Unsupervised Anomaly-Based Malware Detection Using Hardware Features.
RAID(2014)
摘要
Recent works have shown promise in using microarchitectural execution patterns to detect malware programs. These detectors belong to a class of detectors known as signature-based detectors as they catch malware by comparing a program's execution pattern (signature) to execution patterns of known malware programs. In this work, we propose a new class of detectors - anomaly-based hardware malware detectors - that do not require signatures for malware detection, and thus can catch a wider range of malware including potentially novel ones. We use unsupervised machine learning to build profiles of normal program execution based on data from performance counters, and use these profiles to detect significant deviations in program behavior that occur as a result of malware exploitation. We show that real-world exploitation of popular programs such as IE and Adobe PDF Reader on a Windows/x86 platform can be detected with nearly perfect certainty. We also examine the limits and challenges in implementing this approach in face of a sophisticated adversary attempting to evade anomaly-based detection. The proposed detector is complementary to previously proposed signature-based detectors and can be used together to improve security.
更多查看译文
关键词
computer science
AI 理解论文
溯源树
样例
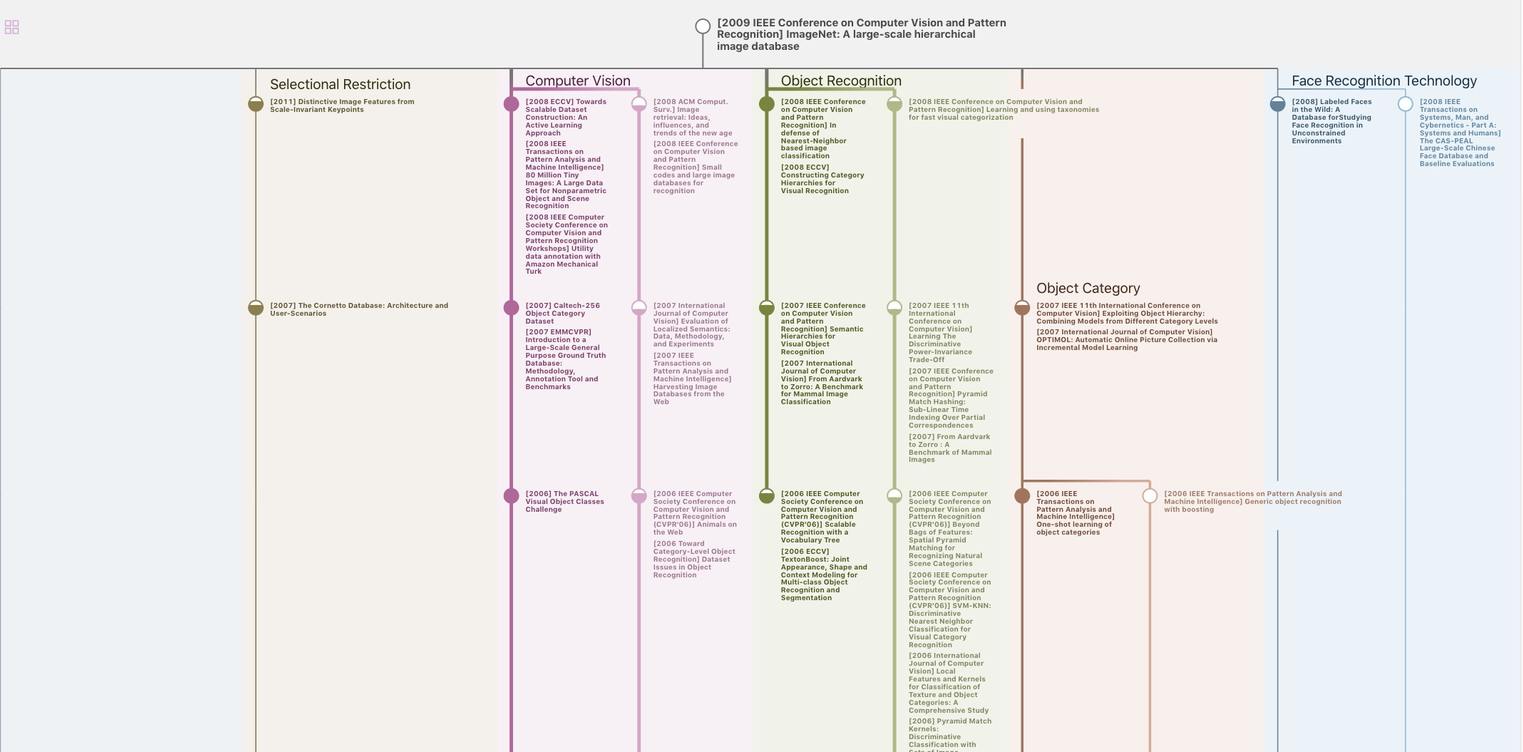
生成溯源树,研究论文发展脉络
Chat Paper
正在生成论文摘要