A Combined Monte-Carlo Localization And Tracking Algorithm For Robocup
2006 IEEE/RSJ INTERNATIONAL CONFERENCE ON INTELLIGENT ROBOTS AND SYSTEMS, VOLS 1-12(2006)
摘要
Self-localization is a major research task in mobile robotics for several years. Efficient self-localization methods have been developed, among which probabilistic Monte-Carlo localization (MCL) is one of the most popular. It enables robots to localize themselves in real-time and to recover from localization errors. However, even those versions of MCL using an adaptive number of samples need at least a minimum in the order of 100 samples to compute an acceptable position estimation. This paper presents a novel approach to MCL based on images from an omnidirectional camera system. The approach uses an adaptive number of samples that drops down to a single sample if the pose estimation is sufficiently accurate. We show that the method enters this efficient tracking mode after a few cycles and remains there using only a single sample for more than 90% of the cycles. Nevertheless, it is still able to cope with the kidnapped robot problem.
更多查看译文
关键词
monte carlo localization,mobile robot,mobile robots,pose estimation,monte carlo methods,path planning
AI 理解论文
溯源树
样例
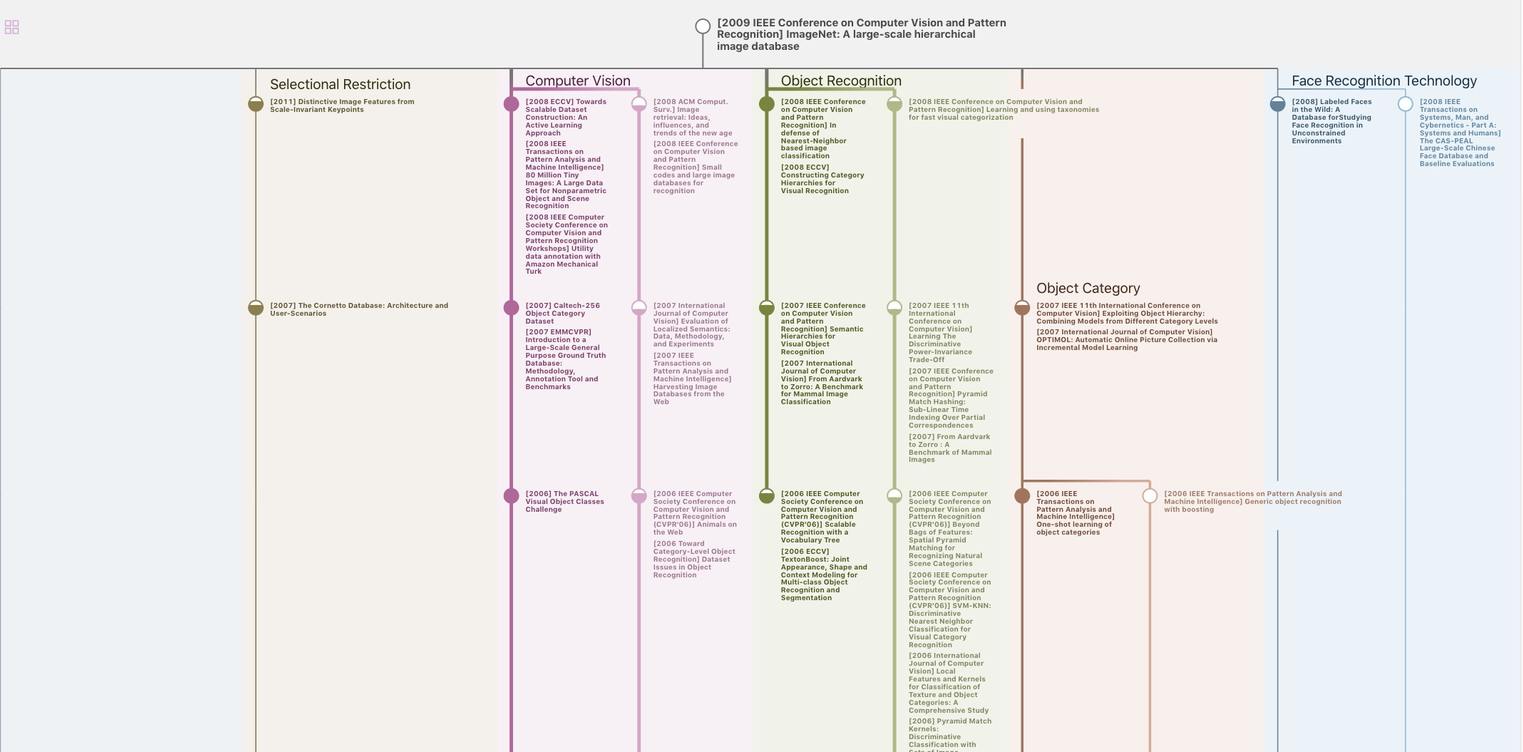
生成溯源树,研究论文发展脉络
Chat Paper
正在生成论文摘要