Distributed Constrained Optimization Over Noisy Networks
49TH IEEE CONFERENCE ON DECISION AND CONTROL (CDC)(2010)
摘要
In this paper we deal with two problems which are of great interest in the field of distributed decision making and control. The first problem we tackle is the problem of achieving consensus on a vector of local decision variables in a network of computational agents when the decision variables of each node are constrained to lie in a subset of the Euclidean space. We assume that the constraint sets for the local variables are private information for each node. We provide a distributed algorithm for the case when there is communication noise present in the network. We show that we can achieve almost sure convergence under certain assumptions. The second problem we discuss is the problem of distributed constrained optimization when the constraint sets are distributed over the agents. Furthermore our model incorporates the presence of noisy communication links and the presence of stochastic errors in the evaluation of subgradients of the local objective function. We establish sufficient conditions and provide an analysis guaranteeing the convergence of the algorithm to the optimal set with probability one.
更多查看译文
关键词
distributed algorithms,objective function,almost sure convergence,random variables,constrained optimization,convergence,euclidean space,noise measurement,private information,algorithm design and analysis,optimization,distributed algorithm,noise
AI 理解论文
溯源树
样例
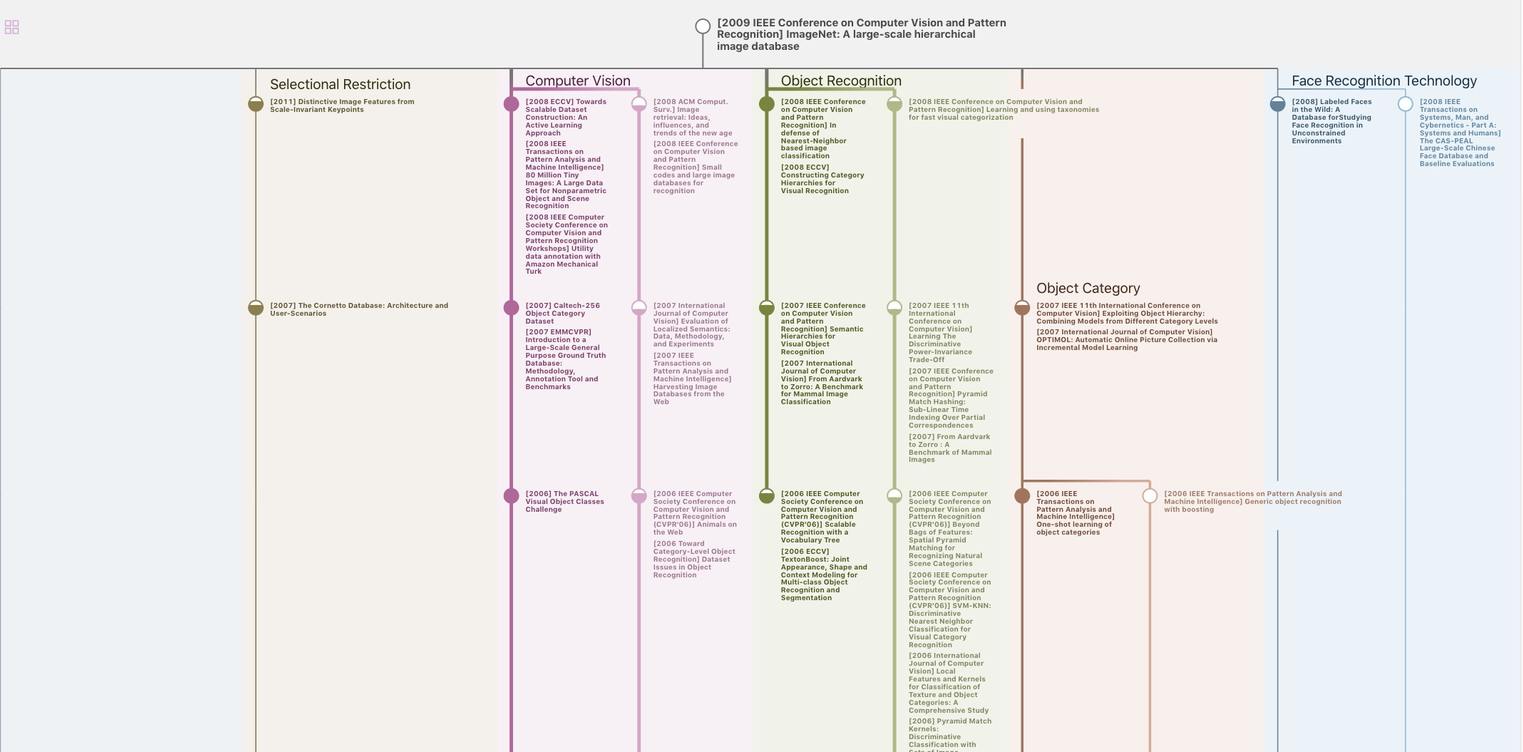
生成溯源树,研究论文发展脉络
Chat Paper
正在生成论文摘要