PowerGraph: distributed graph-parallel computation on natural graphs
OSDI(2012)
摘要
Large-scale graph-structured computation is central to tasks ranging from targeted advertising to natural language processing and has led to the development of several graph-parallel abstractions including Pregel and GraphLab. However, the natural graphs commonly found in the real-world have highly skewed power-law degree distributions, which challenge the assumptions made by these abstractions, limiting performance and scalability. In this paper, we characterize the challenges of computation on natural graphs in the context of existing graph-parallel abstractions. We then introduce the PowerGraph abstraction which exploits the internal structure of graph programs to address these challenges. Leveraging the PowerGraph abstraction we introduce a new approach to distributed graph placement and representation that exploits the structure of power-law graphs. We provide a detailed analysis and experimental evaluation comparing PowerGraph to two popular graph-parallel systems. Finally, we describe three different implementation strategies for PowerGraph and discuss their relative merits with empirical evaluations on large-scale real-world problems demonstrating order of magnitude gains.
更多查看译文
关键词
graph placement,graph program,natural graph,internal structure,natural language processing,powergraph abstraction,large-scale graph-structured computation,large-scale real-world problem,graph-parallel abstraction,graph-parallel computation,popular graph-parallel system
AI 理解论文
溯源树
样例
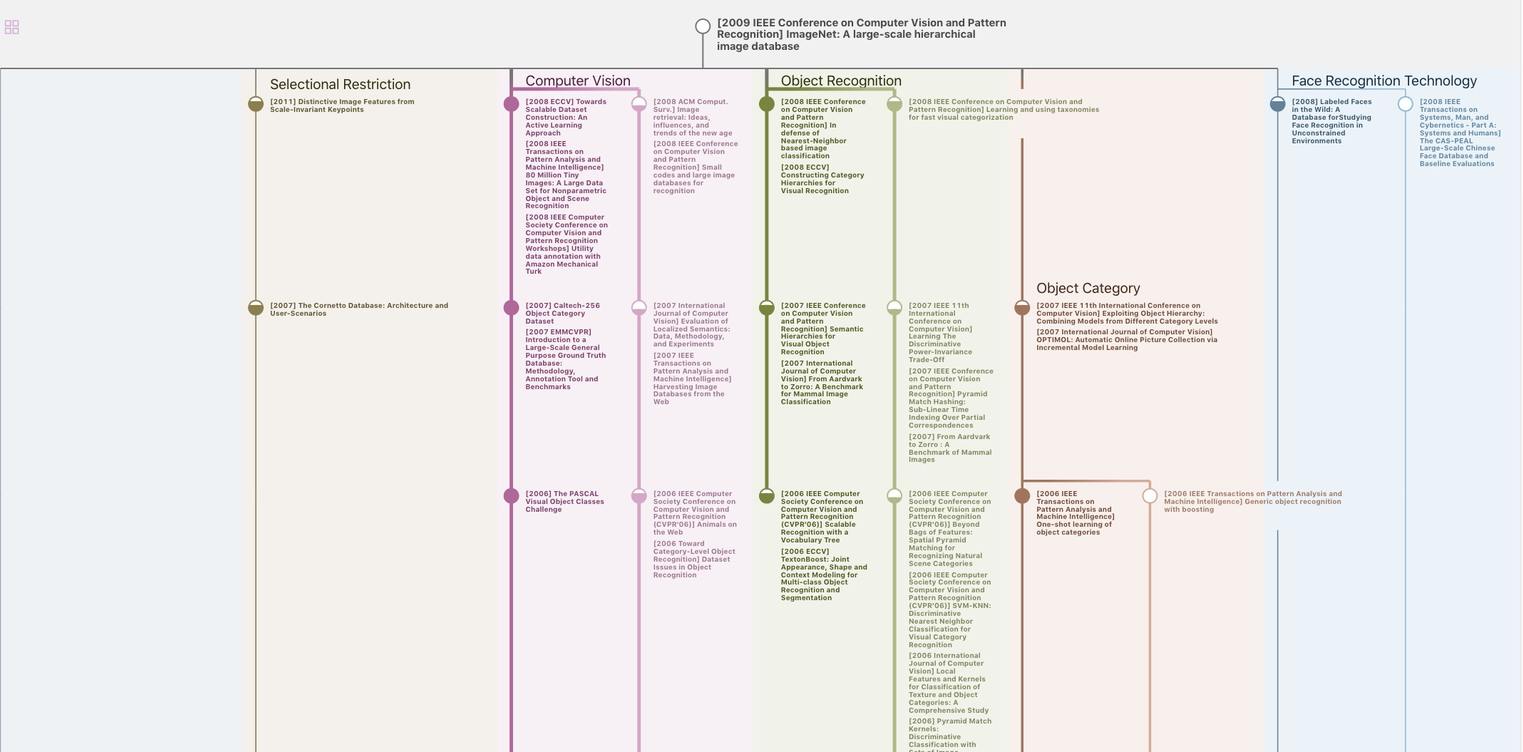
生成溯源树,研究论文发展脉络
Chat Paper
正在生成论文摘要