Ranking Anomalies In Data Centers
2012 IEEE NETWORK OPERATIONS AND MANAGEMENT SYMPOSIUM (NOMS)(2012)
摘要
Data centers are growing in size and complexity driven by trends such as cloud computing and on-line services. Such large data centers pose several challenges for system management. Key among them is anomaly detection which is required to monitor and analyze metrics across several thousands servers and across multiple layers of abstractions to detect anomalous system behavior. In practice, multiple anomaly detection tools are used to continuously raise alarms across multiple metrics and servers. These alarms include both true positives and false alarms. Administrators and management tools act on these alarms for diagnosis and deeper root cause analysis and take appropriate management actions to mitigate the anomalous behaviors. Given the scale and scope of the system, the administrators and management tools are overwhelmed with the large number of alarms at any given instant, many of which are false alarms. It is therefore necessary to prioritize and rank these alarms, so as to take timely actions that maintain the service level agreements for the data center. Existing techniques for such ranking are ad-hoc and not scalable. We propose ranking windows of monitored metrics based on their probability of occurrence. We explain how these probabilities can be computed based either on the false positive rates for which the accompanying anomaly detectors were designed, or, when available, on the probability models underlying the false positive rates. In the simplest case, the ranking procedure reduces to computing the Z-score of the observed measurements and computing a statistic from a window of Z-scores to use as a basis for ranking. The proposed techniques are reliable, lightweight and easy to deploy in the modern data center. We have validated these techniques on synthetic data containing injected anomalies and on data acquired from production data centers.
更多查看译文
关键词
system management,servers,anomaly detection,false positive rate,measurement,time series analysis,root cause analysis,synthetic data,data centers,probability,cloud computing,data center,system monitoring,detectors
AI 理解论文
溯源树
样例
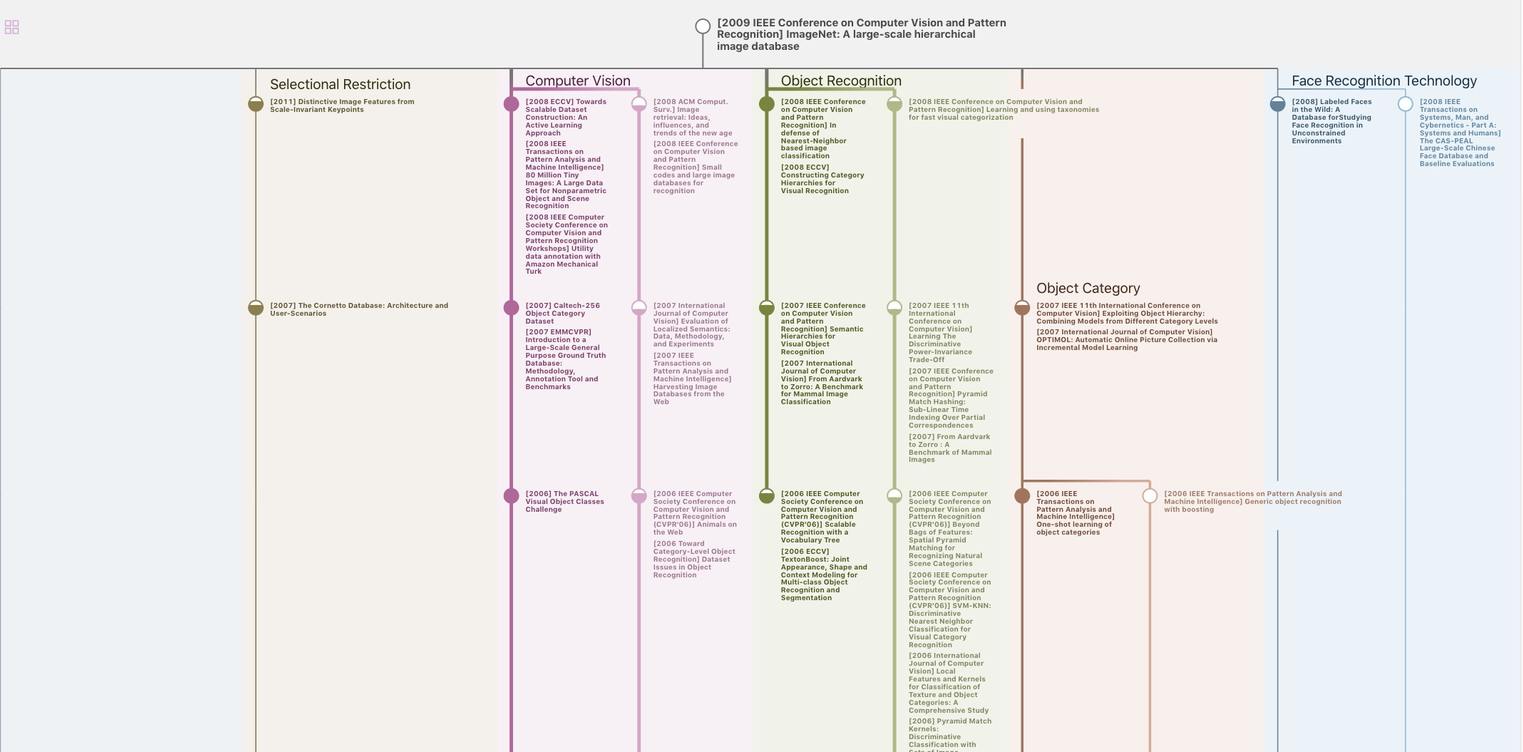
生成溯源树,研究论文发展脉络
Chat Paper
正在生成论文摘要