Variational Bayesian Learning of Directed Graphical Models with Hidden Variables
BAYESIAN ANALYSIS(2006)
摘要
A key problem in statistics and machine learning is inferring suitable structure of a model given some observed data. A Bayesian approach to model comparison makes use of the marginal likelihood of each candidate model to form a posterior distribution over models; unfortunately for most models of interest, notably those containing hidden or latent variables, the marginal likelihood is intractable to compute. We present the variational Bayesian (VB) algorithm for directed graphical models, which optimises a lower bound approximation to the marginal likelihood in a procedure similar to the standard EM algorithm. We show that for a large class of models, which we call conjugate exponential, the VB algorithm is a straightforward generalisation of the EM algorithm that incorporates uncertainty over model parameters. In a thorough case study using a small class of bipartite DAGs containing hidden variables, we compare the accuracy of the VB approximation to existing asymptotic-data approximations such as the Bayesian Information Criterion (BIC) and the Cheeseman-Stutz (CS) criterion, and also to a sampling based gold standard, Annealed Importance Sampling (AIS). We find that the VB algorithm is empirically superior to CS and BIC, and much faster than AIS. Moreover, we prove that a VB approximation can always be constructed in such a way that guarantees it to be more accurate than the CS approximation.
更多查看译文
关键词
Approximate Bayesian Inference,Bayes Factors,Directed Acyclic Graphs,EM Algorithm,Graphical Models,Markov Chain Monte Carlo,Model Selection,Variational Bayes
AI 理解论文
溯源树
样例
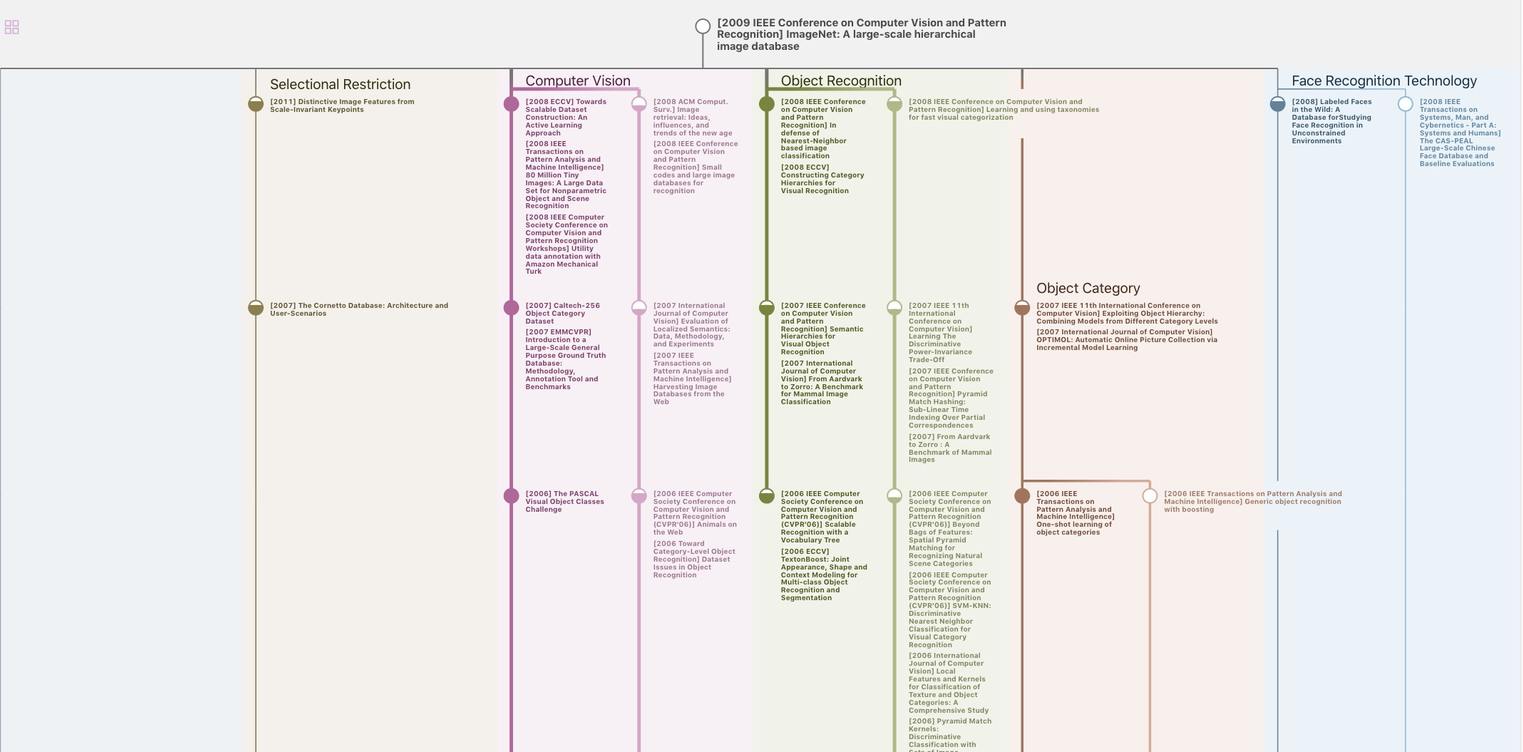
生成溯源树,研究论文发展脉络
Chat Paper
正在生成论文摘要