Combining Different Methods and Numbers of Weak Decision Trees
Pattern Analysis & Applications(2002)
摘要
: Several ways of manipulating a training set have shown that weakened classifier combination can improve prediction accuracy. In the present paper, we focus on learning set sampling (Breiman’s Bagging) and random feature subset selections (Ho’s Random Subspaces). We present a combination scheme labelled ‘Bagfs’, in which new learning sets are generated on the basis of both bootstrap replicates and random subspaces. The performances of the three methods (Bagging, Random Subspaces and Bagfs) are compared to the standard Adaboost algorithm. All four methods are assessed by means of a decision-tree inducer (C4.5). In addition, we also study whether the number and the way in which they are created has a significant influence on the performance of their combination. To answer these two questions, we undertook the application of the McNemar test of significance and the Kappa degree-of-agreement. The results, obtained on 23 conventional databases, show that on average, Bagfs exhibits the best agreement between prediction and supervision.
更多查看译文
关键词
Key words: Bagging,Boosting,Decision trees,Ensemble learning,Random subspaces
AI 理解论文
溯源树
样例
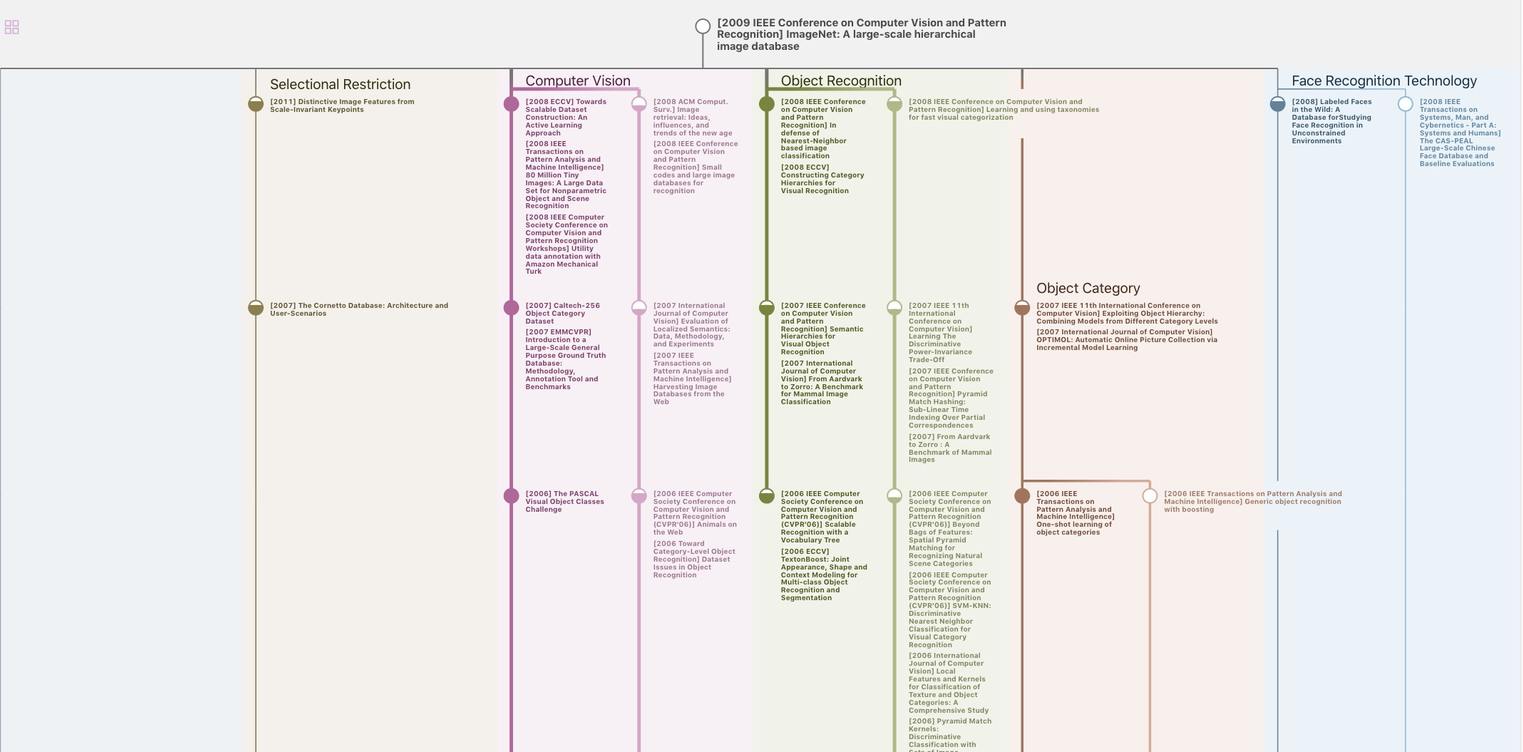
生成溯源树,研究论文发展脉络
Chat Paper
正在生成论文摘要