Efficient Dimensionality Reduction for Canonical Correlation Analysis
SIAM Journal on Scientific Computing(2013)
摘要
We present a fast algorithm for approximate Canonical Correlation Analysis (CCA). Given a pair of tall-and-thin matrices, the proposed algorithm first employs a randomized dimensionality reduction transform to reduce the size of the input matrices, and then applies any CCA algorithm to the new pair of matrices. The algorithm computes an approximate CCA to the original pair of matrices with provable guarantees, while requiring asymptotically less operations than the state-of-the-art exact algorithms.
更多查看译文
关键词
principal angles,canonical correlations,randomized algorithms,dimensionality reduction
AI 理解论文
溯源树
样例
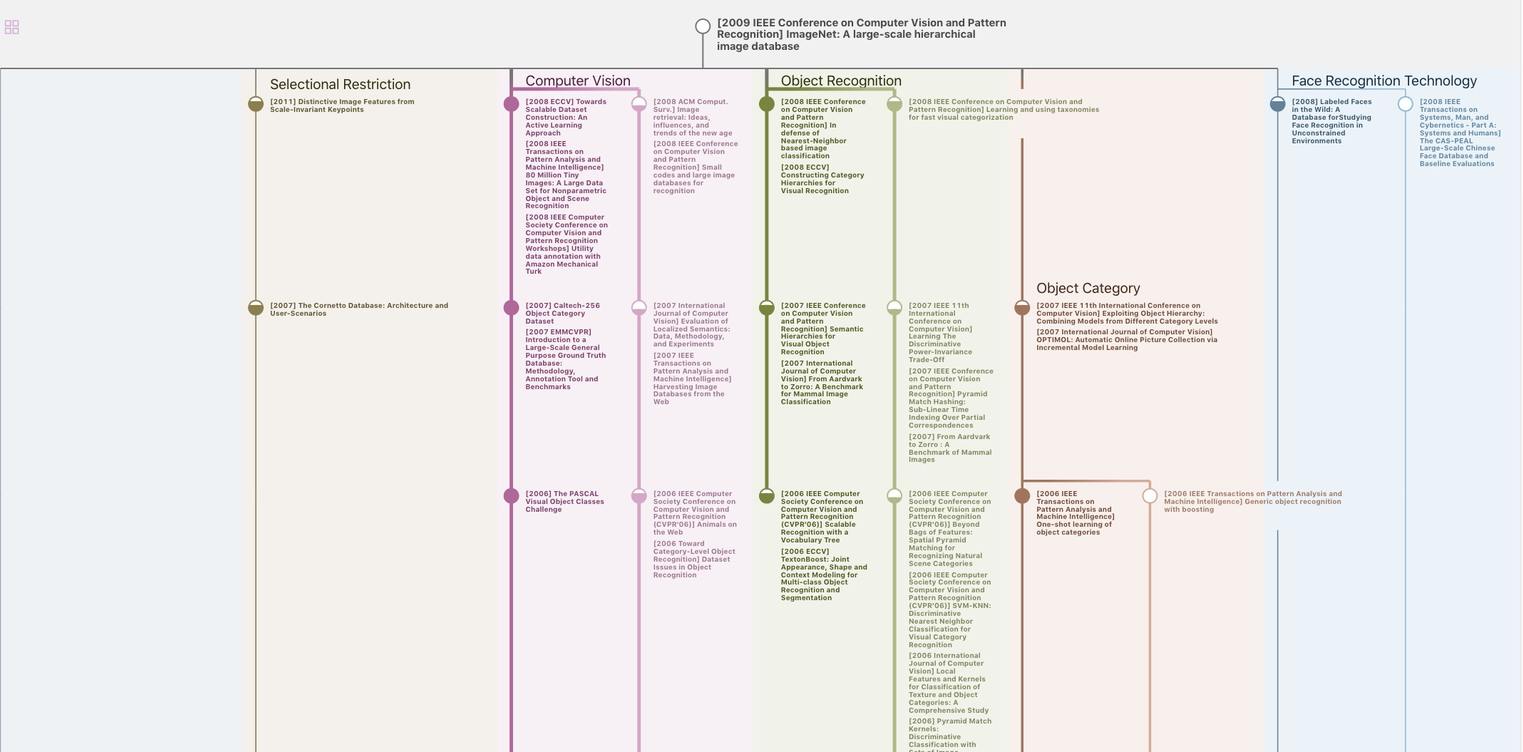
生成溯源树,研究论文发展脉络
Chat Paper
正在生成论文摘要