Towards Codebook-Free: Scalable Cascaded Hashing for Mobile Image Search
IEEE Transactions on Multimedia(2014)
摘要
State-of-the-art image retrieval algorithms using local invariant features mostly rely on a large visual codebook to accelerate the feature quantization and matching. This codebook typically contains millions of visual words, which not only demands for considerable resources to train offline but also consumes large amount of memory at the online retrieval stage. This is hardly affordable in resource limited scenarios such as mobile image search applications. To address this issue, we propose a codebook-free algorithm for large scale mobile image search. In our method, we first employ a novel scalable cascaded hashing scheme to ensure the recall rate of local feature matching. Afterwards, we enhance the matching precision by an efficient verification with the binary signatures of these local features. Consequently, our method achieves fast and accurate feature matching free of a huge visual codebook. Moreover, the quantization and binarizing functions in the proposed scheme are independent of small collections of training images and generalize well for diverse image datasets. Evaluated on two public datasets with a million distractor images, the proposed algorithm demonstrates competitive retrieval accuracy and scalability against four recent retrieval methods in literature.
更多查看译文
关键词
Visualization,Quantization (signal),Principal component analysis,Training,Mobile communication,Feature extraction,Accuracy
AI 理解论文
溯源树
样例
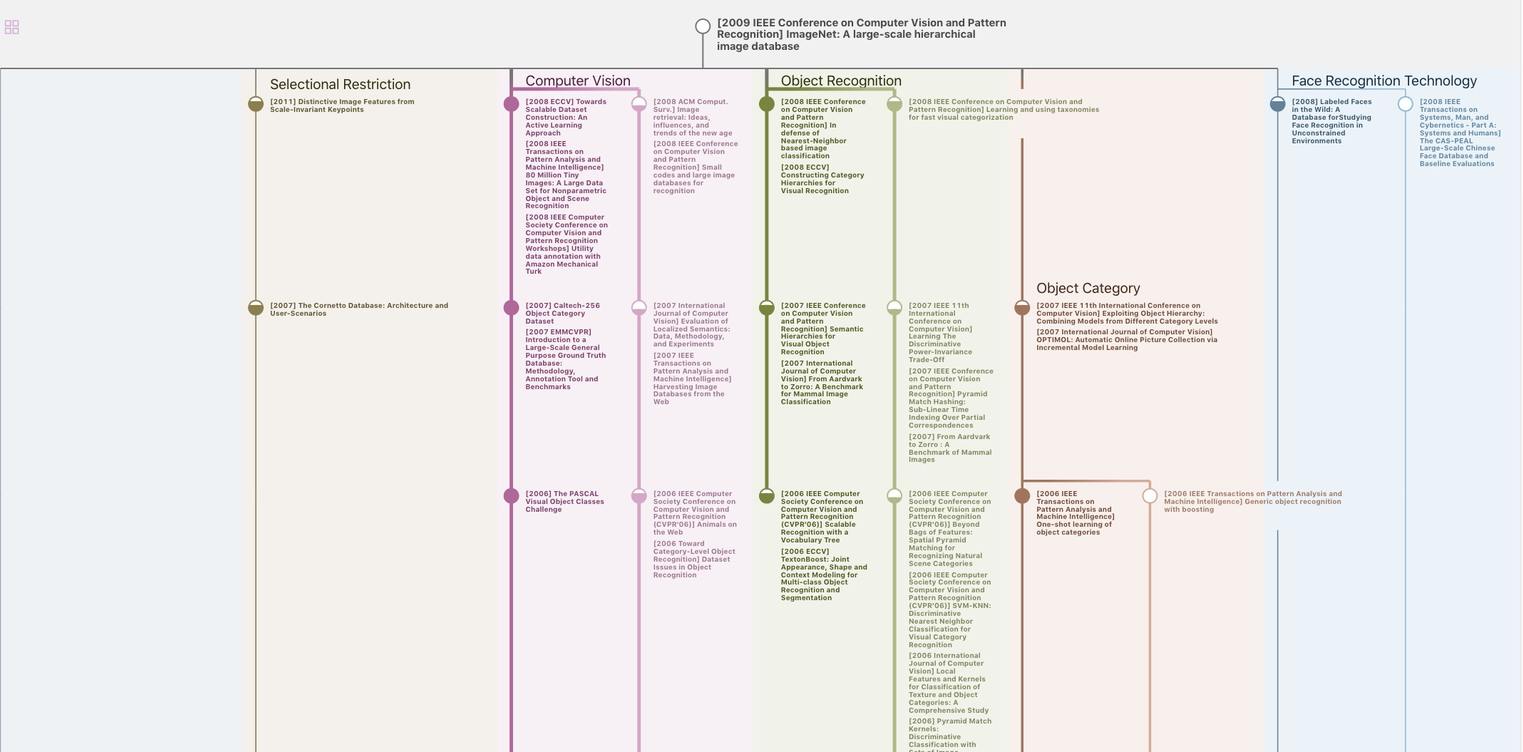
生成溯源树,研究论文发展脉络
Chat Paper
正在生成论文摘要