Mixtures of Conditional Maximum Entropy Models.
ICML(2003)
摘要
Driven by successes in several application areas, maximum entropy modeling has recently gained considerable popularity. We generalize the standard maximum entropy formulation of classification problems to better handle the case where complex data distributions arise from a mixture of simpler underlying (latent) distributions. We develop a theoretical framework for characterizing data as a mixture o] maximum entropy models. We formulate a maximum-likelihood interpretation of the mixture model learning, and derive a generalized EM algorithm to solve the corresponding optimization problem. We present empirical results for a number of data sets showing that modeling the data as a mixture of latent maximum entropy models gives significant improvement over the standard, single component, maximum entropy approach. Keywords: Mixture model, maximum entropy, latent structure, classification.
更多查看译文
关键词
maximum entropy,mixture model,maximum entropy model
AI 理解论文
溯源树
样例
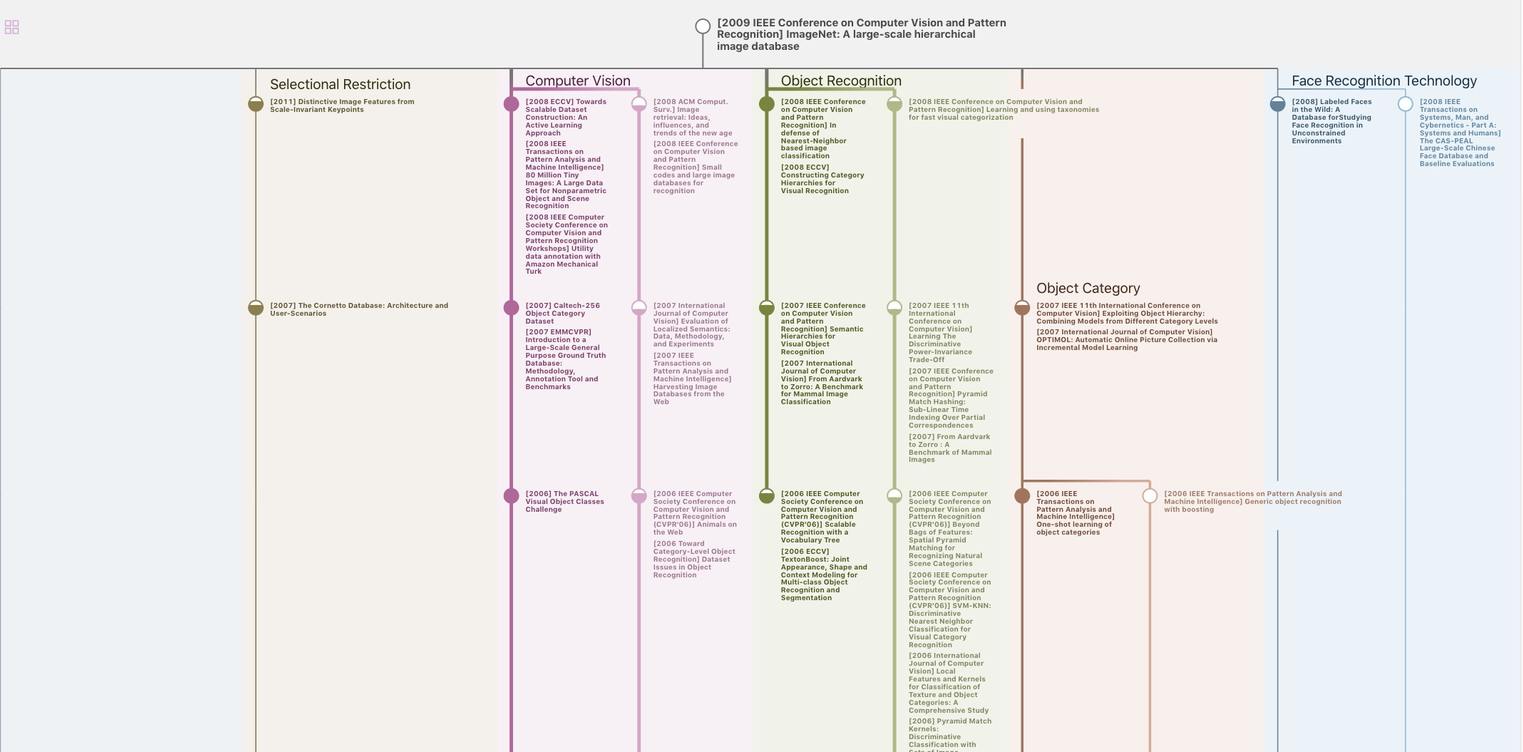
生成溯源树,研究论文发展脉络
Chat Paper
正在生成论文摘要