The Sensitivity of the Number of Clusters in a Gaussian Mixture Model to Prior Distributions
Mathematics in Computer Science(2013)
摘要
One of the main advantages of Bayesian approaches is that they offer principled methods of inference in models of varying dimensionality and of models of infinite dimensionality. What is less widely appreciated is how the model inference is sensitive to prior distributions and therefore how priors should be set for real problems. In this paper prior sensitivity is considered with respect to the problem of inference in Gaussian mixture models. Two distinct Bayesian approaches have been proposed. The first is to use Bayesian model selection based upon the marginal likelihood; the second is to use an infinite mixture model which ‘side steps’ model selection. Explanations for the prior sensitivity are given in order to give practitioners guidance in setting prior distributions. In particular the use of conditionally conjugate prior distributions instead of purely conjugate prior distributions are advocated as a method for investigating prior sensitivity of the mean and variance individually.
更多查看译文
关键词
Density estimation,Learning and adaptive systems,Randomized algorithms,Approximation algorithms
AI 理解论文
溯源树
样例
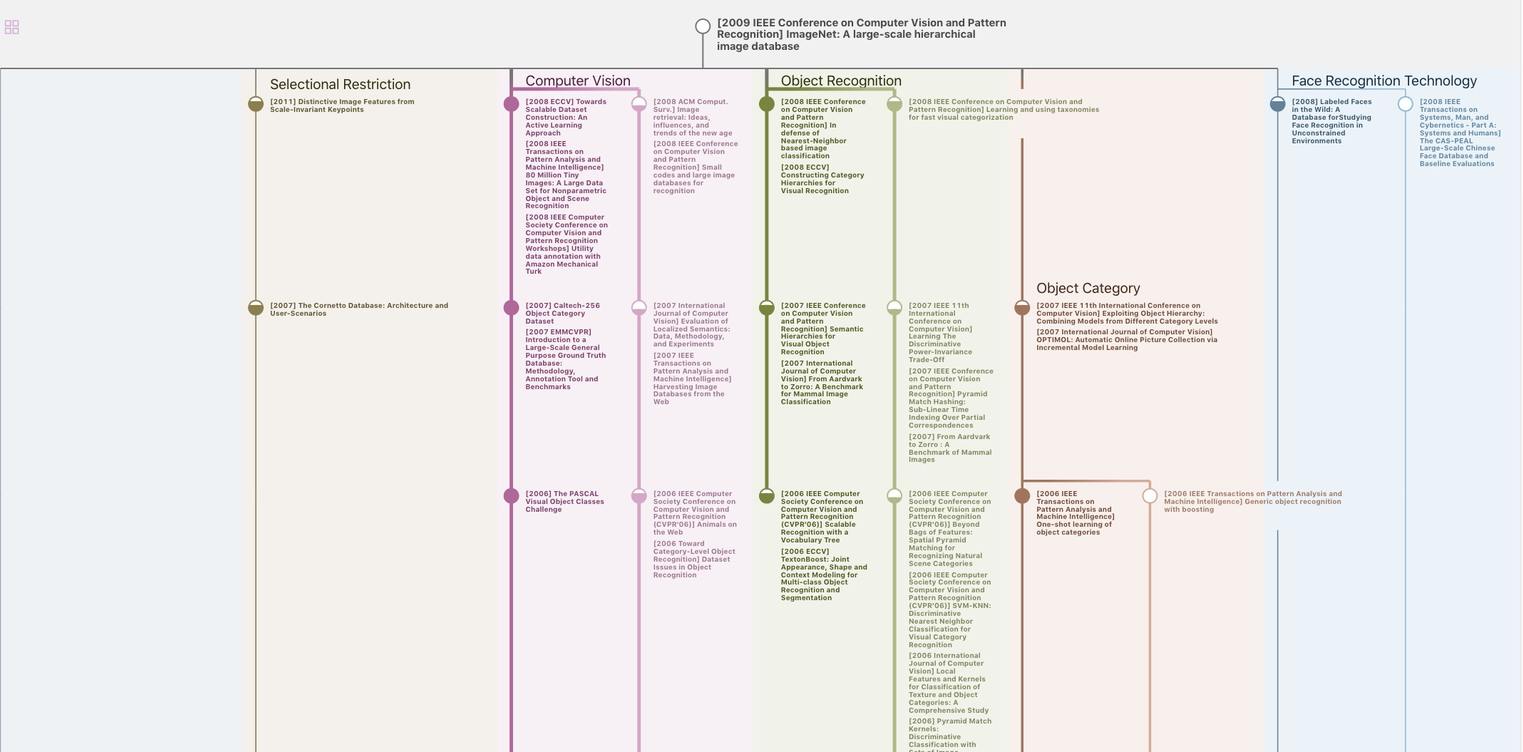
生成溯源树,研究论文发展脉络
Chat Paper
正在生成论文摘要