Robust head pose estimation using supervised manifold projection
ICIP(2012)
摘要
Head poses can be automatically estimated using manifold learning algorithms, with the assumption that with pose being the only variable, the facial images should lie in a smooth and low-dimensional manifold. However, this estimation approach is challenging due to other appearance variations related to identity, head location in image, background, facial expression, and illumination. This problem may be alleviated by incorporating the pose angle information of training samples into the manifold learning process. In this paper, we propose a supervised neighborhood-based linear feature transformation algorithm, which is a variant of Fisher Discriminant Analysis (FDA), to constrain the projection computation of manifold learning. The experimental results show that our algorithm improves the accuracy and robustness of head pose estimation.
更多查看译文
关键词
supervised neighborhood-based fda,head pose estimation,statistical analysis,learning (artificial intelligence),fisher discriminant analysis,pose estimation,manifold learning algorithm,appearance variation,supervised neighborhood-based linear feature transformation algorithm,supervised manifold projection,pose angle information,manifold learning,projection computation,learning artificial intelligence
AI 理解论文
溯源树
样例
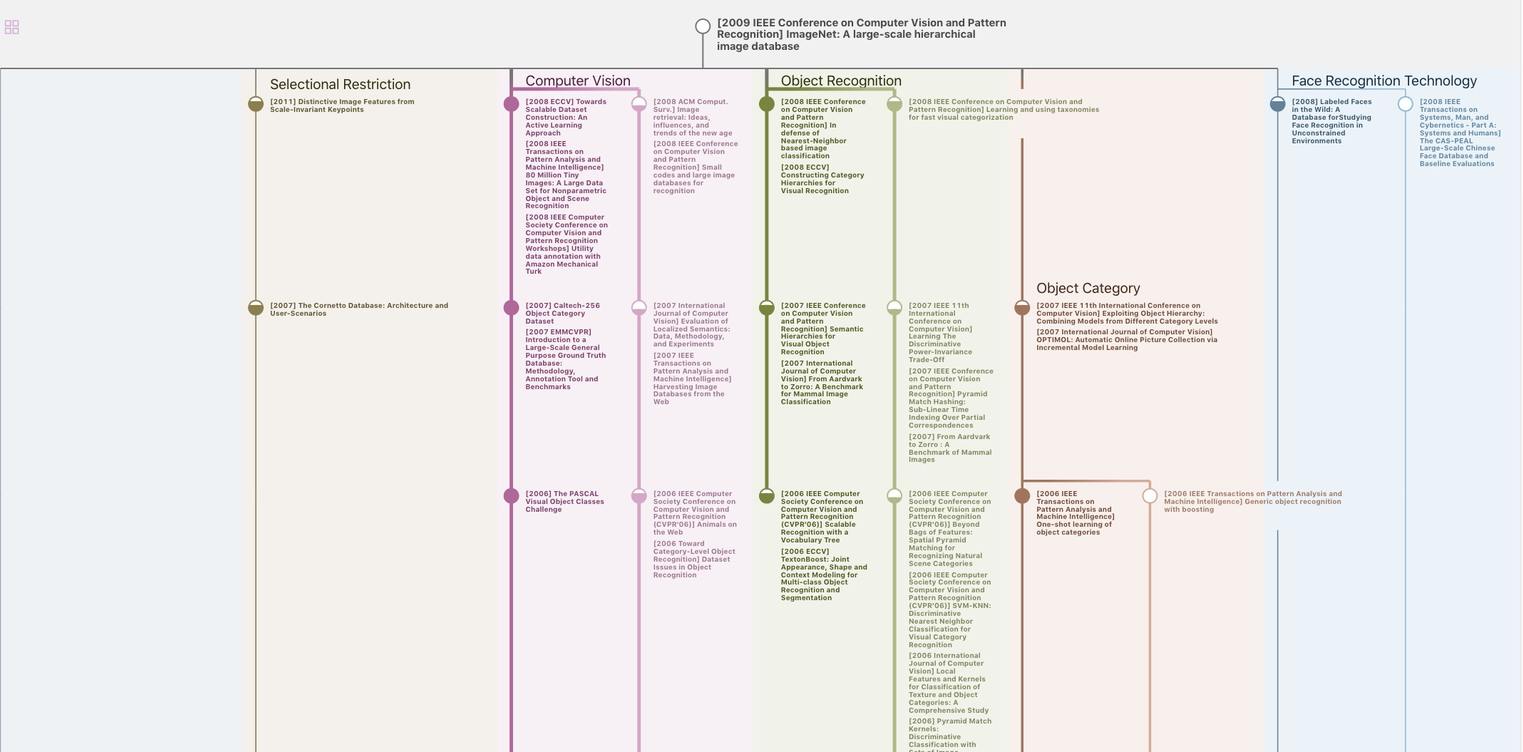
生成溯源树,研究论文发展脉络
Chat Paper
正在生成论文摘要