Active query sensing: Suggesting the best query view for mobile visual search
TOMCCAP(2012)
摘要
While much exciting progress is being made in mobile visual search, one important question has been left unexplored in all current systems. When searching objects or scenes in the 3D world, which viewing angle is more likely to be successful? More particularly, if the first query fails to find the right target, how should the user control the mobile camera to form the second query? In this article, we propose a novel Active Query Sensing system for mobile location search, which actively suggests the best subsequent query view to recognize the physical location in the mobile environment. The proposed system includes two unique components: (1) an offline process for analyzing the saliencies of different views associated with each geographical location, which predicts the location search precisions of individual views by modeling their self-retrieval score distributions. (2) an online process for estimating the view of an unseen query, and suggesting the best subsequent view change. Specifically, the optimal viewing angle change for the next query can be formulated as an online information theoretic approach. Using a scalable visual search system implemented over a NYC street view dataset (0.3 million images), we show a performance gain by reducing the failure rate of mobile location search to only 12% after the second query. We have also implemented an end-to-end functional system, including user interfaces on iPhones, client-server communication, and a remote search server. This work may open up an exciting new direction for developing interactive mobile media applications through the innovative exploitation of active sensing and query formulation.
更多查看译文
关键词
unseen query,mobile location search,mobile environment,interactive mobile media application,query formulation,next query,mobile visual search,subsequent query view,location search precision,active query,mobile camera
AI 理解论文
溯源树
样例
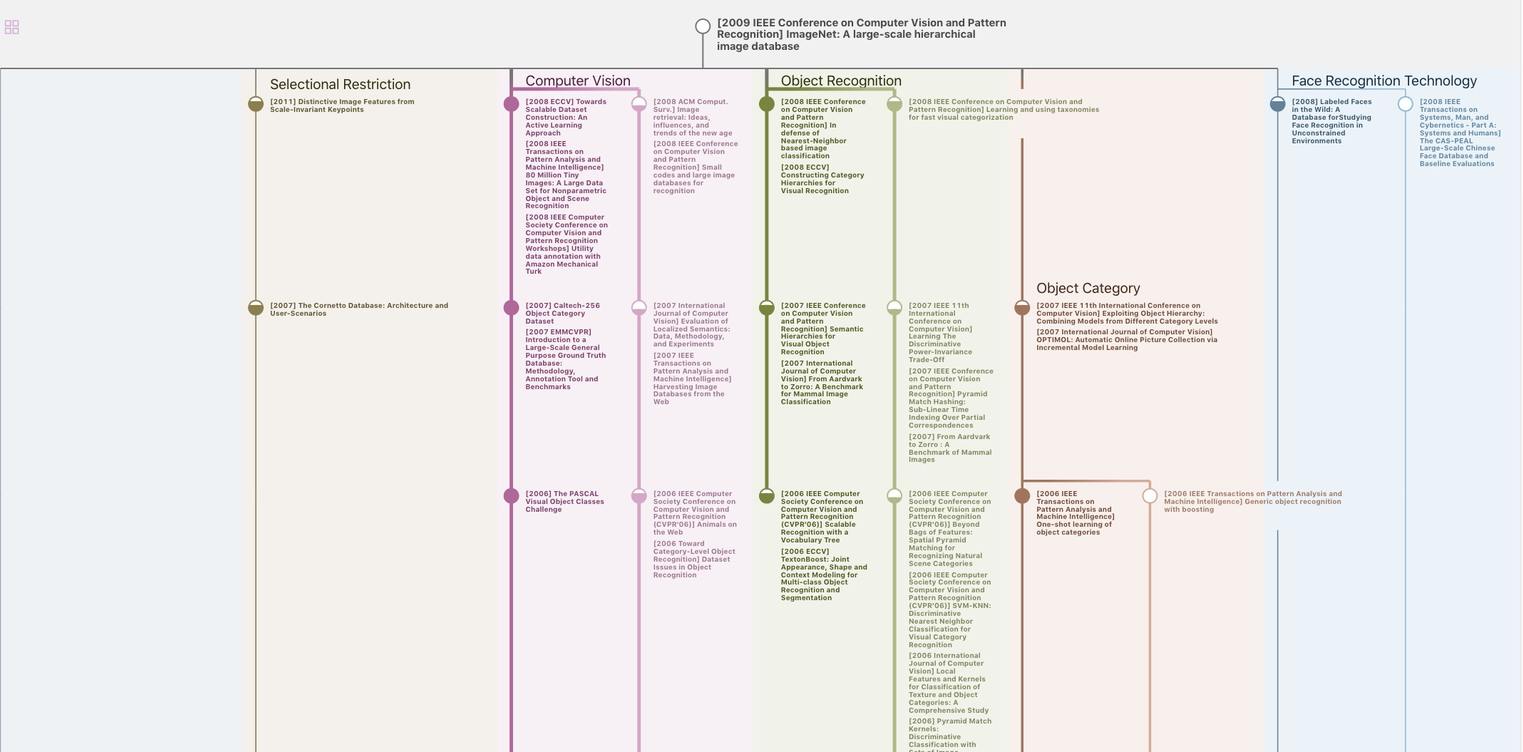
生成溯源树,研究论文发展脉络
Chat Paper
正在生成论文摘要