STPCA: Sparse tensor Principal Component Analysis for feature extraction
ICPR(2012)
摘要
Due to the fact that many objects in the real world can be naturally represented as tensors, tensor subspace analysis has become a hot research area in pattern recognition and computer vision. However, existing tensor subspace analysis methods cannot provide an intuitionistic nor semantic interpretation for the projection matrices. In this paper, we propose Sparse Tensor Principal Component Analysis (STPCA), which transforms the eigen-decomposition problem to a series of regression problems. Since its projection matrices are sparse, STPCA can also address the occlusion problem. Experiment on Georgia tech database and AR database showed that the proposed method outperforms the Multilinear Principal Component Analysis (MPCA) in terms of accuracy and robustness.
更多查看译文
关键词
stpca,pattern recognition,semantic interpretation,tensor subspace analysis methods,eigen-decomposition problem,computer graphics,matrix algebra,multilinear principal component analysis,occlusion problem,projection matrices,feature extraction,sparse tensor principal component analysis,mpca,georgia tech database,intuitionistic interpretation,regression problems,computer vision,ar database,principal component analysis,tensors
AI 理解论文
溯源树
样例
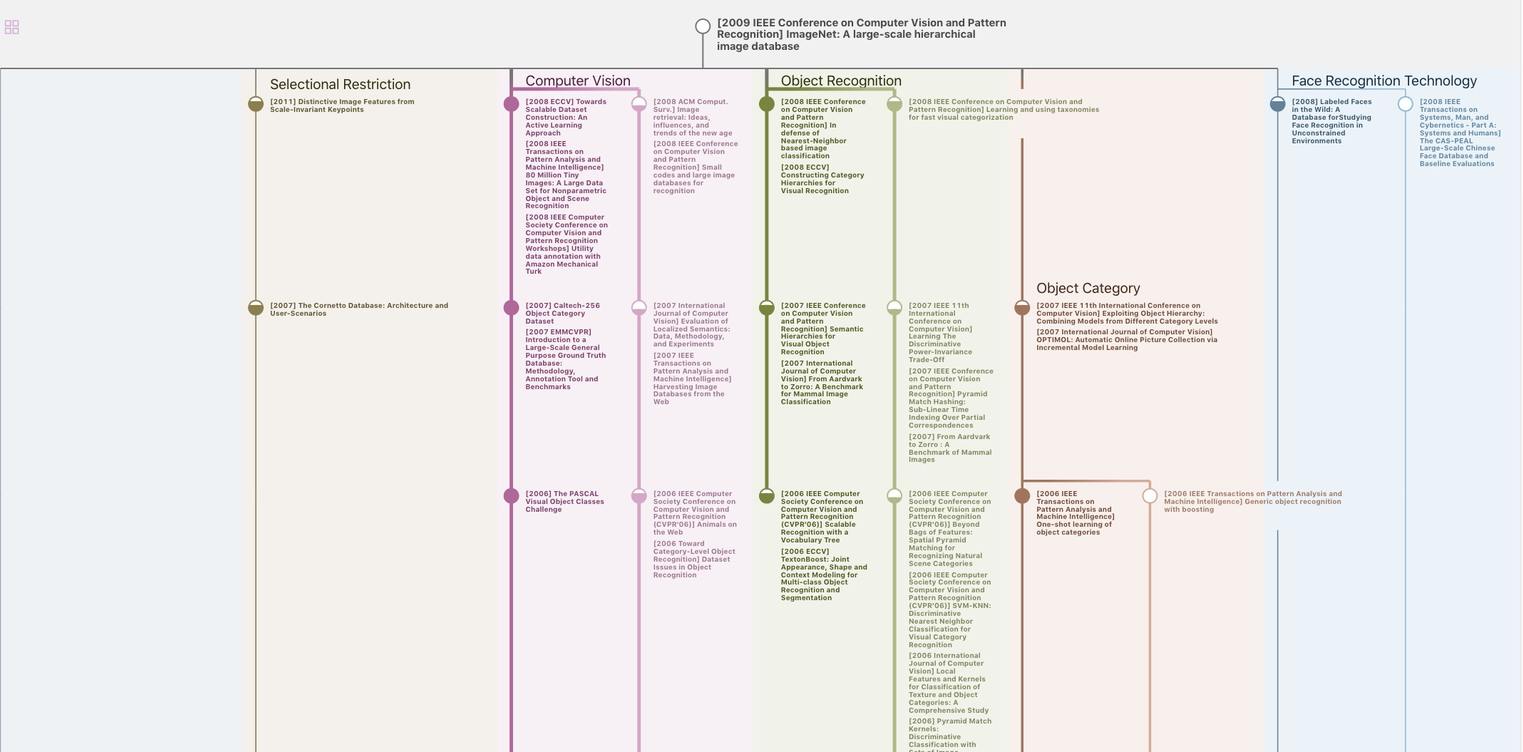
生成溯源树,研究论文发展脉络
Chat Paper
正在生成论文摘要