Semi-supervised learning, large graphs, betweenness measure, passage times
msra
摘要
This paper describes a novel technique, called D-walks, to tackle semi-supervised classifica- tion problems in large graphs. We introduce here a betweenness measure based on pas- sage times during random walks of bounded lengths in the input graph. The class of un- labeled nodes is predicted by maximizing the betweenness with labeled nodes. This ap- proach can deal with directed or undirected graphs with a linear time complexity with respect to the number of edges, the maxi- mum walk length considered and the number of classes. Preliminary experiments on the CORA database show that D-walks outper- forms NetKit (Macskassy & Provost, 2007) as well as Zhou et al's algorithm (Zhou et al., 2005), both in classification rate and comput- ing time.
更多查看译文
AI 理解论文
溯源树
样例
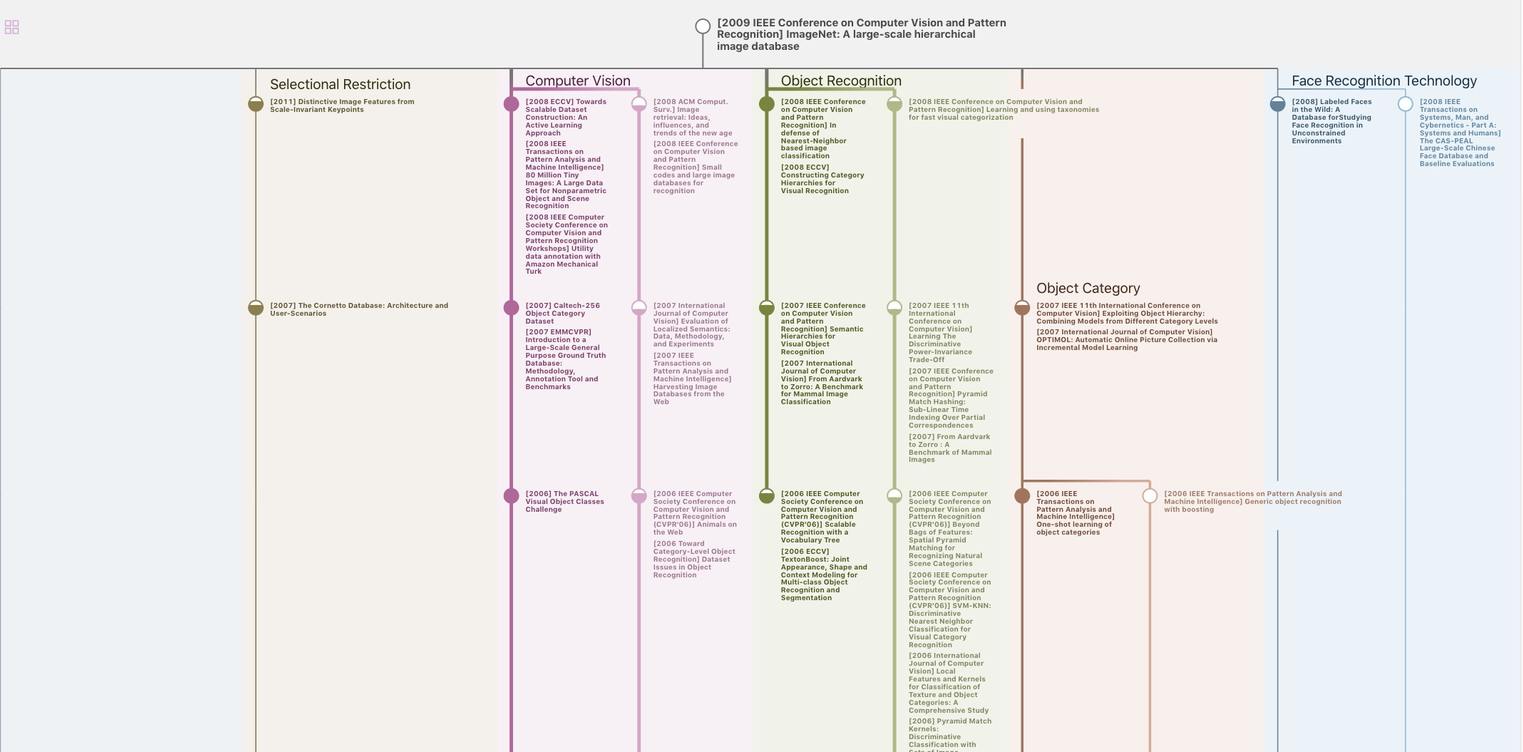
生成溯源树,研究论文发展脉络
Chat Paper
正在生成论文摘要