Noisy Filtered Sparse Processes: Reconstruction And Compression
49TH IEEE CONFERENCE ON DECISION AND CONTROL (CDC)(2010)
摘要
In this paper we consider estimation and compression of filtered sparse processes. Specifically, the filtered sparse process is a signal x is an element of R-n obtained by driving a k-sparse signal u is an element of R-n through an arbitrary unknown stable discrete-linear time invariant system H of a known order. The signal x (t) is measured noisily. We consider estimation of x (t) from noisy measurements. We also consider compression of x (t) by means of random projections analogous to compressed sensing. For different cases including AR and MA systems we show that x can indeed be reconstructed from O(k log (n)) measurements. We develop a novel LP optimization algorithm and show that both the unknown filter H and the sparse input u can be reliably estimated.
更多查看译文
关键词
compressed sensing,mathematical model,estimation,correlation,convolution,linear systems,noise measurement,linear time invariant
AI 理解论文
溯源树
样例
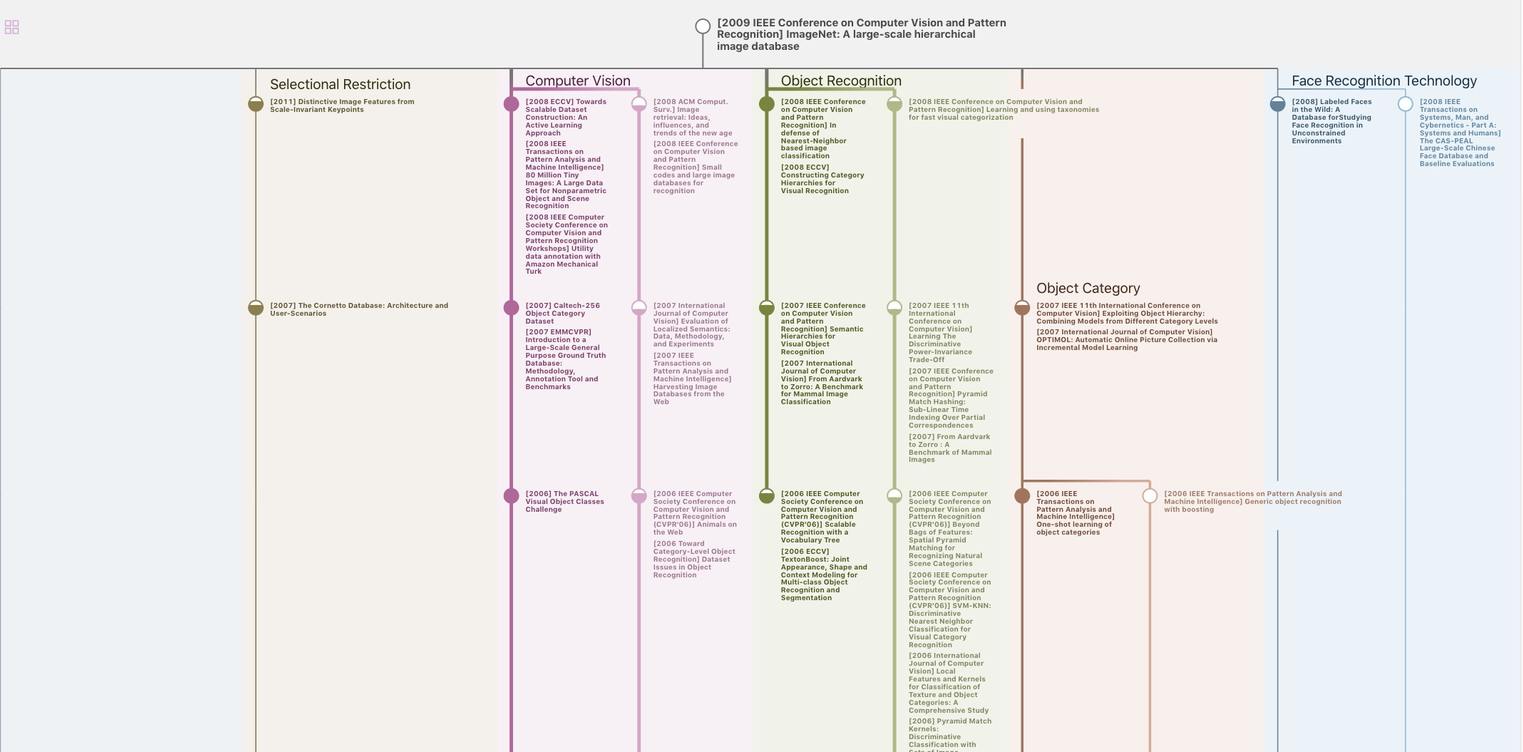
生成溯源树,研究论文发展脉络
Chat Paper
正在生成论文摘要