What Makes Paris Look Like Paris?
international conference on computer graphics and interactive techniques(2012)
摘要
Given a large repository of geotagged imagery, we seek to automatically find visual elements, e. g. windows, balconies, and street signs, that are most distinctive for a certain geo-spatial area, for example the city of Paris. This is a tremendously difficult task as the visual features distinguishing architectural elements of different places can be very subtle. In addition, we face a hard search problem: given all possible patches in all images, which of them are both frequently occurring and geographically informative? To address these issues, we propose to use a discriminative clustering approach able to take into account the weak geographic supervision. We show that geographically representative image elements can be discovered automatically from Google Street View imagery in a discriminative manner. We demonstrate that these elements are visually interpretable and perceptually geo-informative. The discovered visual elements can also support a variety of computational geography tasks, such as mapping architectural correspondences and influences within and across cities, finding representative elements at different geo-spatial scales, and geographically-informed image retrieval.
更多查看译文
关键词
data mining,visual summarization,reference art,big data,computational geography,visual perception
AI 理解论文
溯源树
样例
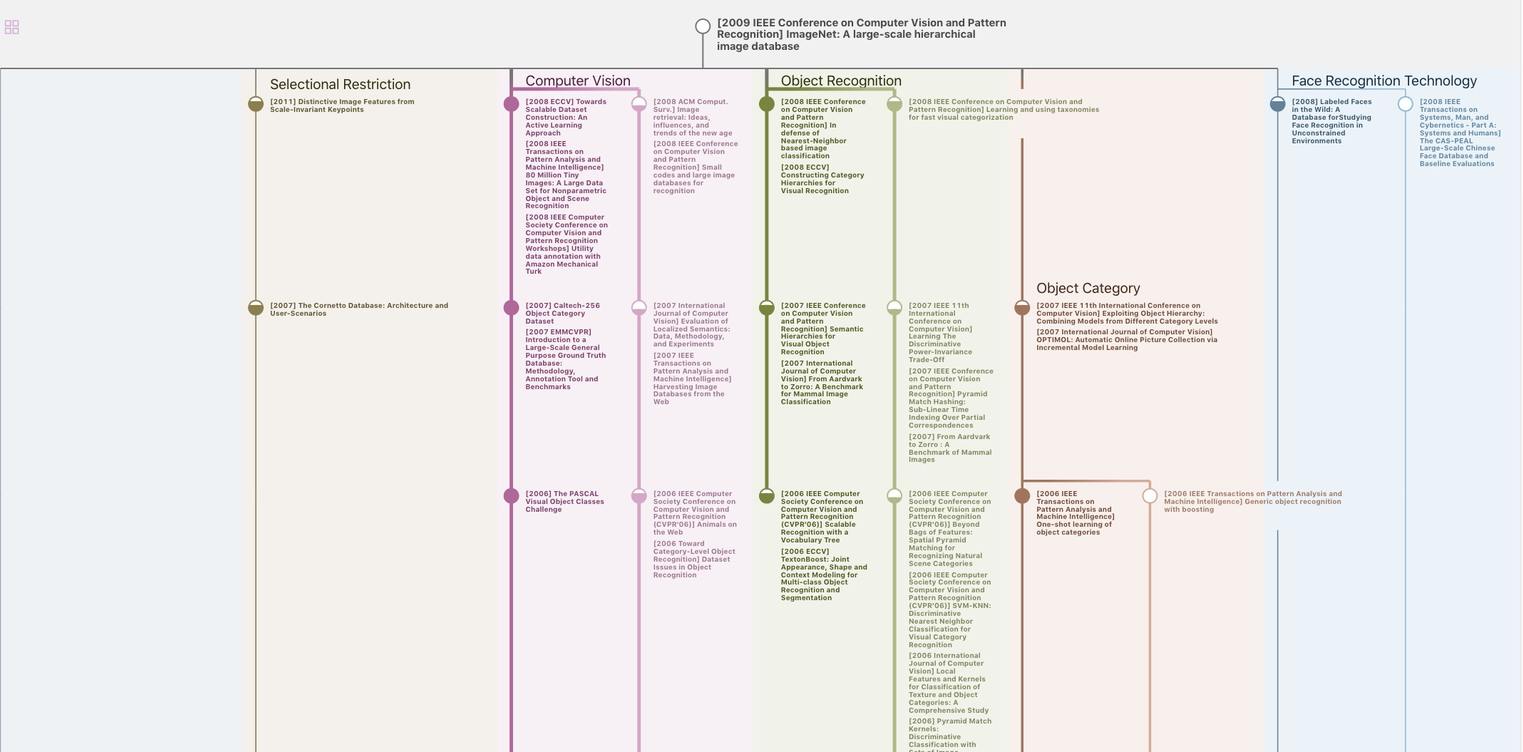
生成溯源树,研究论文发展脉络
Chat Paper
正在生成论文摘要