Localization with multi-modal vision measurements in limited GPS environments using Gaussian sum filters
ICRA(2009)
摘要
A Gaussian Sum Filter (GSF) with component extended Kalman filters (EKF) is proposed as an approach to localize an autonomous vehicle in an urban environment with limited GPS availability. The GSF uses vehicle relative vision-based measurements of known map features coupled with inertial navigation solutions to accomplish localization in the absence of GPS. The vision-based measurements are shown to have multi-modal measurement likelihood functions that are well represented as a weighted sum of Gaussian densities and the GSF is ideally suited to accomplish recursive Bayesian state estimation for this problem. A sequential merging technique is used for Gaussian mixture condensation in the posterior density approximation after fusing multi-modal measurements in the GSF to maintain mixture size over time. The representation of the posterior density with the GSF is compared over a common dataset against a benchmark particle filter solution. The Expectation-Maximization (EM) algorithm is used offline to determine the representational efficiency of the particle filter in terms of an effective number of Gaussian densities. The GSF with vision-based vehicle relative measurements is shown to remain converged using 37 minutes of recorded data from the Cornell University DARPA Urban Challenge (DUC) autonomous vehicle in an urban environment that includes a 32 minute GPS blackout.
更多查看译文
关键词
vision-based vehicle,multi-modal vision measurement,gaussian sum filter,limited gps environment,urban environment,minute gps blackout,autonomous vehicle,gaussian mixture condensation,vehicle relative vision-based measurement,vision-based measurement,gaussian density,limited gps availability,global positioning system,ekf,likelihood function,em algorithm,kalman filters,path planning,expectation maximization,time measurement,remotely operated vehicles,bayesian methods,merging,gaussian processes,extended kalman filter,inertial navigation,mobile robot,particle filters,expectation maximization algorithm,mobile robots,em,particle filter
AI 理解论文
溯源树
样例
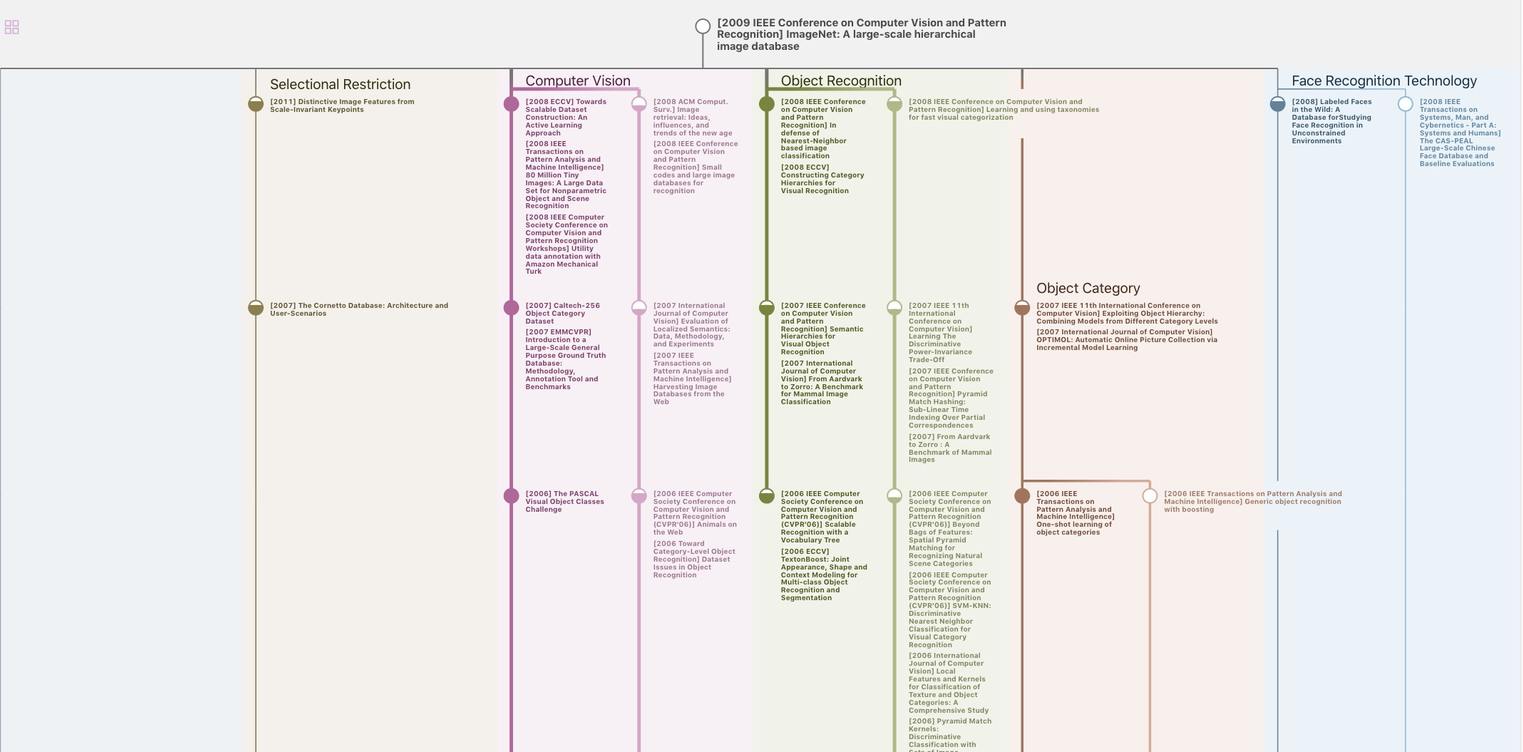
生成溯源树,研究论文发展脉络
Chat Paper
正在生成论文摘要