Constraining the Phrase-Based, Joint Probability Statistical Translation Model.
StatMT '06: Proceedings of the Workshop on Statistical Machine Translation(2006)
摘要
The joint probability model proposed by Marcu and Wong (2002) provides a strong probabilistic framework for phrase-based statistical machine translation (SMT). The model's usefulness is, however, limited by the computational complexity of estimating parameters at the phrase level. We present the first model to use word alignments for constraining the space of phrasal alignments searched during Expectation Maximization (EM) training. Constraining the joint model improves performance, showing results that are very close to state-of-the-art phrase-based models. It also allows it to scale up to larger corpora and therefore be more widely applicable.
更多查看译文
关键词
joint model,joint probability model,state-of-the-art phrase-based model,phrase-based statistical machine translation,Expectation Maximization,computational complexity,larger corpus,phrasal alignment,phrase level,strong probabilistic framework,statistical translation model
AI 理解论文
溯源树
样例
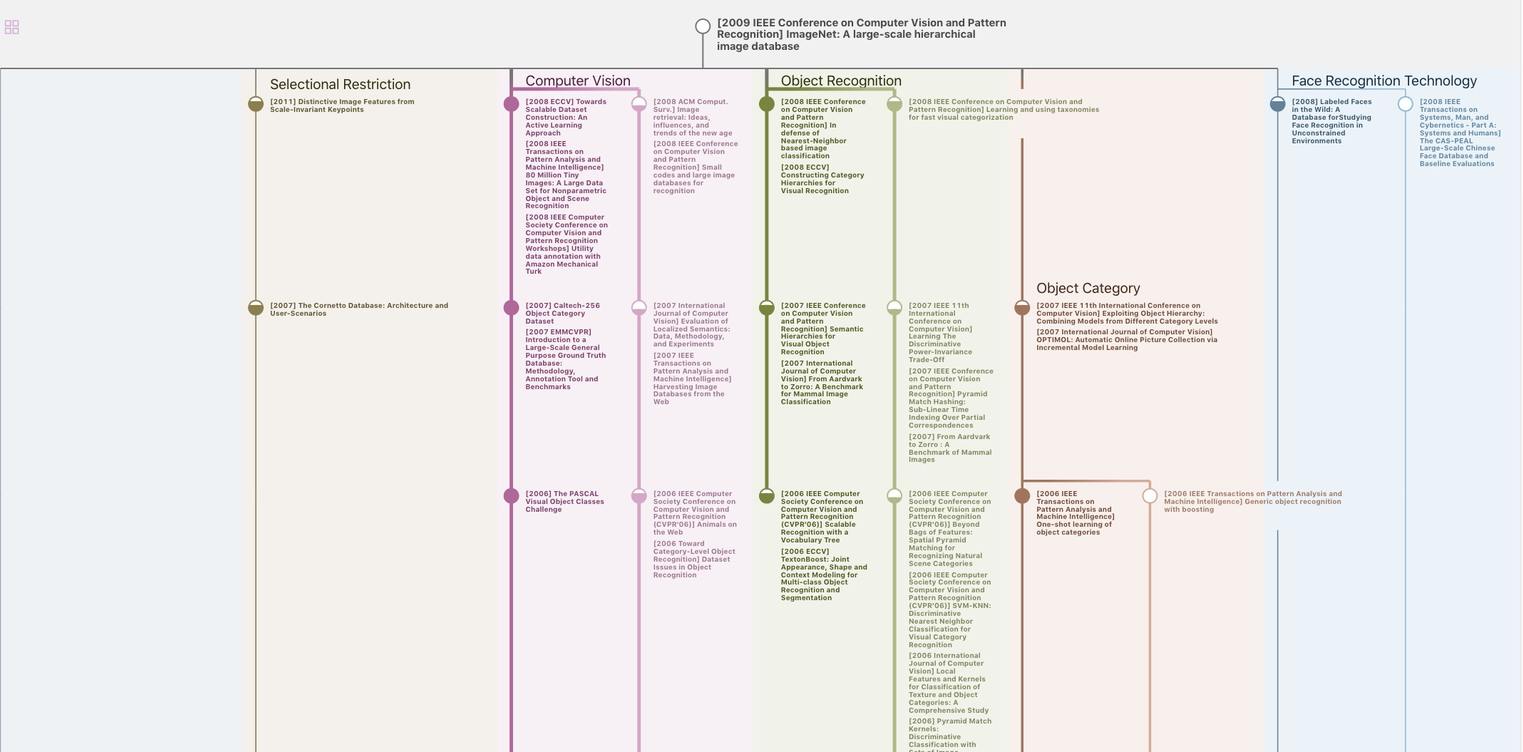
生成溯源树,研究论文发展脉络
Chat Paper
正在生成论文摘要