Bilinear Generalized Approximate Message Passing-Part I: Derivation
IEEE TRANSACTIONS ON SIGNAL PROCESSING(2014)
摘要
In this paper, we extend the generalized approximate message passing (G-AMP) approach, originally proposed for high-dimensional generalized-linear regression in the context of compressive sensing, to the generalized-bilinear case, which enables its application to matrix completion, robust PCA, dictionary learning, and related matrix-factorization problems. Here, in Part I of a two-part paper, we derive our Bilinear G-AMP (BiG-AMP) algorithm as an approximation of the sum-product belief propagation algorithm in the high-dimensional limit, where central-limit theorem arguments and Taylor-series approximations apply, and under the assumption of statistically independent matrix entries with known priors. In addition, we propose an adaptive damping mechanism that aids convergence under finite problem sizes, an expectation-maximization (EM)-based method to automatically tune the parameters of the assumed priors, and two rank-selection strategies. In Part II of the paper, we will discuss the specializations of EM-BiG-AMP to the problems of matrix completion, robust PCA, and dictionary learning, and we will present the results of an extensive empirical study comparing EM-BiG-AMP to state-of-the-art algorithms on each problem.
更多查看译文
关键词
Approximate message passing, belief propagation, bilinear estimation, matrix completion, dictionary learning, robust principal components analysis, matrix factorization
AI 理解论文
溯源树
样例
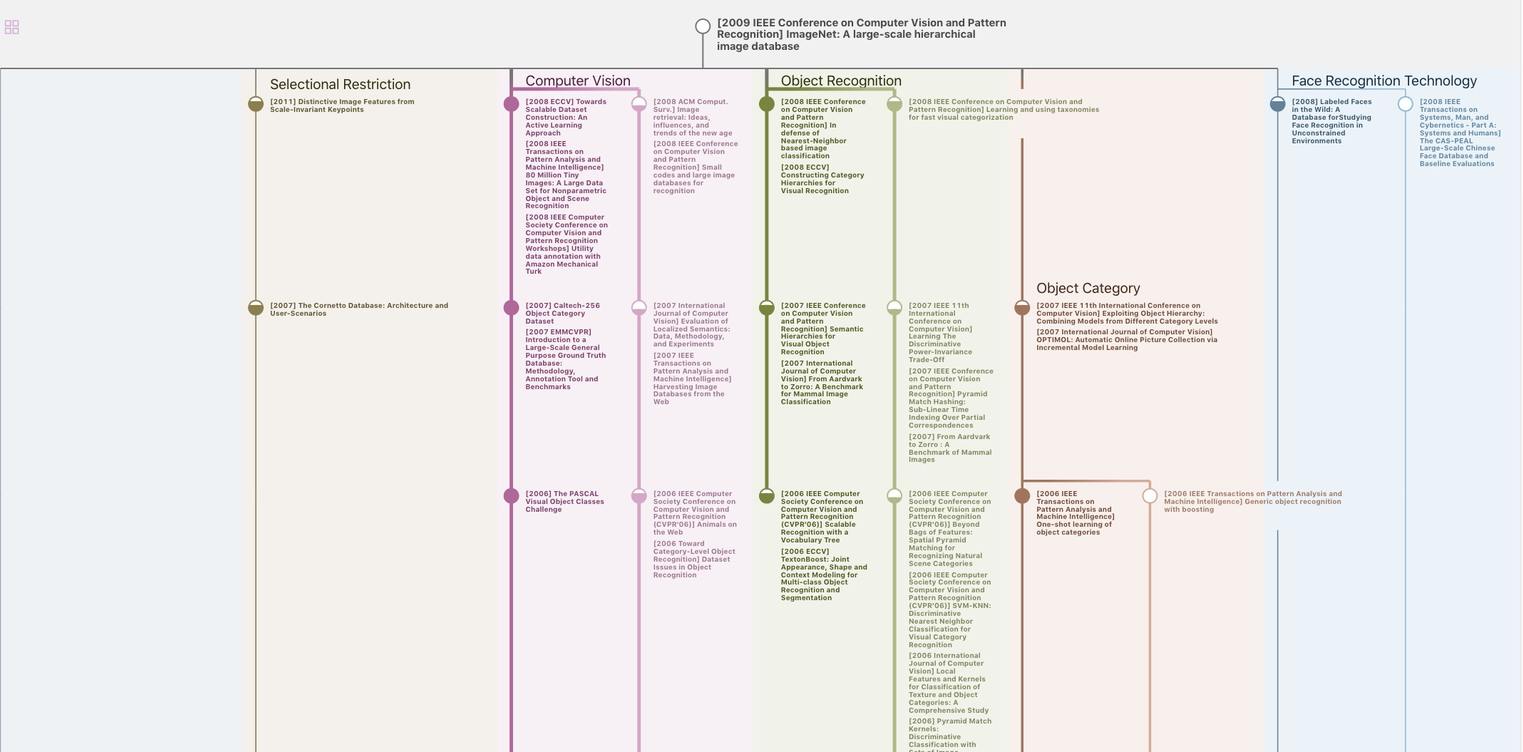
生成溯源树,研究论文发展脉络
Chat Paper
正在生成论文摘要