Imbalanced Learning Ensembles for Defect Detection in X-Ray Images.
IEA/AIE'13: Proceedings of the 26th International Conference on Industrial, Engineering and Other Applications of Applied Intelligent Systems(2013)
摘要
This paper describes the process of detection of defects in metallic pieces through the analysis of X-ray images. The images used in this work are highly variable (several different pieces, different views, variability introduced by the inspection process such as positioning the piece). Because of this variability, the sliding window technique has been used, an approach based on data mining. Experiments have been carried out with various window sizes, several feature selection algorithms and different classification algorithms, with a special focus on learning unbalanced data sets. The results show that Bagging achieved significantly better results than decision trees by themselves or combined with SMOTE or Undersampling.
更多查看译文
关键词
Non Destructive testing, ensemble learning, X-ray, Bagging, Undersampling, SMOTE
AI 理解论文
溯源树
样例
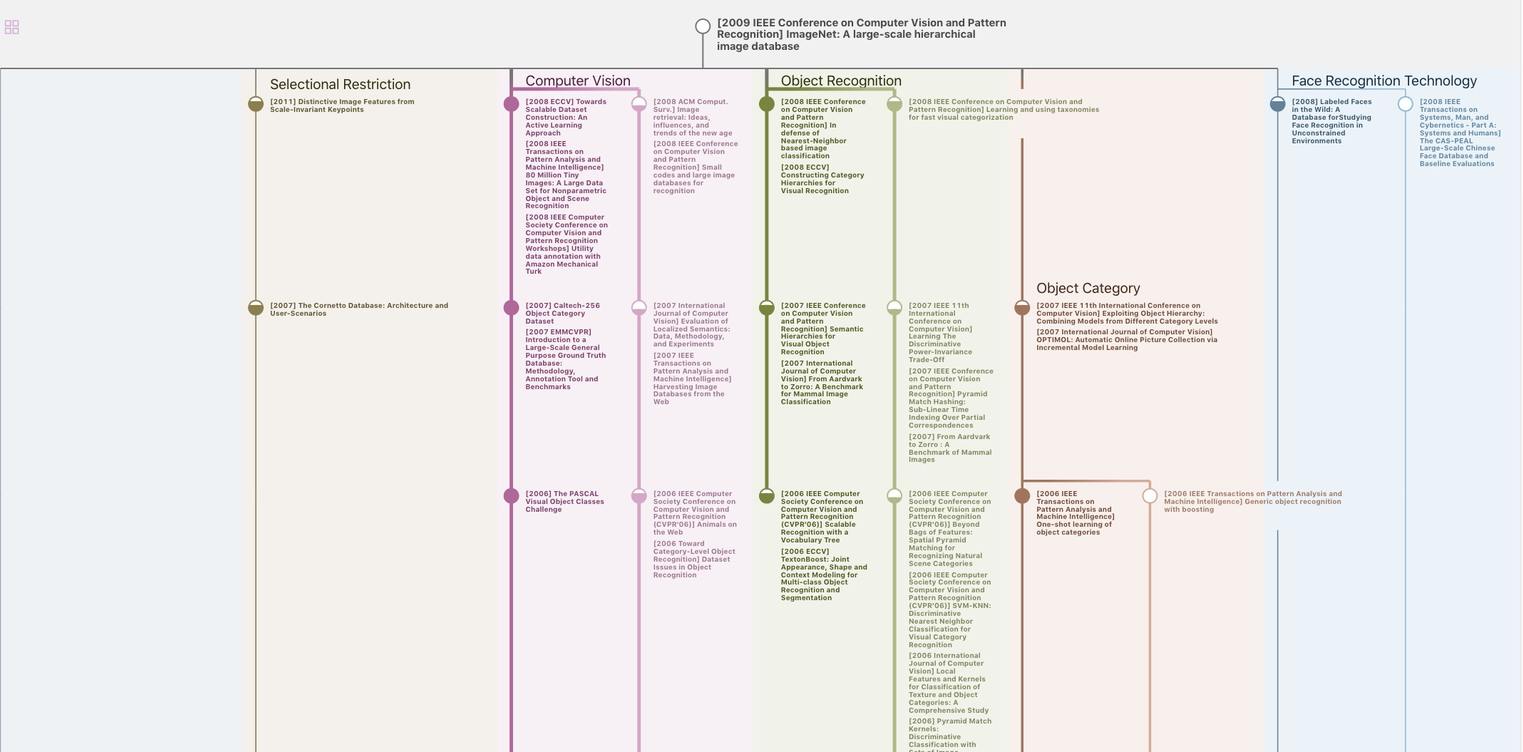
生成溯源树,研究论文发展脉络
Chat Paper
正在生成论文摘要