A comparison of alternative classifiers for detecting occurrence and intensity in spontaneous facial expression of infants with their mothers
Automatic Face and Gesture Recognition(2013)
摘要
To model the dynamics of social interaction, it is necessary both to detect specific Action Units (AUs) and variation in their intensity and coordination over time. An automated method that performs well when detecting occurrence may or may not perform well for intensity measurements. We compared two dimensionality reduction approaches - Principal Components Analysis with Large Margin Nearest Neighbor (PCA+LMNN) and Laplacian Eigenmap - and two classifiers, SVM and K-Nearest Neighbor. Twelve infants were video-recorded during face-to-face interactions with their mothers. AUs related to positive and negative affect were manually coded from the video by certified FACS coders. Facial features were tracked using Active Appearance Models (AAM) and registered to a canonical view before extracting Histogram of Oriented Gradients (HOG) features. All possible combinations of dimensionality reduction approaches and classifiers were tested using a leave-onesubject-out cross-validation. For detecting consistency (i.e. reliability as measured by ICC), PCA+LMNN and SVM classifiers gave best results.
更多查看译文
关键词
eigenvalues and eigenfunctions,face recognition,gradient methods,object detection,pattern classification,principal component analysis,support vector machines,AAM,FACS coder,HOG feature,LMNN,Laplacian eigenmap,PCA,SVM,action unit,active appearance model,dimensionality reduction,face-to-face interaction,facial feature,histogram of oriented gradient feature,infant,k-nearest neighbor,large margin nearest neighbor classifier,leave-one-subject-out cross-validation,mother,principal components analysis,social interaction dynamics,spontaneous facial expression,Action Unit,Facial Expressions,Histogram of Oriented Gradients,Laplacian Eigenmap,Structural SVM
AI 理解论文
溯源树
样例
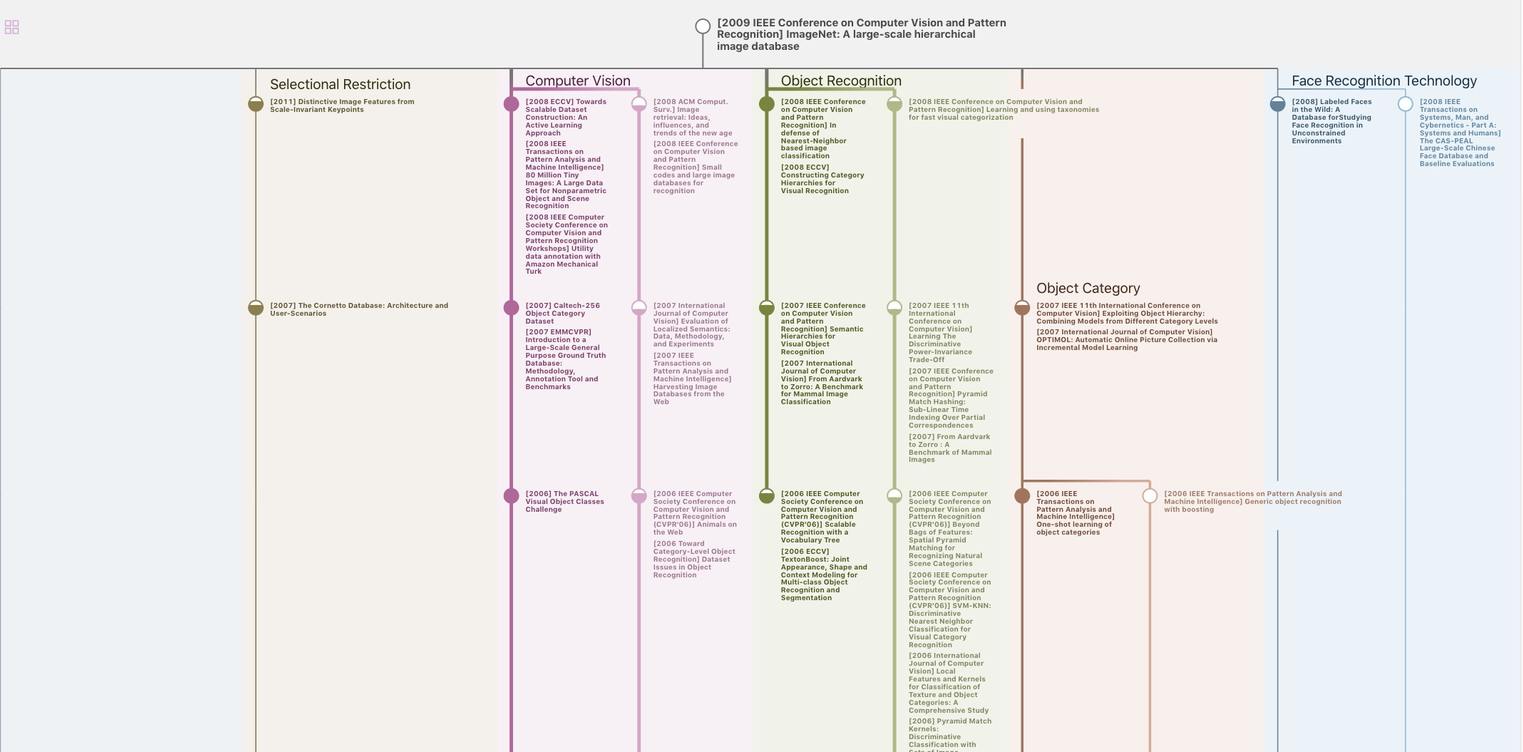
生成溯源树,研究论文发展脉络
Chat Paper
正在生成论文摘要