly improved estimate of the state, enabling the new particle filter to effectively track human subjects in a video sequence where the standard condensation filter fails to maintain track lock. Moreover, because of the improved proposal distribution, the new filter can achieve a given level of performance using fewer particles than its conventional SIS counterparts.
更多查看译文
关键词
video signal processing,particle filtering (numerical methods),visual tracking,kalman filters,particle filtering,image sampling,tracking,image sequences,sequential importance sampling,extended kalman filters,state partition technique,video sequence,particle filter,extended kalman filter
AI 理解论文
溯源树
样例
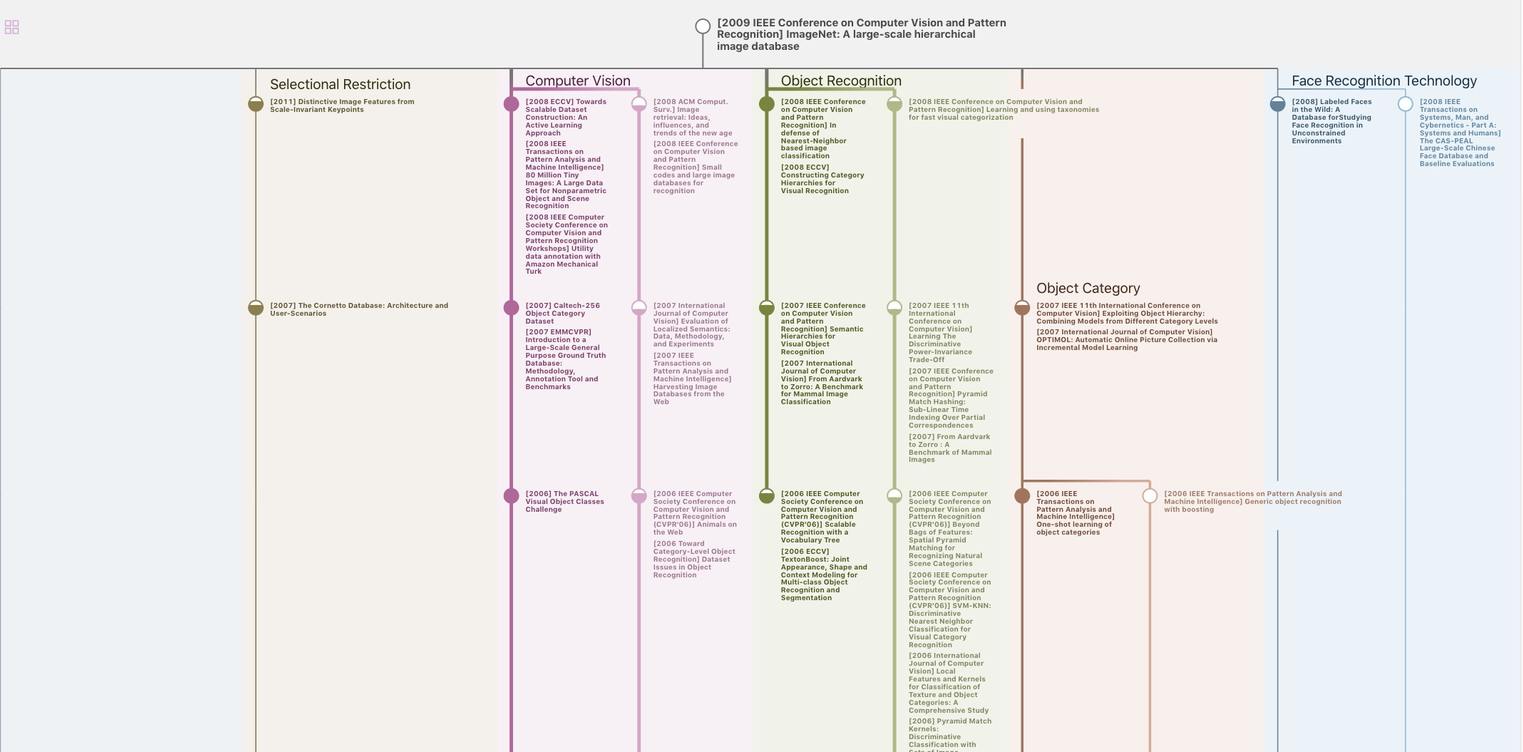
生成溯源树,研究论文发展脉络
Chat Paper
正在生成论文摘要