TH-D-213A-02: An Improved Iterative Thresholding Approach for 3D PET Tumor Delineation: Phantom Study
MEDICAL PHYSICS(2009)
摘要
Purpose: To develop an improved iterative thresholding method (IITM) for 3D PET tumor delineation, motivated by the iterative thresholding method (ITM). Method and Materials: The IITM method is an iterative thresholding method that selects the threshold level to segment a tumor based on calibrated source‐to‐background threshold‐volume (SBTV) curves. From an initial tumor volume V 0, the source‐to‐background (S/B) ratio R 0 is calculated as the ratio of the average intensity of V 0 and the average intensity of regions of interest in the background. A calibrated SBTV curve corresponding to R 0 is generated by interpolation using those pre‐defined calibrated SBTV curves. This SBTV curve is used for finding a threshold level T 1. The threshold level T 1 is applied to the image and a new estimation of volume V 1 is obtained. This iterative process of updating the SBTV curve, the threshold level and the volume is repeated until the threshold values T i and T i‐1 are not significantly different. In comparison, the ITM method calculates the source as the average intensity in a small neighborhood of the voxel with the maximum intensity and the S/B ratio is fixed during its iterative process. A phantom study using six spherical tumor phantoms (0.5 – 20 mL) with S/B ratio ranging from 16 to 0.5 is used to test these two methods. Also a 3D Gaussian function was used to generate heterogeneous pattern in the phantoms. Results: The IITM method yielded better or equivalent delineation accuracy than the ITM method on all images, particularly at low S/B ratio of 3 for heterogeneous targets. Conclusion: By measuring the source intensity in the entire tumor region and iteratively updating the SBTV curve in addition to the volume and threshold level, the IITM method shows some advantages over the ITM method, particularly for heterogeneous targets.
更多查看译文
关键词
3d pet tumor delineation,improved iterative thresholding approach
AI 理解论文
溯源树
样例
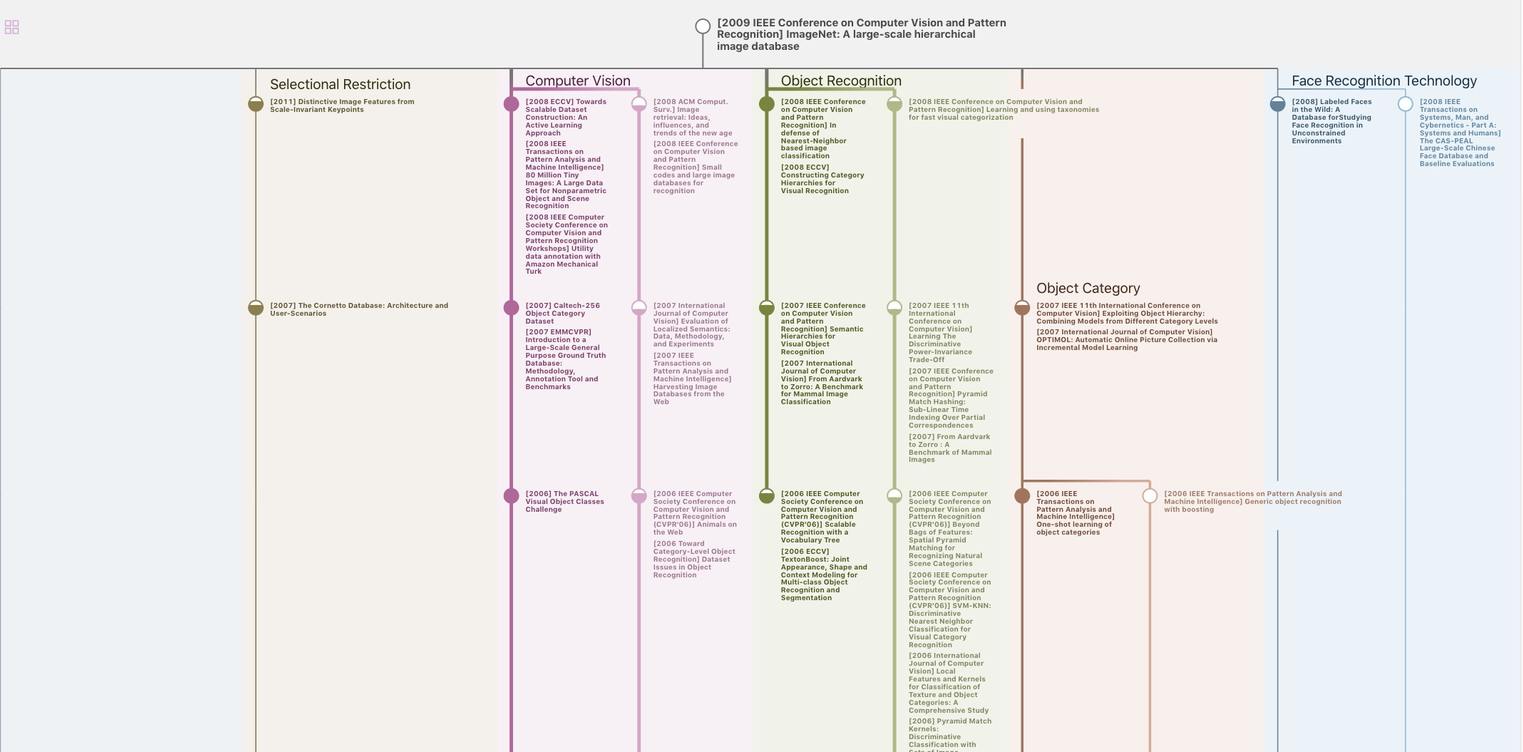
生成溯源树,研究论文发展脉络
Chat Paper
正在生成论文摘要