Validating Network Value Of Influencers By Means Of Explanations
2013 IEEE 13TH INTERNATIONAL CONFERENCE ON DATA MINING (ICDM)(2013)
摘要
Recently, there has been significant interest in social influence analysis. One of the central problems in this area is the problem of identifying influencers, such that by convincing these users to perform a certain action (like buying a new product), a large number of other users get influenced to follow the action. The client of such an application is a marketer who would target these influencers for marketing a given new product, say by providing free samples or discounts. It is natural that before committing resources for targeting an influencer the marketer would be interested in validating the influence (or network value) of influencers returned. This requires digging deeper into such analytical questions as: who are their followers, on what actions (or products) they are influential, etc. However, the current approaches to identifying influencers largely work as a black box in this respect. The goal of this paper is to open up the black box, address these questions and provide informative and crisp explanations for validating the network value of influencers.We formulate the problem of providing explanations (called PROXI) as a discrete optimization problem of feature selection. We show that PROXI is not only NP-hard to solve exactly, it is NP-hard to approximate within any reasonable factor. Nevertheless, we show interesting properties of the objective function and develop an intuitive greedy heuristic. We perform detailed experimental analysis on two real world datasets Twitter and Flixster, and show that our approach is useful in generating concise and insightful explanations of the influence distribution of users and that our greedy algorithm is effective and efficient with respect to several baselines.
更多查看译文
关键词
data mining,marketing,computational complexity,feature selection,greedy algorithms
AI 理解论文
溯源树
样例
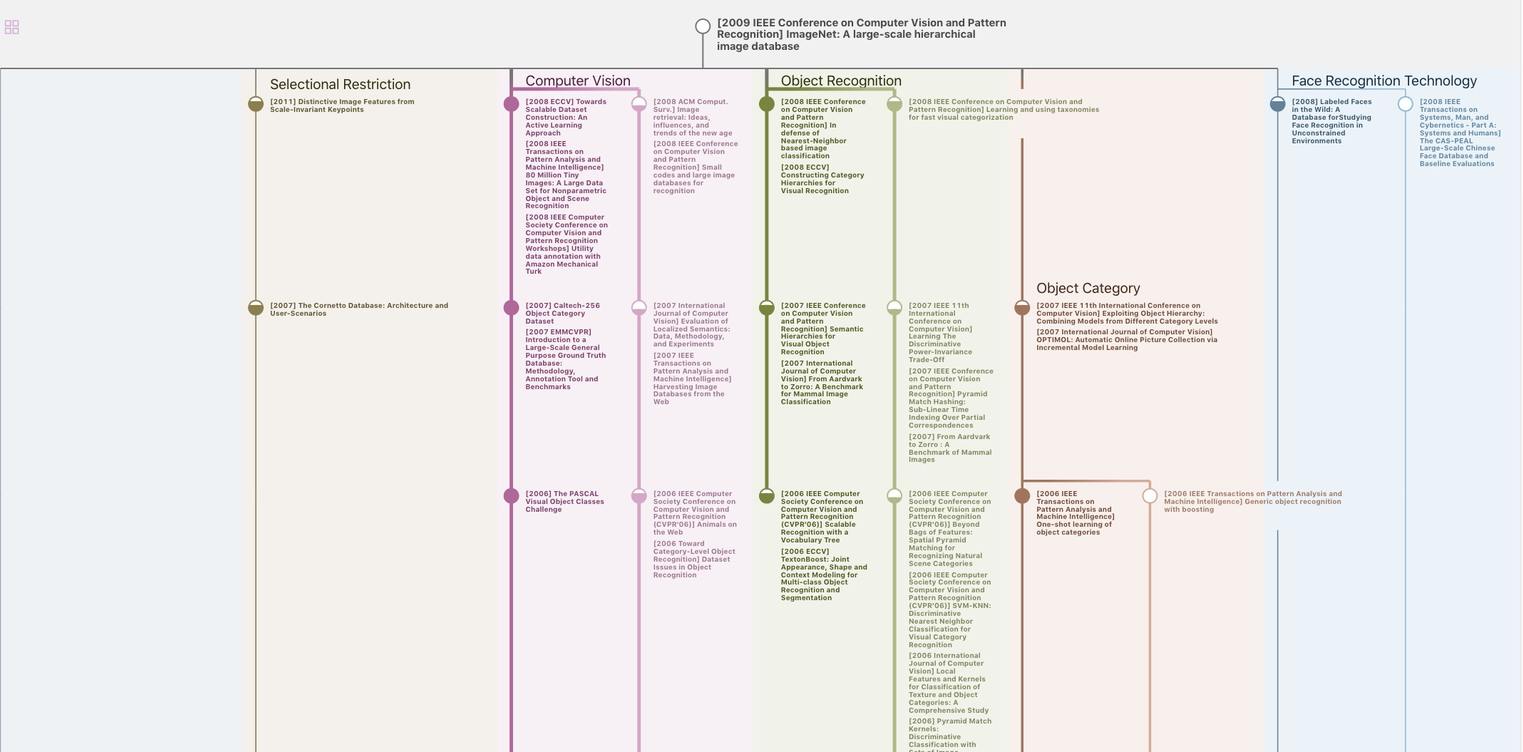
生成溯源树,研究论文发展脉络
Chat Paper
正在生成论文摘要