A Self-Learning Approach to Single Image Super-Resolution
IEEE Transactions on Multimedia(2013)
摘要
Learning-based approaches for image super-resolution (SR) have attracted the attention from researchers in the past few years. In this paper, we present a novel self-learning approach for SR. In our proposed framework, we advance support vector regression (SVR) with image sparse representation, which offers excellent generalization in modeling the relationship between images and their associated SR versions. Unlike most prior SR methods, our proposed framework does not require the collection of training low and high-resolution image data in advance, and we do not assume the reoccurrence (or self-similarity) of image patches within an image or across image scales. With theoretical supports of Bayes decision theory, we verify that our SR framework learns and selects the optimal SVR model when producing an SR image, which results in the minimum SR reconstruction error. We evaluate our method on a variety of images, and obtain very promising SR results. In most cases, our method quantitatively and qualitatively outperforms bicubic interpolation and state-of-the-art learning-based SR approaches.
更多查看译文
关键词
image representation,svr,interpolation,bicubic interpolation,learning (artificial intelligence),self-learning approach,support vector regression,image resolution,regression analysis,single image super-resolution,bayes methods,super-resolution,bayes decision theory,image sparse representation,self-learning,support vector machines,sparse representation,materials,image reconstruction,learning artificial intelligence,training data,super resolution,decision theory
AI 理解论文
溯源树
样例
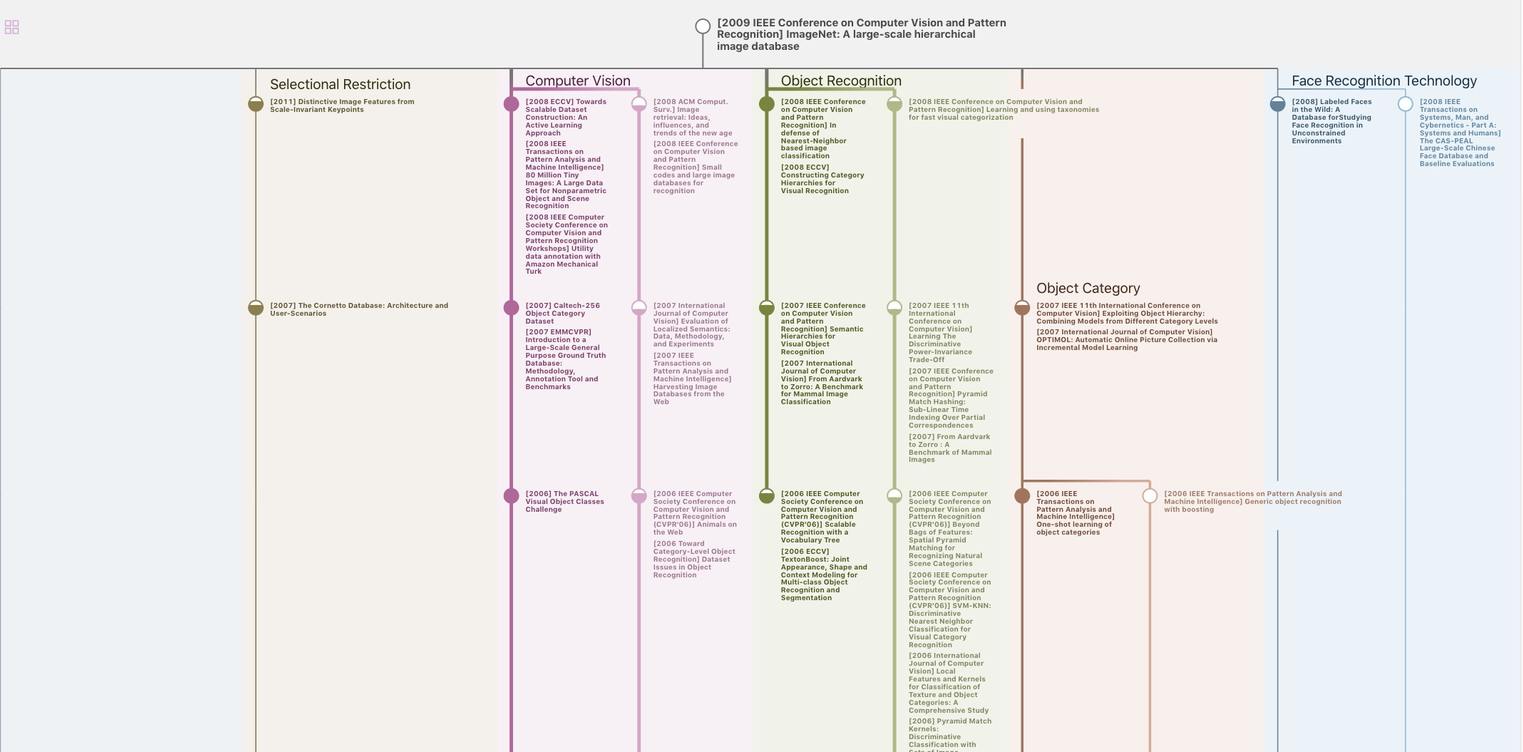
生成溯源树,研究论文发展脉络
Chat Paper
正在生成论文摘要