Story similarity measures for drama management with ttd-mdps
AAMAS(2014)
摘要
In interactive drama, whether for entertainment or training purposes, there is a need to balance the enforcement of authorial intent with player autonomy. A promising approach to this problem is the incorporation of an intelligent Drama Manager (DM) into the simulated environment. The DM can intervene in the story as it progresses in order to (more or less gently) guide the player in an appropriate direction. Framing drama management as the selection of an optimal probabilistic policy in a Targeted Trajectory Distribution Markov Decision Process (TTD-MDP) describing the simulation has been shown to be an effective technique for drama management with a focus on replayability. One of the challenges of drama management is providing a means for the author to express desired story outcomes. In the case of TTD-MDP-based drama management, this is generally understood to involve defining a distance measure over trajectories through the story. While this is a central issue in the practical deployment of TTD-MDP-based DMs, it has not been systematically studied to date. In this paper, we present the results of experiments with distance measures in this context, as well as lessons learned. This paper's main contribution is presenting empirically-founded practical advice for those wishing to actually deploy drama management based on TTD-MDPs on how best to construct a similarity measure over story trajectories. We also validate the effectiveness of the local probabilistic policy optimization technique used to solve TTD-MDPs in a regular but extremely large synthetic domain.
更多查看译文
关键词
ttd-mdp-based drama management,story similarity measure,interactive drama,deploy drama management,ttd-mdp-based dms,distance measure,empirically-founded practical advice,story trajectory,story outcome,effective technique,drama management
AI 理解论文
溯源树
样例
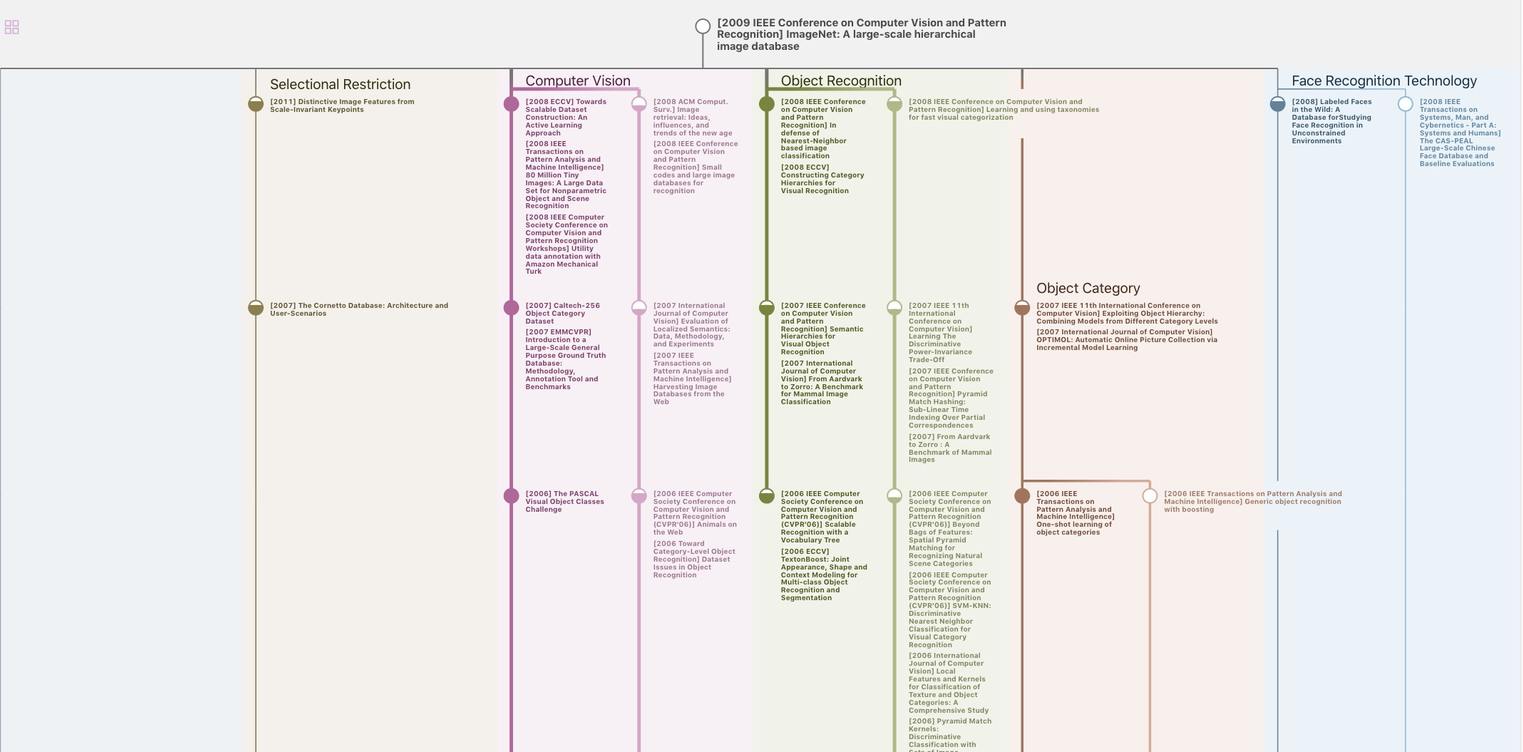
生成溯源树,研究论文发展脉络
Chat Paper
正在生成论文摘要