Choosing the Right Patterns An Experimental Comparison between Different Tree Inclusion Relations
Advances in Health Sciences Education(2008)
摘要
In recent years a variety of mining algorithms has been developed, to derive all frequent subtrees from a database of labeled ordered rooted trees. These algorithms share properties such as enumeration strategies and pruning techniques. They differ however in the tree inclusion relation used and how at- tribute values are dealt with. In this work we investigate the different approaches with respect to 'usefulness' of the derived patterns, in particular, the performance of classifiers that use the derived patterns as features. In order to find a good trade- off between expressiveness and runtime performance of the different approaches, we also take the complexity of the different classifiers into account, as well as the run time and memory usage of the different approaches. The experiments are performed on two real datasets. The results show that significant improvement in both predictive performance and computational efficiency can be gained by choosing the right tree mining approach.
更多查看译文
AI 理解论文
溯源树
样例
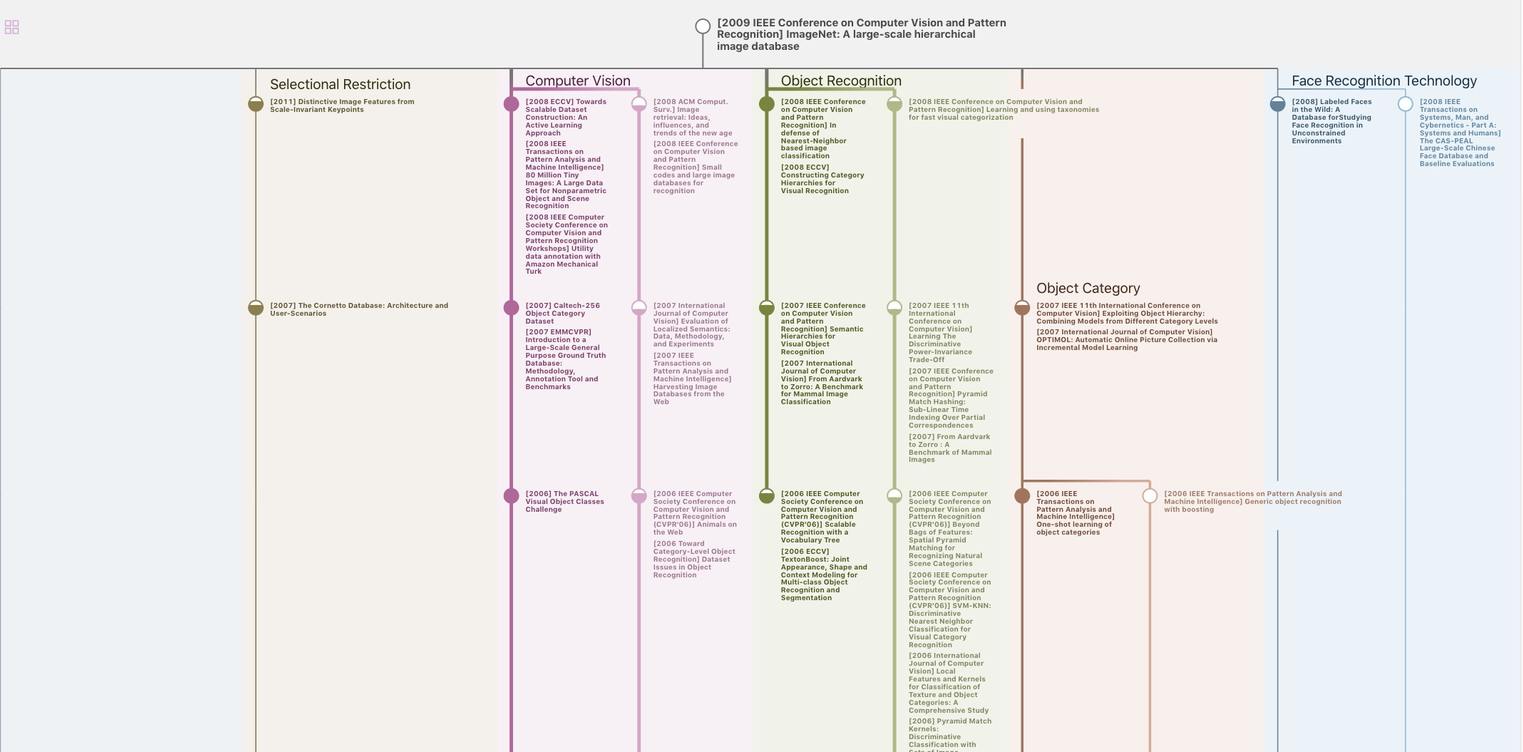
生成溯源树,研究论文发展脉络
Chat Paper
正在生成论文摘要