Graph clustering based on structural/attribute similarities
PVLDB(2009)
摘要
The goal of graph clustering is to partition vertices in a large graph into different clusters based on various criteria such as vertex connectivity or neighborhood similarity. Graph clustering techniques are very useful for detecting densely connected groups in a large graph. Many existing graph clustering methods mainly focus on the topological structure for clustering, but largely ignore the vertex properties which are often heterogenous. In this paper, we propose a novel graph clustering algorithm, SA-Cluster, based on both structural and attribute similarities through a unified distance measure. Our method partitions a large graph associated with attributes into k clusters so that each cluster contains a densely connected subgraph with homogeneous attribute values. An effective method is proposed to automatically learn the degree of contributions of structural similarity and attribute similarity. Theoretical analysis is provided to show that SA-Cluster is converging. Extensive experimental results demonstrate the effectiveness of SA-Cluster through comparison with the state-of-the-art graph clustering and summarization methods.
更多查看译文
关键词
neighborhood similarity,densely connected subgraph,structural similarity,attribute similarity,graph clustering,state-of-the-art graph clustering,existing graph,densely connected group,novel graph,large graph
AI 理解论文
溯源树
样例
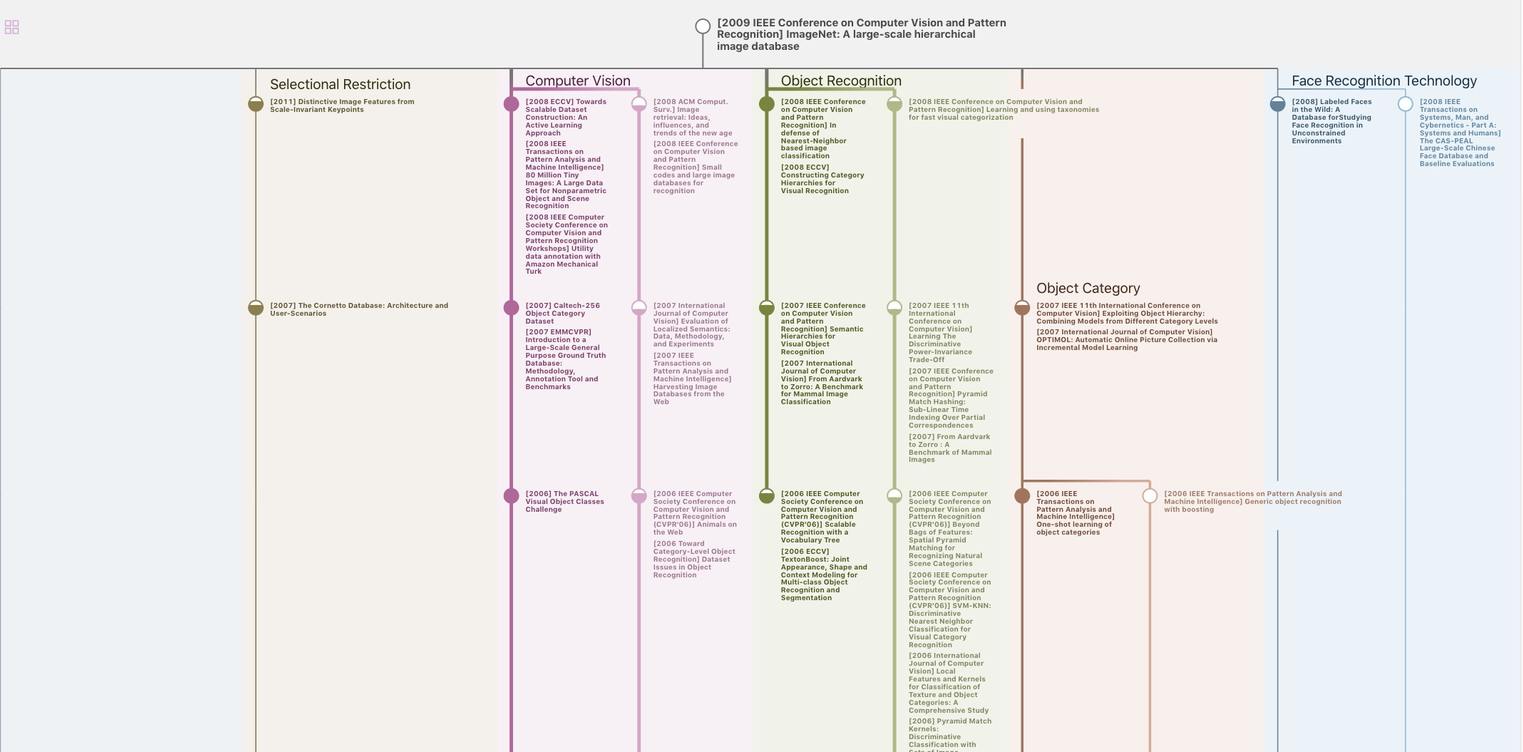
生成溯源树,研究论文发展脉络
Chat Paper
正在生成论文摘要