Yet Another Method for Combining Classifiers Outputs: A Maximum Entropy Approach
Lecture Notes in Computer Science(2004)
摘要
In this paper, we present a maximum entropy (maxent) approach to the fusion of experts opinions, or classifiers outputs, problem. The maxent approach is quite versatile and allows us to express in a clear, rigorous, way the a priori knowledge that is available on the problem. For instance, our knowledge about the reliability of the experts and the correlations between these experts can be easily integrated: Each piece of knowledge is expressed in the form of a linear constraint. An iterative scaling algorithm is used in order to compute the maxent solution of the problem. The maximum entropy method seeks the joint probability density of a set of random variables that has maximum entropy while satisfying the constraints. It is therefore the "most honest" characterization of our knowledge given the available facts (constraints). In the case of conflicting constraints, we propose to minimise the "lack of constraints satisfaction" or to relax some constraints and recompute the maximum entropy solution. The maxent fusion rule is illustrated by some simulations.
更多查看译文
关键词
random variable,constraint satisfaction,probability density,satisfiability,a priori knowledge,maximum entropy
AI 理解论文
溯源树
样例
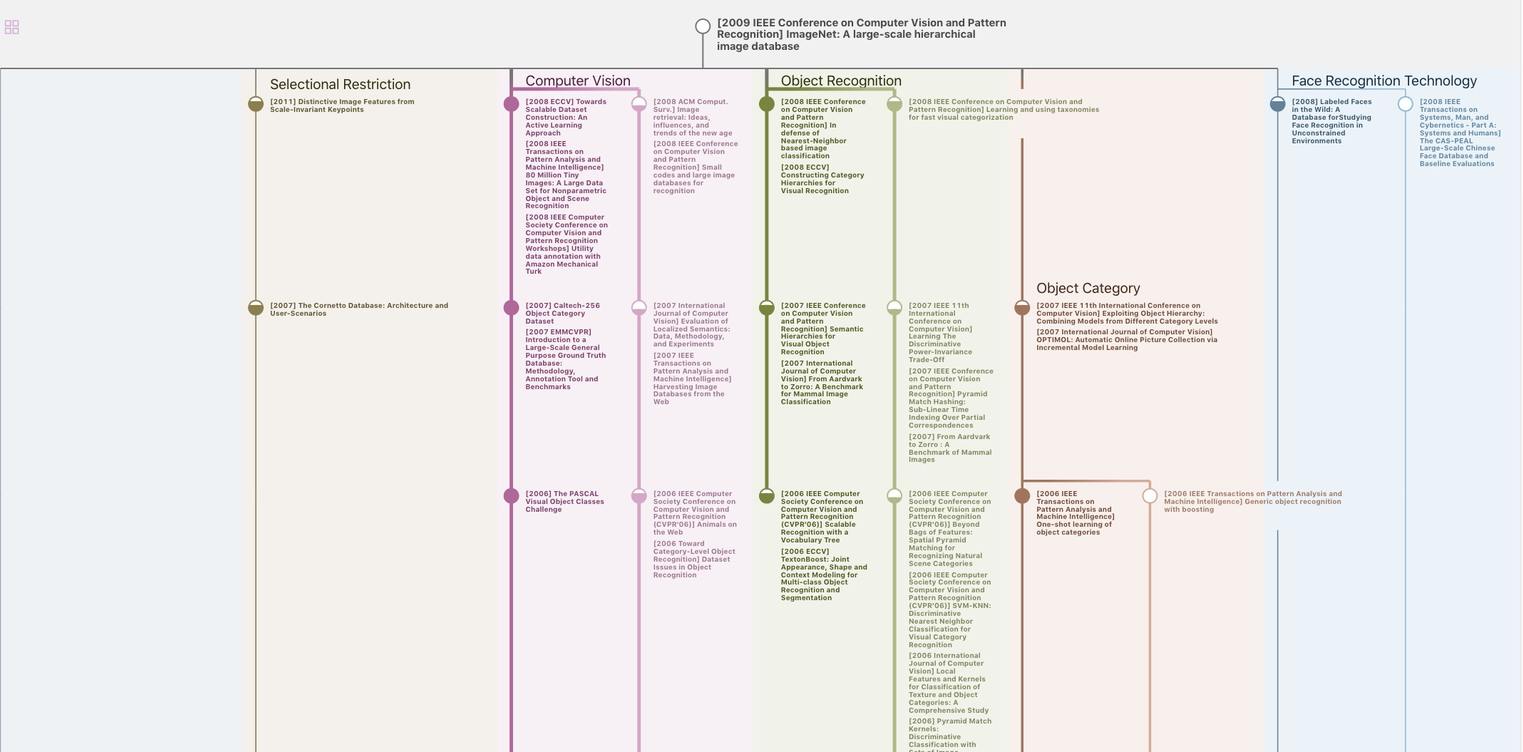
生成溯源树,研究论文发展脉络
Chat Paper
正在生成论文摘要