Cross-Guided Clustering: Transfer of Relevant Supervision across Tasks
TKDD(2012)
摘要
Lack of supervision in clustering algorithms often leads to clusters that are not useful or interesting to human reviewers. We investigate if supervision can be automatically transferred for clustering a target task, by providing a relevant supervised partitioning of a dataset from a different source task. The target clustering is made more meaningful for the human user by trading-off intrinsic clustering goodness on the target task for alignment with relevant supervised partitions in the source task, wherever possible. We propose a cross-guided clustering algorithm that builds on traditional k-means by aligning the target clusters with source partitions. The alignment process makes use of a cross-task similarity measure that discovers hidden relationships across tasks. When the source and target tasks correspond to different domains with potentially different vocabularies, we propose a projection approach using pivot vocabularies for the cross-domain similarity measure. Using multiple real-world and synthetic datasets, we show that our approach improves clustering accuracy significantly over traditional k-means and state-of-the-art semi-supervised clustering baselines, over a wide range of data characteristics and parameter settings.
更多查看译文
关键词
source partition,target cluster,different source task,cross-guided clustering algorithm,state-of-the-art semi-supervised clustering baselines,target task,relevant supervision,target clustering,clustering algorithm,cross-guided clustering,trading-off intrinsic clustering goodness,traditional k-means,transfer,multitask
AI 理解论文
溯源树
样例
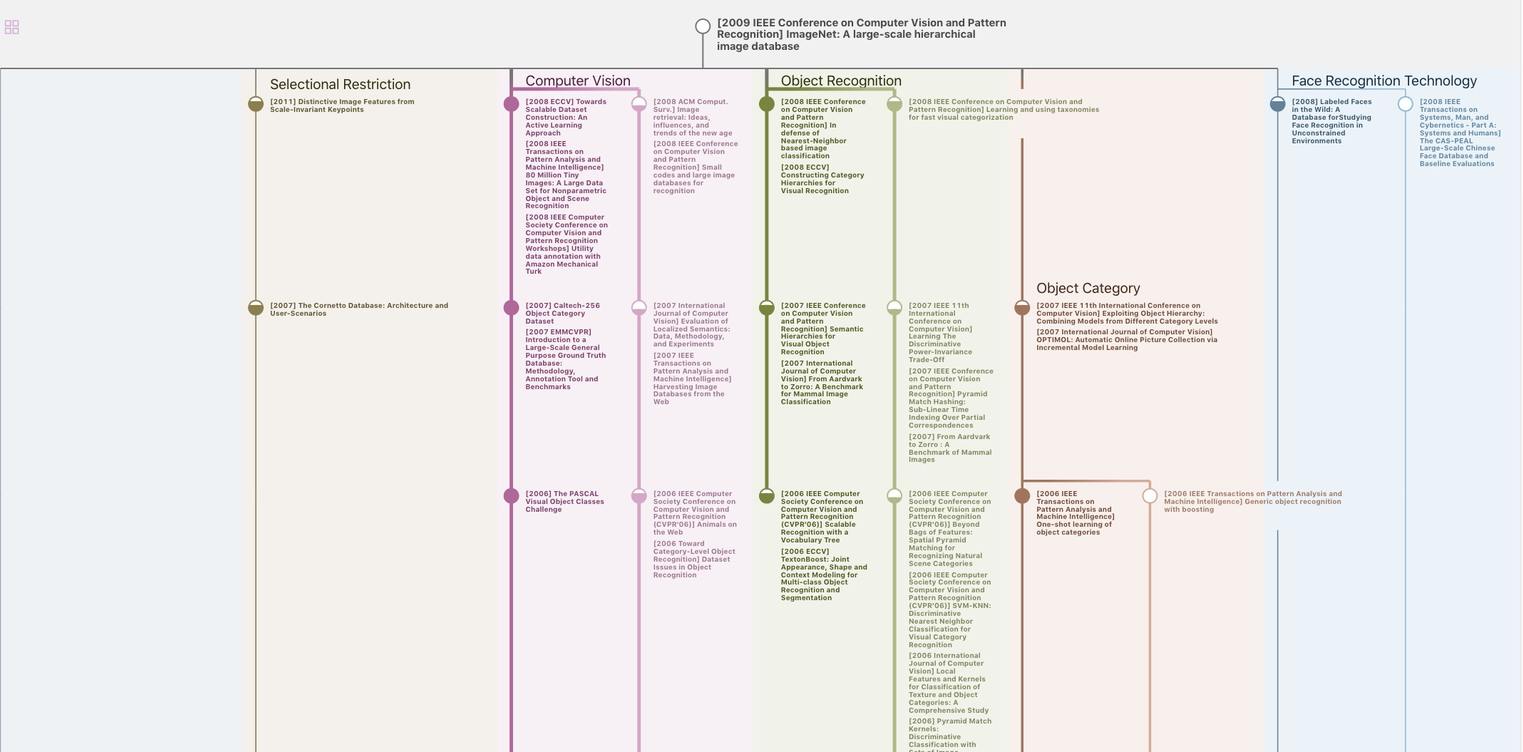
生成溯源树,研究论文发展脉络
Chat Paper
正在生成论文摘要