Hidden Markov models for tracking neuronal structure contours in electron micrograph stacks
ISBI(2012)
摘要
This paper is focused on the problem of tracking cell contours across an electron micrograph stack, so as to discern the 3D neuronal structures, with particular application to analysis of retinal images. While the problem bears similarity to traditional object tracking in video sequences, it poses additional significant challenges due to the coarse z-axis resolution which causes large contour deformations across frames, and involves major topological changes including contour splits and merges. The method proposed herein applies a deformable trellis, on which a hidden Markov model is defined, to track contour deformation. The first phase produces an estimated new contour and computes its probability given the model. The second phase detects low-confidence contour segments and tests the hypothesis that a topological change has occurred, by introducing corresponding hypothetical arcs and re-optimizing the contour. The most probable solution, including the topological hypothesis, is identified. Experimental results show, both quantitatively and qualitatively, that the proposed approach can effectively and efficiently track cell contours while accounting for splitting, merging, large contour displacements and deformations.
更多查看译文
关键词
eye,topological change,cellular biophysics,neuronal structure tracking,neurophysiology,topological changes,retinal image,image segmentation,contour segments,topological hypothesis,deformable trellis,object tracking,electron micrograph,scanning electron microscopy,electron micrograph stack,3d neuronal structure contour tracking,cell contour,hidden markov models,medical image processing,hidden markov model,contour deformation tracking,video sequence,merging,computational modeling,level set,active contour,computer model
AI 理解论文
溯源树
样例
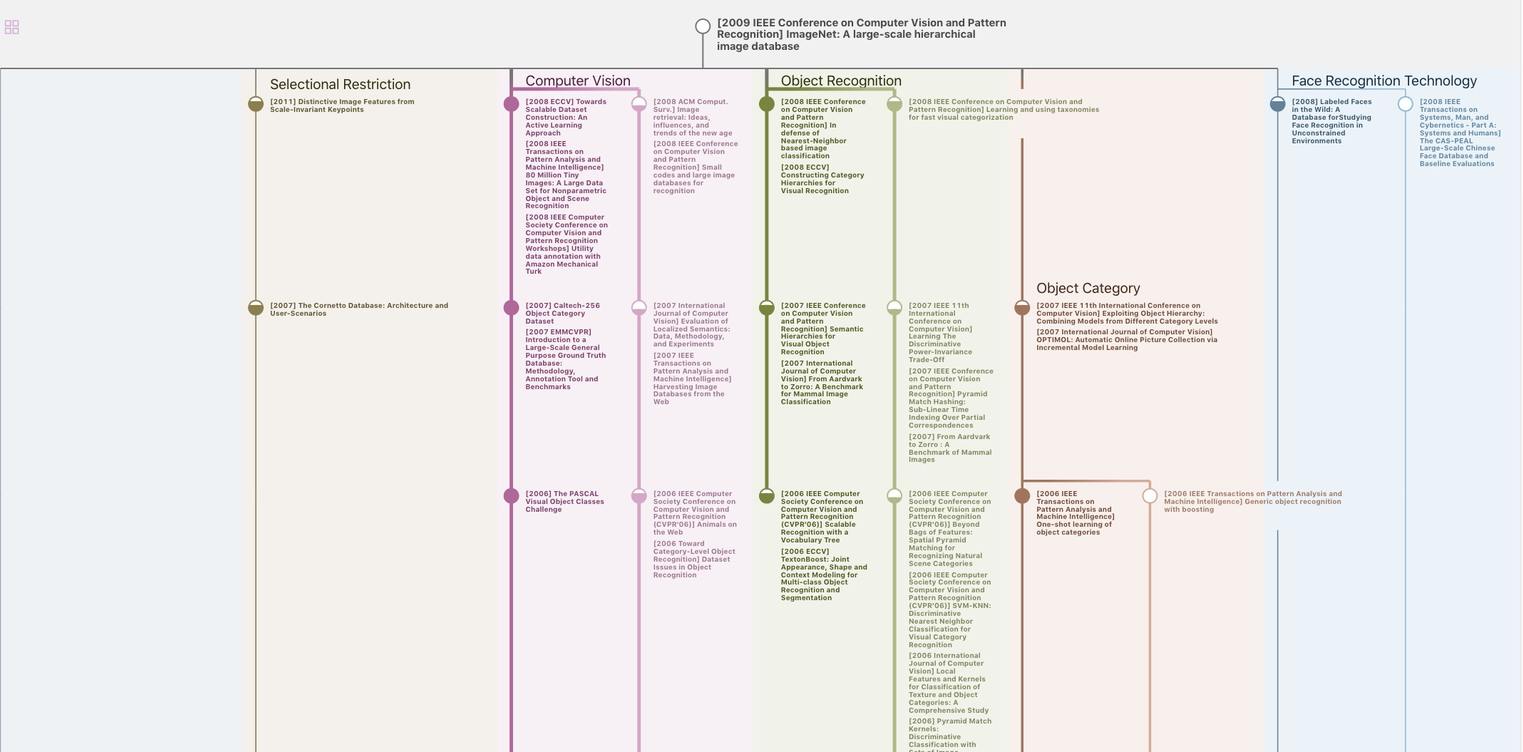
生成溯源树,研究论文发展脉络
Chat Paper
正在生成论文摘要