Robust regularized feature selection for iris recognition via linear programming
ICPR(2012)
摘要
Ordinal measures are robust image descriptors for encoding discriminative features of iris images. However, there are many tunable parameters in ordinal filters which can generate an over-complete feature pool. This paper proposes a novel feature selection method based on linear programming, which can learn a compact and effective ordinal feature set for iris recognition. Firstly, large margin principle is employed to obtain strong generalization capacity. Secondly, discriminative information for each feature is added to make the model more robust to noise. Finally, non-negative weight is not only interpretable but also suitable for a linear model. Additionally, the model can be efficiently solved by the Simplex algorithm. The comparative experiments are conducted on CASIA-Iris-V4 database, and the results show that our method has outperformed other state-of-the-art feature selection methods, including Adaboost and Sparsity based methods.
更多查看译文
关键词
comparative experiments,discriminative information,learning (artificial intelligence),visual databases,vocabulary,state-of-the-art feature selection methods,over-complete feature pool,iris images,linear programming,simplex algorithm,adaboost based methods,ordinal filters,iris recognition,feature extraction,sparsity based methods,casia-iris-v4 database,nonnegative weight,tunable parameters,generalisation (artificial intelligence),discriminative feature encoding,robust image descriptors,generalization capacity,ordinal feature set,robust regularized feature selection methods,margin principle,learning artificial intelligence
AI 理解论文
溯源树
样例
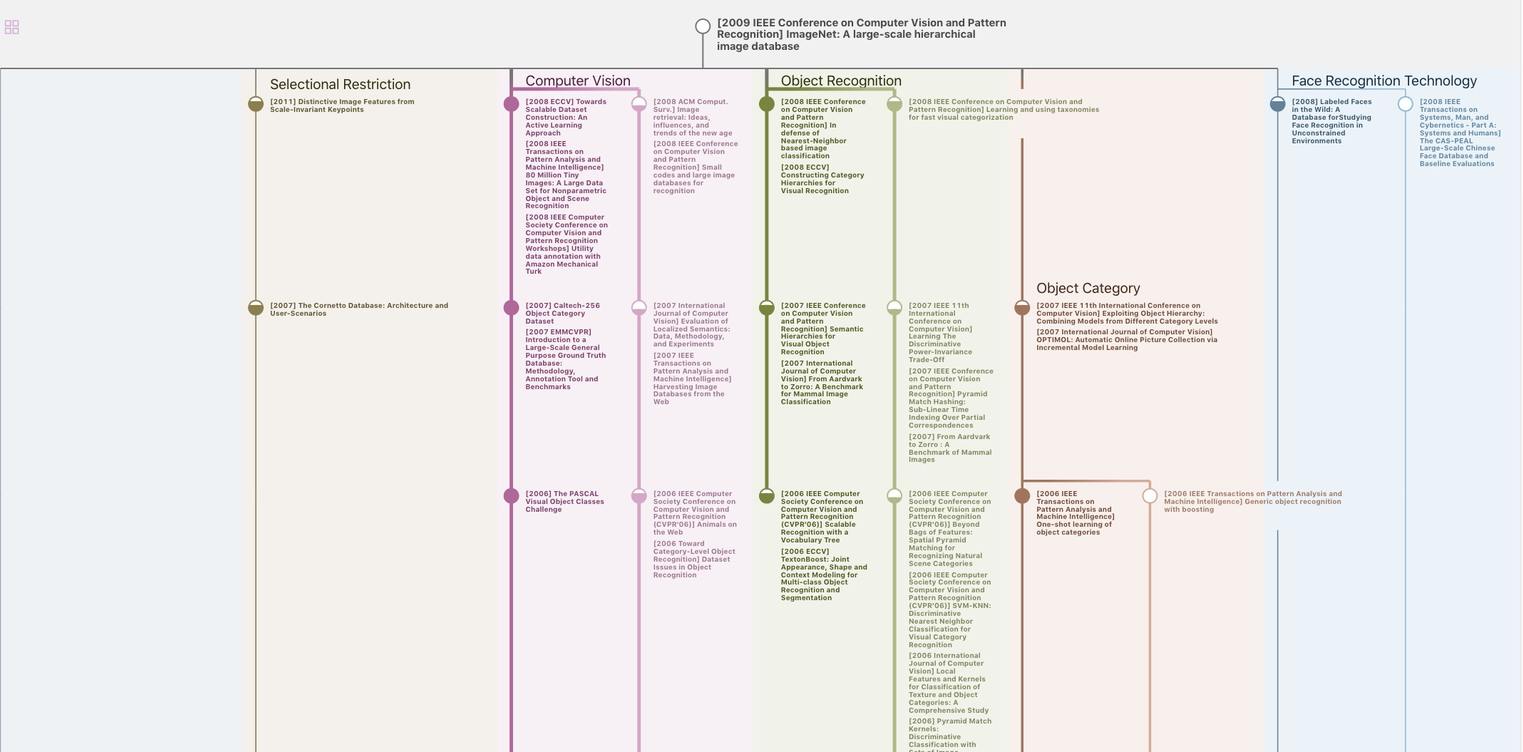
生成溯源树,研究论文发展脉络
Chat Paper
正在生成论文摘要