Domain Adaptation for Structured Regression
International Journal of Computer Vision(2013)
摘要
Discriminative regression models have proved effective for many vision applications (here we focus on 3D full-body and head pose estimation from image and depth data). However, dataset bias is common and is able to significantly degrade the performance of a trained model on target test sets. As we show, covariate shift, a form of unsupervised domain adaptation (USDA), can be used to address certain biases in this setting, but is unable to deal with more severe structural biases in the data. We propose an effective and efficient semi-supervised domain adaptation (SSDA) approach for addressing such more severe biases in the data. Proposed SSDA is a generalization of USDA, that is able to effectively leverage labeled data in the target domain when available. Our method amounts to projecting input features into a higher dimensional space (by construction well suited for domain adaptation) and estimating weights for the training samples based on the ratio of test and train marginals in that space. The resulting augmented weighted samples can then be used to learn a model of choice, alleviating the problems of bias in the data; as an example, we introduce SSDA twin Gaussian process regression (SSDA-TGP) model. With this model we also address the issue of data sharing , where we are able to leverage samples from certain activities (e.g., walking, jogging) to improve predictive performance on very different activities (e.g., boxing). In addition, we analyze the relationship between domain similarity and effectiveness of proposed USDA versus SSDA methods. Moreover, we propose a computationally efficient alternative to TGP (Bo and Sminchisescu 2010 ), and it’s variants, called the direct TGP. We show that our model outperforms a number of baselines, on two public datasets: HumanEva and ETH Face Pose Range Image Dataset. We can also achieve 8–15 times speedup in computation time, over the traditional formulation of TGP, using the proposed direct formulation, with little to no loss in performance.
更多查看译文
关键词
3D pose estimation,Semi-supervised domain adaptation,Covariate shift adaptation
AI 理解论文
溯源树
样例
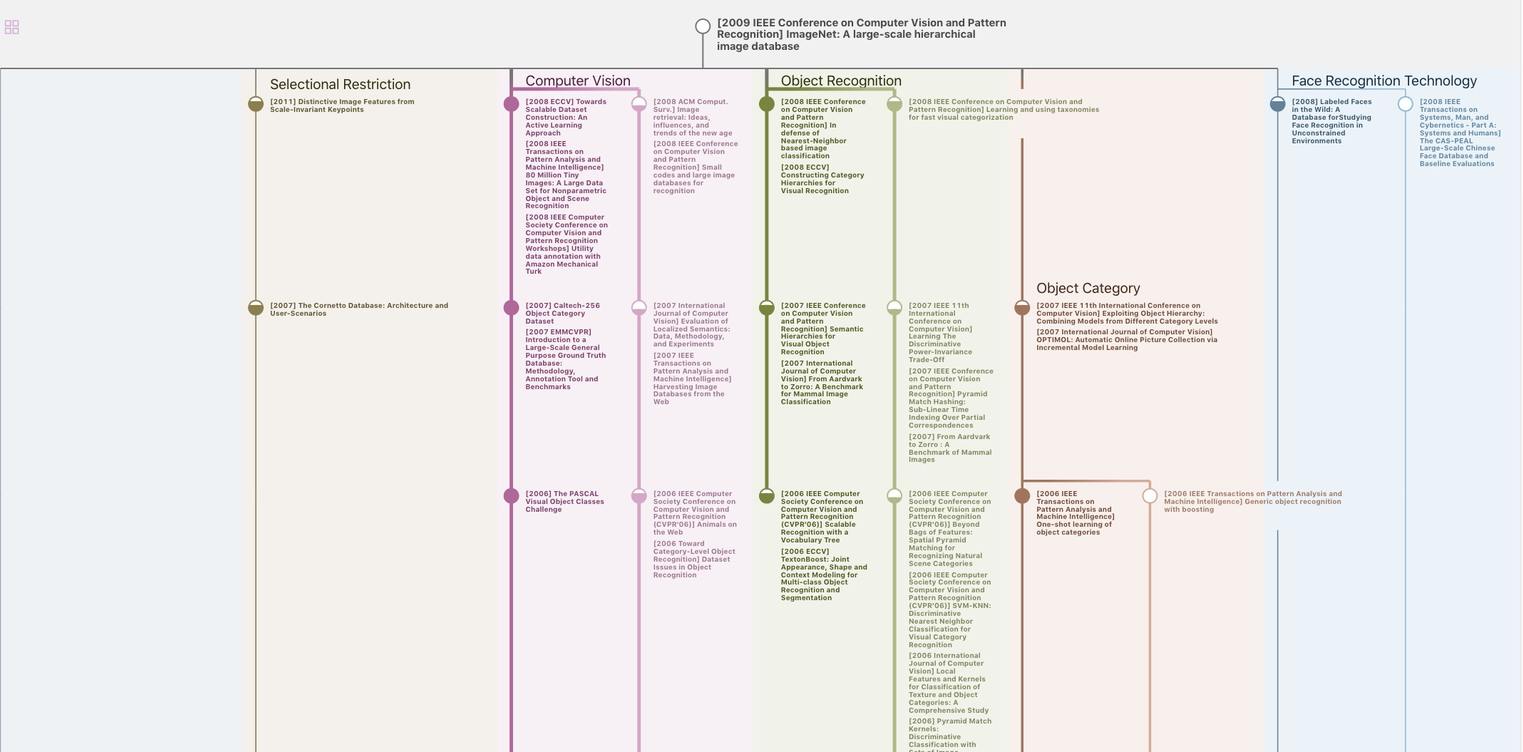
生成溯源树,研究论文发展脉络
Chat Paper
正在生成论文摘要