Fuzzy maximum scatter discriminant analysis with kernel methods
FSKD(2010)
摘要
A novel nonlinear feature extraction method with fuzzy set theory, called KFMSD (kernel based fuzzy maximum scatter difference), is proposed. The proposed method first maps the input data into a potentially much higher dimensional feature space by virtue of nonlinear kernel trick, and thus, the problem of nonlinear feature extraction is overcome. Then, the fuzzy maximum scatter difference is performed on the feature space; therefore, not only the singularity problem of the within-class scatter matrix due to small sample size problem occurred in classical Fisher discriminant analysis is avoided, but also the overlapping (outlier) samples' distribution information is incorporated in the redefinition of corresponding scatter matrices, which is important for classification. The experiment results on the FERET face database and Yale face database show that the proposed method can work well.
更多查看译文
关键词
fuzzy set theory,kfmsd,face recognition,kernel methods,fuzzy maximum scatter discriminant analysis,nonlinear kernel trick,matrix algebra,distribution information,kernel fuzzy maximum scatter difference (kfmsd),feature extraction,yale face database,fuzzy k-nearest neighbor (fknn),nonlinear feature extraction method,feret face database,discriminant analysis,euclidean distance,kernel,scattering matrix,kernel method,face,k nearest neighbor,feature space,databases
AI 理解论文
溯源树
样例
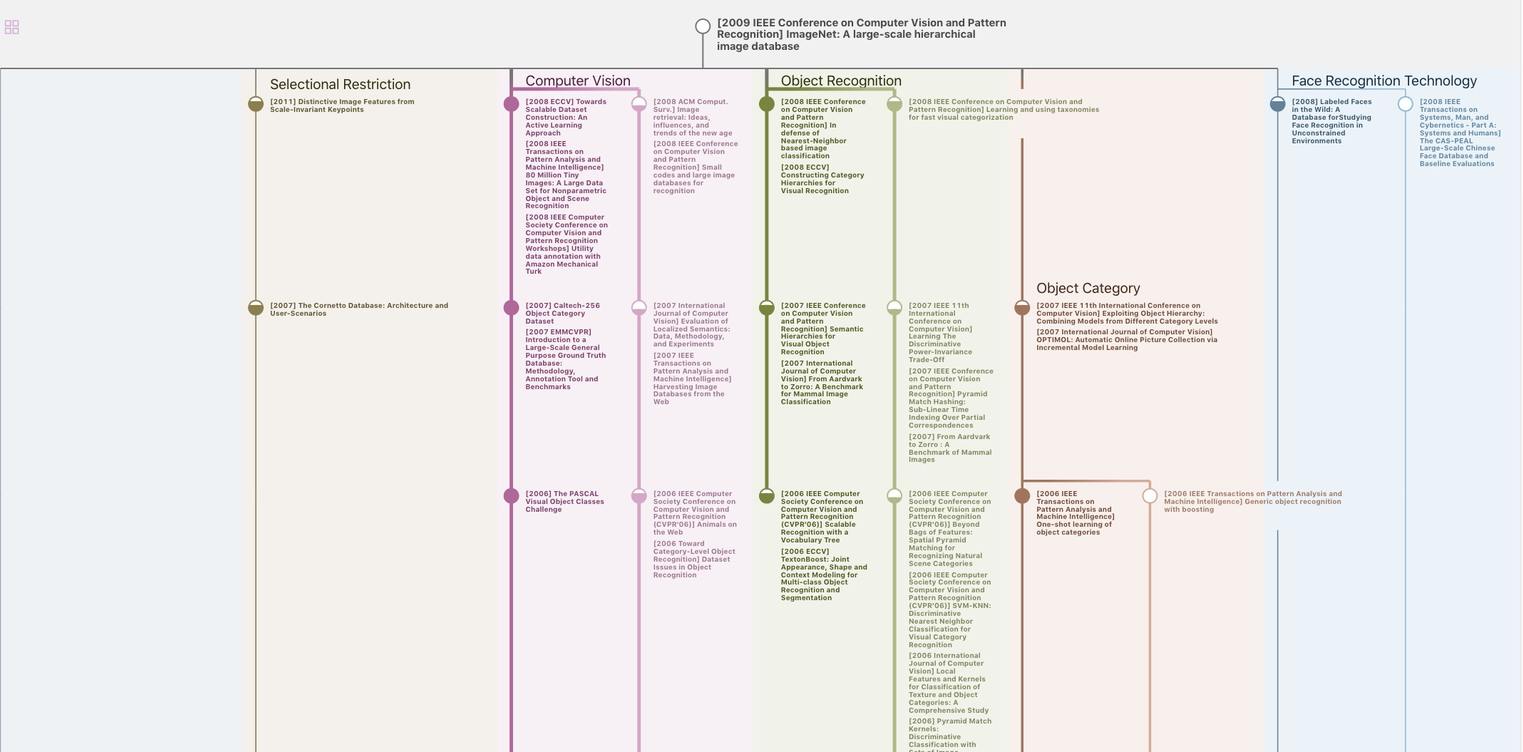
生成溯源树,研究论文发展脉络
Chat Paper
正在生成论文摘要