Edge-count probabilities for the identification of local protein communities and their organization.
PROTEINS-STRUCTURE FUNCTION AND BIOINFORMATICS(2006)
摘要
We present a computational approach based on a local search strategy that discovers sets of proteins that preferentially interact with each other. Such sets are referred to as protein communities and are likely to represent functional modules. Preferential interaction between module members is quantified via an analytical framework based on a network null model known as the random graph with given expected degrees. Based on this framework, the concept of local protein community is generalized to that of community of communities. Protein communities and higher-level structures are extracted from two yeast protein interaction data sets and a network of published interactions between human proteins. The high level structures obtained with the human network correspond to broad biological concepts such as signal transduction, regulation of gene expression, and intercellular communication. Many of the obtained human communities are enriched, in a statistically significant way, for proteins having no clear orthologs in lower organisms. This indicates that the extracted modules are quite coherent in terms of function.
更多查看译文
关键词
random graph,random graph with given expected degrees,degree sequence,protein interaction network
AI 理解论文
溯源树
样例
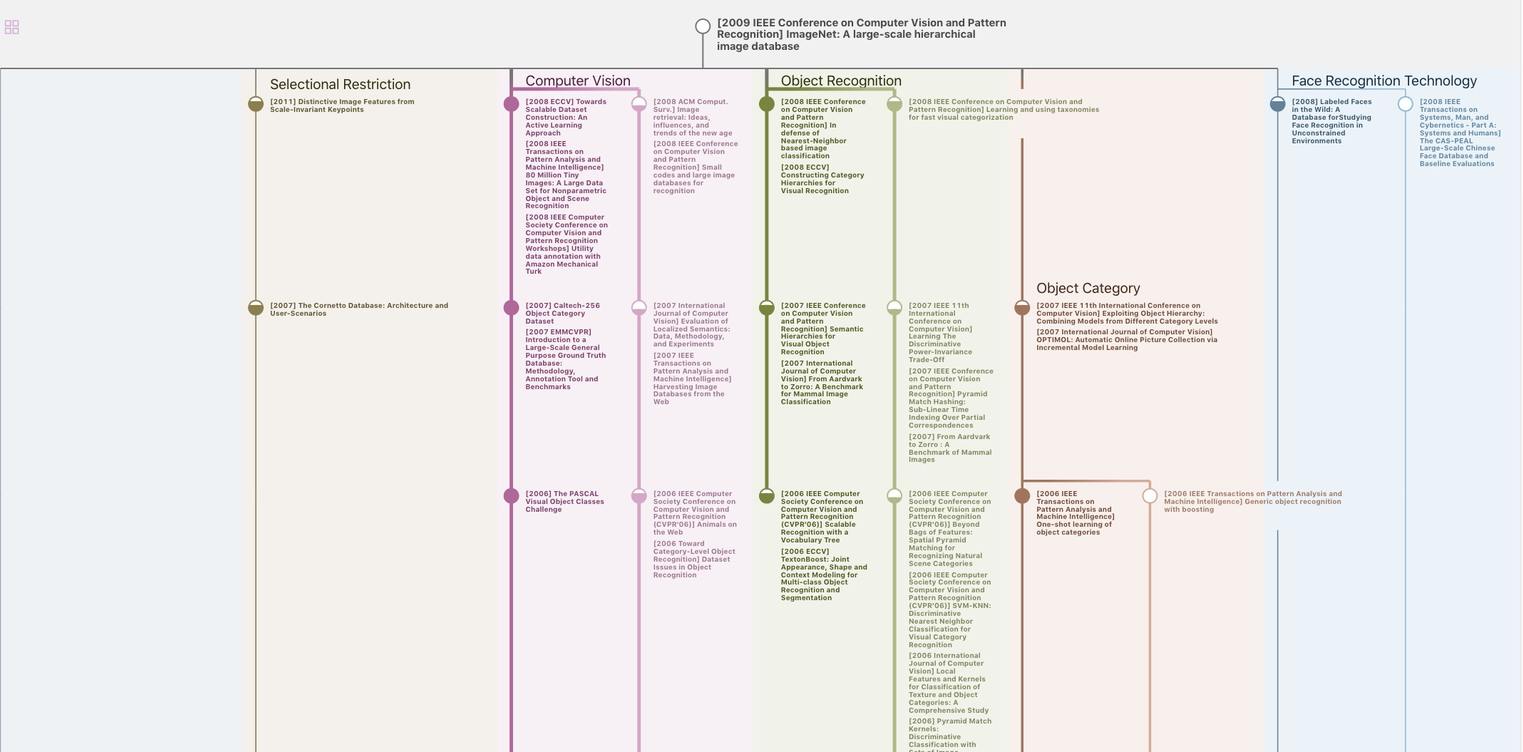
生成溯源树,研究论文发展脉络
Chat Paper
正在生成论文摘要