Neural activity tracking using spatial compressive particle filtering
ICASSP(2012)
摘要
We investigate and demonstrate the sparsity of electroencephalography (EEG) signals in the spatial domain by incorporating grid spacing in the area of the head enclosing the brain volume. We exploit this spatial sparsity and propose a new approach for tracking neural activity that is based on compressive particle filtering. Our approach results in reducing the number of EEG channels required to be stored and processed for neural tracking using particle filtering. Simulations using both synthetic and real EEG signals illustrate that the proposed algorithm has tracking performance comparable to existing methods while using only a reduced set of EEG channels.
更多查看译文
关键词
electroencephalography signals,spatial sparsity,electroencephalography,spatial compressive particle filtering,medical signal processing,compressive sensing,dipole model,eeg signals,filtering theory,eeg,neural activity tracking,grid spacing,multiple particle filter,compressed sensing,mathematical model,vectors
AI 理解论文
溯源树
样例
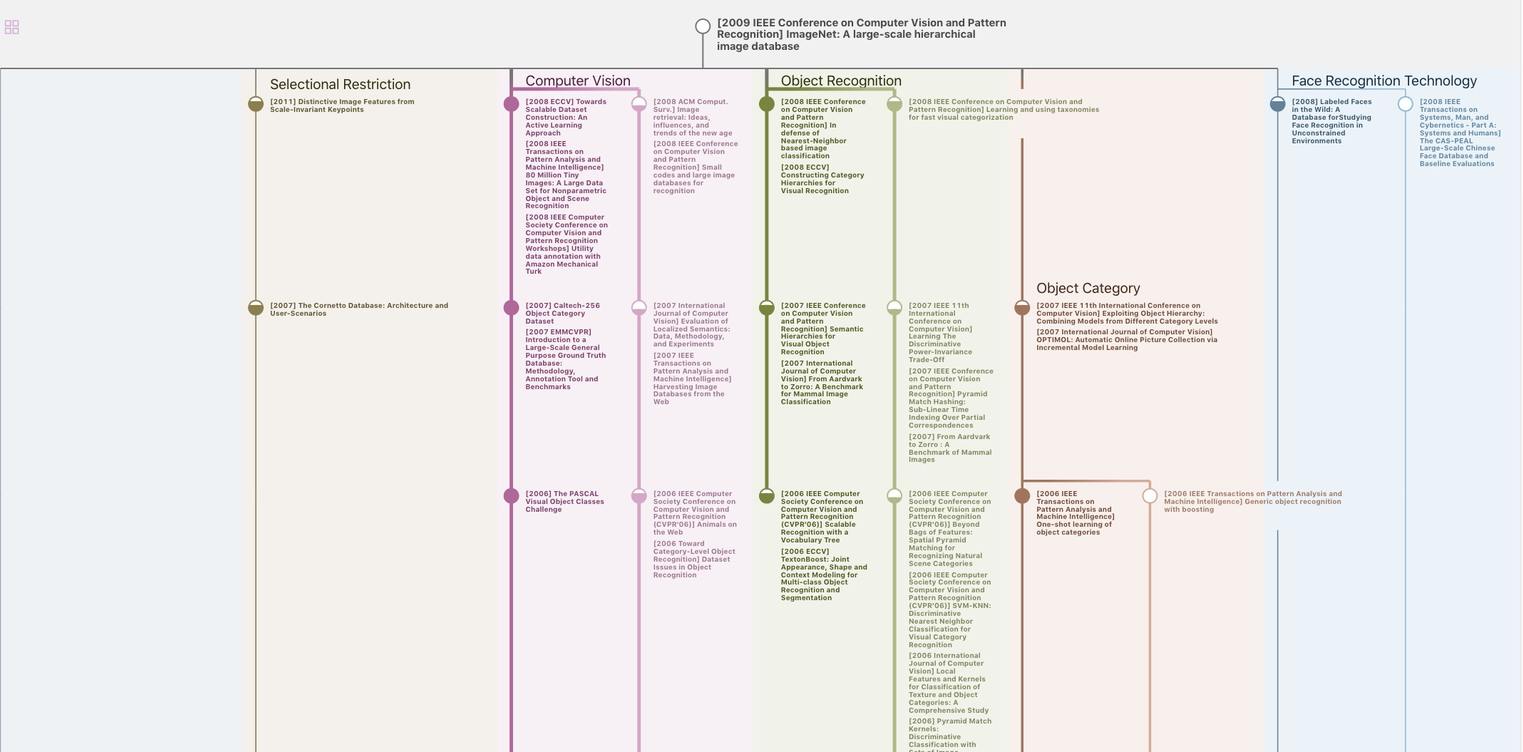
生成溯源树,研究论文发展脉络
Chat Paper
正在生成论文摘要