Robust Music Signal Separation Based On Supervised Nonnegative Matrix Factorization With Prevention Of Basis Sharing
2013 IEEE INTERNATIONAL SYMPOSIUM ON SIGNAL PROCESSING AND INFORMATION TECHNOLOGY (IEEE ISSPIT 2013)(2013)
摘要
In this paper, we address a monaural source separation problem and propose a new penalized supervised nonnegative matrix factorization (SNMF). Conventional SNMF often degrades the separation performance owing to the basissharing problem between supervised bases and nontarget bases. To solve this problem, we employ two types of penalty term based on orthogonality and divergence maximization in the cost function to force the nontarget bases to become as different as possible from the supervised bases. From the experimental results, it can be confirmed that the proposed method prevents the simultaneous generation of similar spectral patterns in the supervised bases and other bases, and increases the separation performance compared with the conventional method.
更多查看译文
关键词
music signal separation,nonnegative matrix factorization,supervised method,basis sharing
AI 理解论文
溯源树
样例
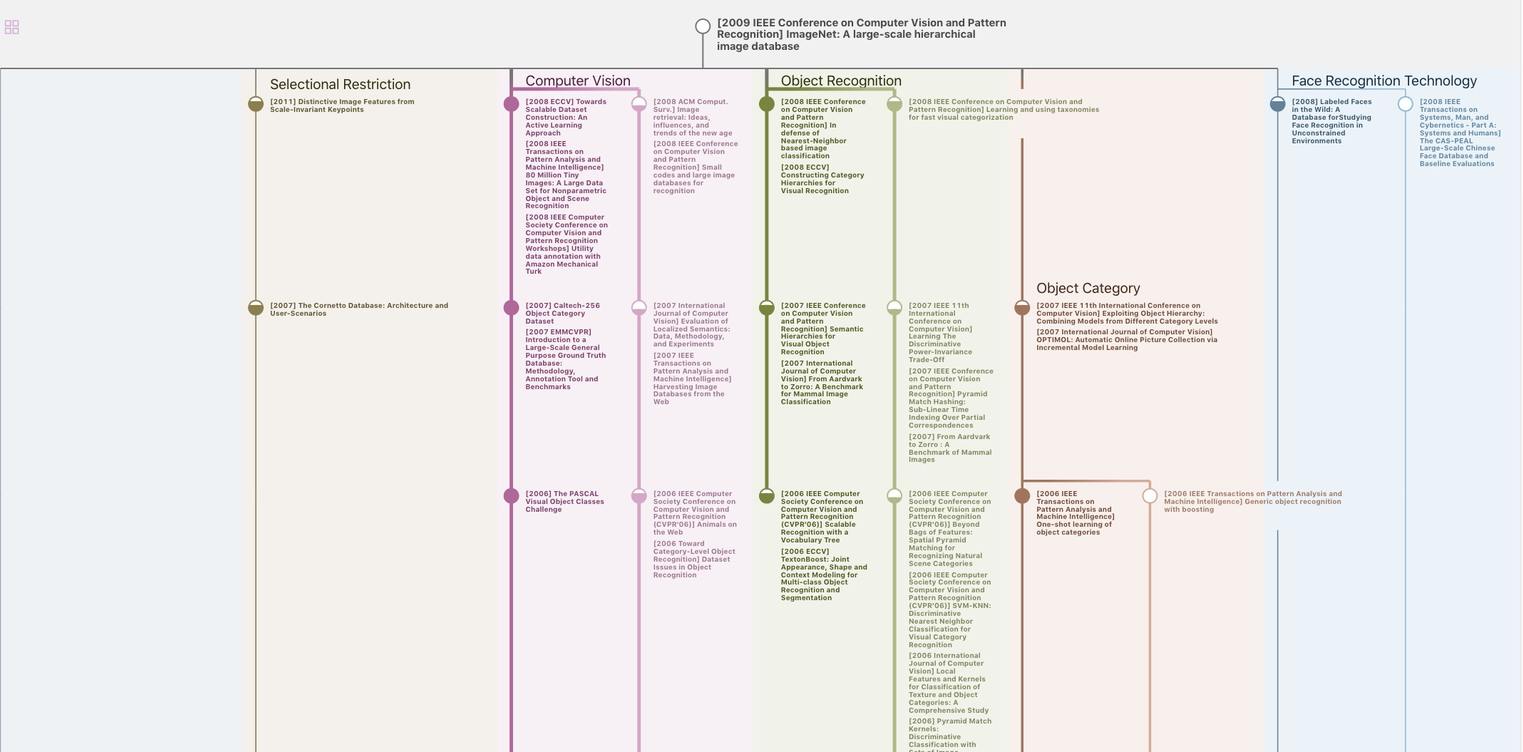
生成溯源树,研究论文发展脉络
Chat Paper
正在生成论文摘要